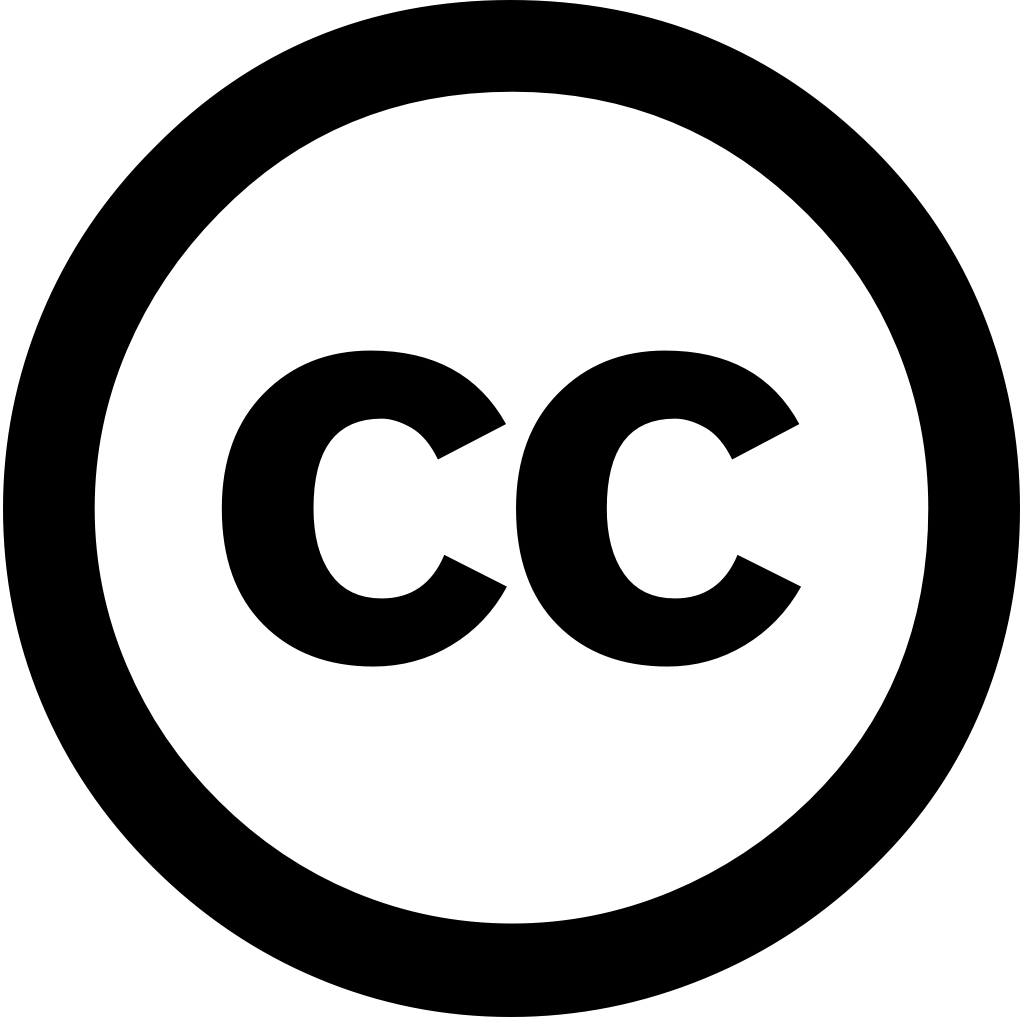
Energy and AI, Journal Year: 2024, Volume and Issue: 18, P. 100418 - 100418
Published: Aug. 23, 2024
Language: Английский
Energy and AI, Journal Year: 2024, Volume and Issue: 18, P. 100418 - 100418
Published: Aug. 23, 2024
Language: Английский
Transportation Infrastructure Geotechnology, Journal Year: 2024, Volume and Issue: 12(1)
Published: Nov. 13, 2024
Language: Английский
Citations
4International Journal of Green Energy, Journal Year: 2025, Volume and Issue: unknown, P. 1 - 17
Published: Jan. 7, 2025
This research proposes a new method for wind turbine fault detection using hybrid deep neural networks, along with explainable Artificial Intelligence (XAI) methods. unique combination delivers more accurate and interpretable model to enable improved maintenance strategies efficiency in operations. The novelty of this work is that it an intact methodology utilizing AI turbines overcome the known weaknesses existing methods terms transparency interpretability. aims create elaborate system capable accurately predicting faults providing engineers transparent comprehendible explanations regarding decisions undertaken, which will further contribute learning. observations were made during numerous simulations tests, proposed XAI-driven indicated significant increase 99% accuracy rate maintained level effectiveness upkeep. approach expected reduce frequency operation disruptions put as standard reliability industry, nests potential novel industrial asset would significantly redefine rules field.
