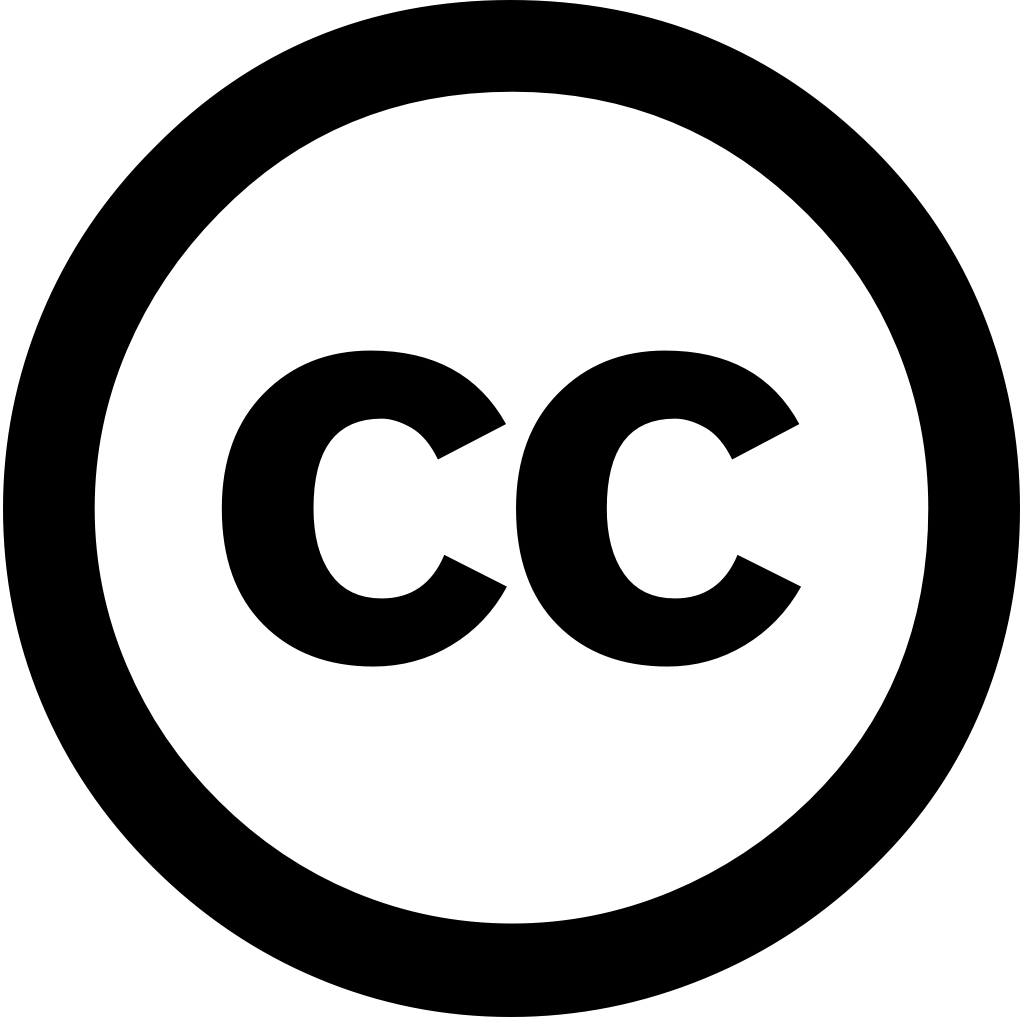
Journal of King Saud University - Computer and Information Sciences, Journal Year: 2024, Volume and Issue: 36(10), P. 102253 - 102253
Published: Dec. 1, 2024
Language: Английский
Journal of King Saud University - Computer and Information Sciences, Journal Year: 2024, Volume and Issue: 36(10), P. 102253 - 102253
Published: Dec. 1, 2024
Language: Английский
Machine Learning and Knowledge Extraction, Journal Year: 2024, Volume and Issue: 6(2), P. 827 - 841
Published: April 15, 2024
Dataset imbalances pose a significant challenge to predictive modeling in both medical and financial domains, where conventional strategies, including resampling algorithmic modifications, often fail adequately address minority class underrepresentation. This study theoretically practically investigates how the inherent nature of data affects classification classes. It employs ten machine deep learning classifiers, ranging from ensemble learners cost-sensitive algorithms, across comparably sized datasets. Despite these efforts, none classifiers achieved effective dataset, with sensitivity below 5.0% area under curve (AUC) 57.0%. In contrast, similar applied dataset demonstrated strong discriminative power, overall accuracy exceeding 95.0%, over 73.0%, AUC above 96.0%. disparity underscores unpredictable variability data, as exemplified by dispersed homogeneous distribution among other classes principal component analysis (PCA) graphs. The application synthetic oversampling technique (SMOTE) introduced 62 patients based on merely 20 original cases, casting doubt its clinical validity representation real-world patient variability. Furthermore, post-SMOTE feature importance analysis, utilizing SHapley Additive exPlanations (SHAP) tree-based methods, contradicted established cerebral stroke parameters, further questioning coherence augmentation. These findings call into question SMOTE underscore urgent need for advanced techniques innovations predicting minority-class outcomes datasets without depending strategies. approach developing methods that are not only robust but also clinically relevant applicable scenarios. Consequently, this future research efforts bridge gap between theoretical advancements practical, applications models like healthcare.
