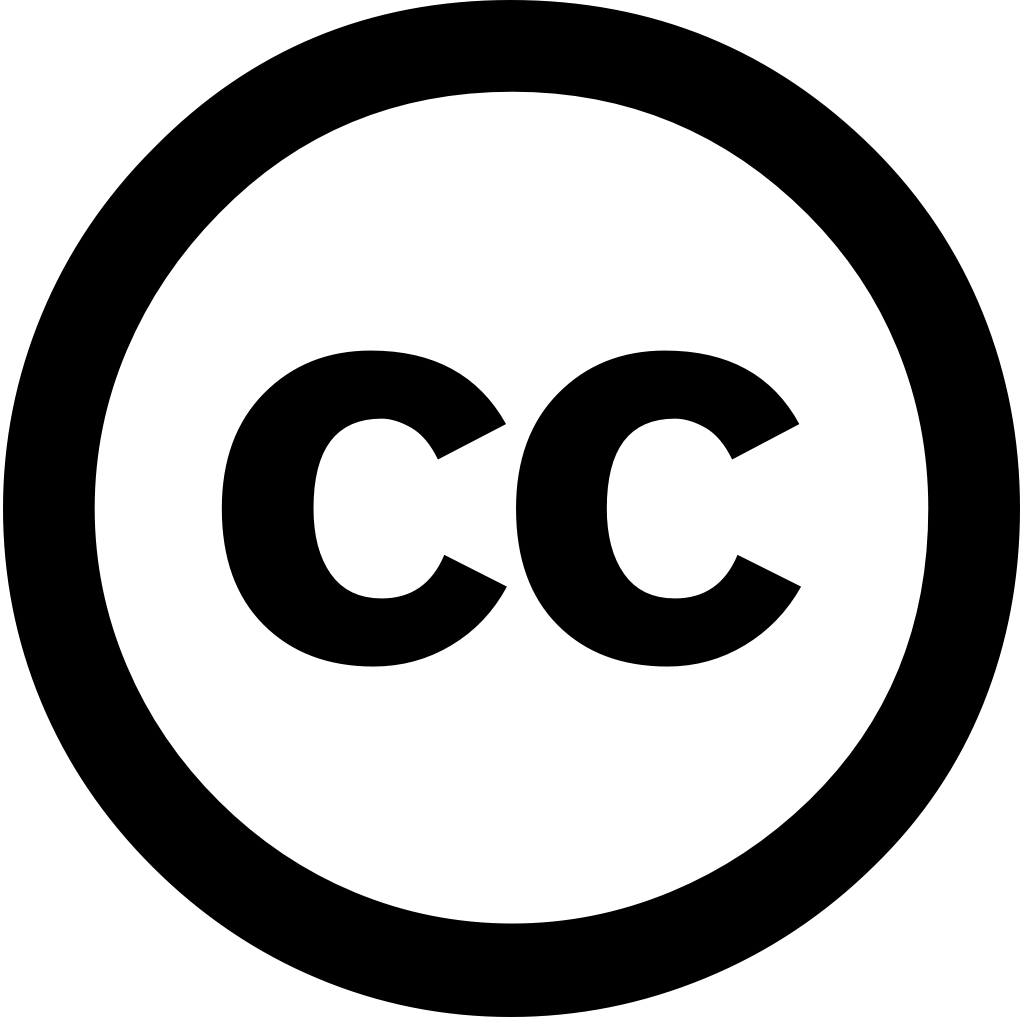
Biomedicines, Journal Year: 2025, Volume and Issue: 13(5), P. 1025 - 1025
Published: April 24, 2025
Background: Artificial intelligence tools can help improve the clinical management of patients with severe COVID-19. The aim this study was to validate a machine learning model predict admission Intensive Care Unit (ICU) in individuals Methods: A total 201 hospitalized COVID-19 were included. Sociodemographic and data as well laboratory biomarker results obtained from medical records information system. Three models generated, trained, internally validated: logistic regression (LR), random forest (RF), extreme gradient boosting (XGBoost). evaluated for sensitivity (Sn), specificity (Sp), area under curve (AUC), precision (P), SHapley Additive exPlanation (SHAP) values, utility predictive using decision analysis (DCA). Results: included following variables: type 2 diabetes mellitus (T2DM), obesity, absolute neutrophil basophil counts, neutrophil-to-lymphocyte ratio (NLR), D-dimer levels on day hospital admission. LR showed an Sn 0.67, Sp 0.65, AUC 0.74, P 0.66. RF achieved 0.87, 0.83, 0.96, 0.85. XGBoost demonstrated 0.85, 0.95, 0.86. Conclusions: Among models, robust performance (Sn = 0.86) favorable net benefit analysis, confirming its suitability predicting ICU aiding decision-making.
Language: Английский