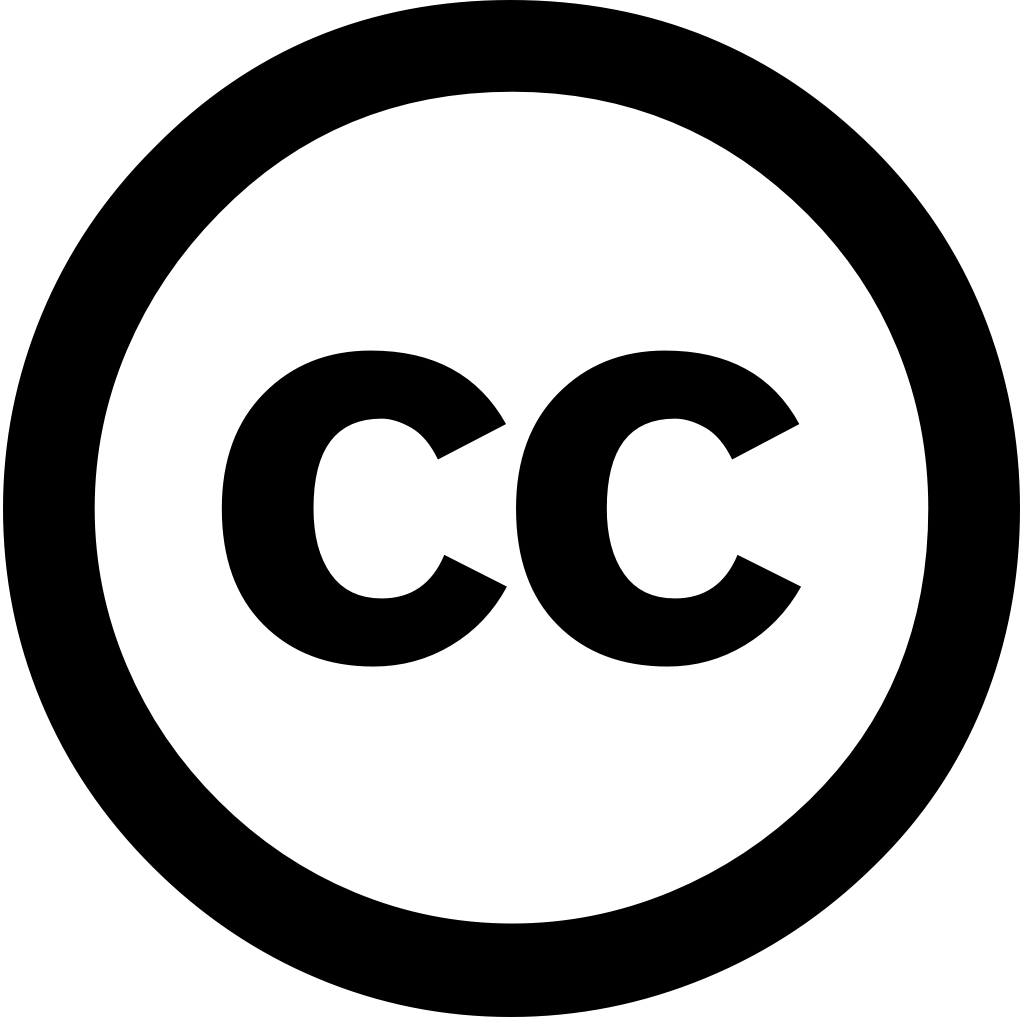
Remote Sensing, Journal Year: 2024, Volume and Issue: 17(1), P. 54 - 54
Published: Dec. 27, 2024
The timely and accurate monitoring of wildfires other sudden natural disasters is crucial for safeguarding the safety residents their property. Satellite imagery wildfire offers a unique opportunity to obtain near-real-time disaster information through rapid, large-scale remote sensing mapping. However, existing methods are constrained by temporal spatial limitations imagery, preventing comprehensive fulfillment need high resolution in early warning. To address this gap, we propose high-precision extraction method without training—SAFE. SAFE combines generalization capabilities Segmentation Anything Model (SAM) effectiveness hotspot product data such as MODIS VIIRS. employs two-step localization strategy incrementally identify burned areas pixels post-wildfire thereby reducing computational load providing high-resolution impact areas. area generated can subsequently be used train lightweight regional models, establishing detection models applicable various regions, ultimately undetected We validated four test regions representing two typical scenarios—grassland forest. results showed that SAFE’s F1-score was, on average, 9.37% higher than alternative methods. Additionally, application scenarios demonstrated its potential capability detect fine distribution impacts global scale.
Language: Английский