Language: Английский
Citations
9PLoS ONE, Journal Year: 2025, Volume and Issue: 20(1), P. e0312124 - e0312124
Published: Jan. 2, 2025
Predicting learning achievement is a crucial strategy to address high dropout rates. However, existing prediction models often exhibit biases, limiting their accuracy. Moreover, the lack of interpretability in current machine methods restricts practical application education. To overcome these challenges, this research combines strengths various algorithms design robust model that performs well across multiple metrics, and uses analysis elucidate results. This study introduces predictive framework for based on ensemble techniques. Specifically, six distinct are utilized establish base learner, with logistic regression serving as meta learner construct an predicting achievement. The SHapley Additive exPlanation (SHAP) then employed explain Through experiments XuetangX dataset, effectiveness proposed verified. outperforms traditional deep terms results demonstrate learning-based significantly methods. feature importance analysis, SHAP method enhances improves reliability results, enabling more personalized interventions support students.
Language: Английский
Citations
0Applied Intelligence, Journal Year: 2025, Volume and Issue: 55(5)
Published: Jan. 22, 2025
Language: Английский
Citations
0Engineering Applications of Artificial Intelligence, Journal Year: 2025, Volume and Issue: 144, P. 110102 - 110102
Published: Jan. 25, 2025
Language: Английский
Citations
0Soft Computing, Journal Year: 2025, Volume and Issue: 29(4), P. 2031 - 2045
Published: Feb. 1, 2025
Language: Английский
Citations
0Applied Sciences, Journal Year: 2025, Volume and Issue: 15(9), P. 4670 - 4670
Published: April 23, 2025
In view of the data fault diagnosis and good product testing in industrial field, high-noise unbalanced samples exist widely, such are very difficult to analyze field analysis. The oversampling technique has proved be a simple solution past, but it no significant resistance noise. order solve binary classification problem data, an enhanced majority-weighted minority technique, MWMOTE-FRIS-INFFC, is introduced this study, which specially used for processing noise-unbalanced classified sets. method uses Euclidean distance assign sample weights, synthesizes combines new into with larger weights belonging few classes, thus solves scarcity smaller class clusters. Then, fuzzy rough instance selection (FRIS) eliminate subsets synthetic low clustering membership, effectively reduces overfitting tendency caused by oversampling. addition, integration fusion iterative filters (INFFC) helps mitigate noise issues, both raw On basis, series experiments designed improve performance 6 algorithms on 8 sets using MWMOTE-FRIS-INFFC algorithm proposed paper.
Language: Английский
Citations
0Published: Jan. 1, 2024
Class imbalance and heterogeneous data distribution pose significant challenges in classification tasks across various real-world applications. Addressing these issues, this paper introduces the Geometric Relative Margin Machine (GRMM), a novel model that innovatively merges strategies of with advanced adjustment techniques. GRMM is specifically designed to effectively manage dual class heterogeneity. Empirical evaluations on benchmark datasets practical scenarios reveal not only significantly improves accuracy but also enhances robustness against diverse distributions. This study underscores efficacy navigating complexities varied sizes distributions, showcasing its potential as superior tool for complex problems.
Language: Английский
Citations
0Published: May 2, 2024
Language: Английский
Citations
0Information Processing & Management, Journal Year: 2024, Volume and Issue: 62(2), P. 103975 - 103975
Published: Nov. 23, 2024
Language: Английский
Citations
0Journal of King Saud University - Computer and Information Sciences, Journal Year: 2024, Volume and Issue: 36(10), P. 102253 - 102253
Published: Dec. 1, 2024
Language: Английский
Citations
0