Language: Английский
Citations
0STUDIES IN ENGINEERING AND EXACT SCIENCES, Journal Year: 2025, Volume and Issue: 6(1), P. e13159 - e13159
Published: Jan. 8, 2025
Wind Energy Systems is an area of research which maturing rapidly due to the concern long term global energy consumption, currently systems and dynamics ongoing in modeling identification primarily for controller design, state estimation, fault detection diagnosis. This paper presents a method estimate identify wind turbine mathematical model, includes structural deformation (the growth size, loads turbine…) aerodynamic effects. Finding model that would be good representation system at same time suitable design very difficult task strongly nonlinear. To get since we used algorithm, namely autoregressive eigensystem realization algorithm. improve prediction, optimal filtering by using Kalman filter.
Language: Английский
Citations
0Eng—Advances in Engineering, Journal Year: 2025, Volume and Issue: 6(1), P. 20 - 20
Published: Jan. 20, 2025
Despite their significant environmental benefits, solar photovoltaic (PV) systems are susceptible to malfunctions and performance degradation. This paper addresses detecting diagnosing faults from a dataset representing 250 kW PV power plant with three types of faults. A comprehensive analysis is conducted improve the quality uncover intricate relationships between features target variable. By introducing novel feature importance averaging techniques, two-phase fault detection diagnosis framework employing tree-based models proposed identify normal cases diagnose type. An ensemble six classifiers, including decision trees, random forest, Stochastic Gradient Boosting, LightGBM, CatBoost, Extra Trees, trained in both phases. The results show 100% accuracy first phase, particularly Trees classifier. In second XGBoost, CatBoost achieve similar accuracy, demonstrating superior training convergence speed. study then incorporates Explainable Artificial Intelligence (XAI), utilizing LIME SHAP analyzers validate research findings. highlight superiority approach over others, solidifying its position as an innovative effective solution for systems.
Language: Английский
Citations
0Computer-Aided Civil and Infrastructure Engineering, Journal Year: 2025, Volume and Issue: unknown
Published: March 22, 2025
Abstract High‐frequency detection of track defects is crucial for accurate condition assessment and system safety. Onboard vibration data collection devices can significantly increase density without additional costs. However, defect based on this challenging, including the spatial heterogeneity parameters, distribution mismatch between labels, variability in responses across different defects. This study proposes a multilevel framework vehicle body vibration. The correlation intensity vibrations factors was analyzed, correlation‐view spectral clustering algorithm designed to achieve effective set partitioning. A spectral‐normalized neural Gaussian process‐based adaptive‐threshold self‐training method (SNGP‐ASM) developed generate high‐quality pseudo‐labels fully labeled set. An attention‐guided multitask cascaded convolutional network (CNN) constructed progressively assess using channel‐wise attentions cross‐hierarchical attention guidance module. Validations multiple Chinese metro lines demonstrated that achieved high performance training testing most tasks within lines, trained model effectively adapt new with only lightweight fine‐tuning. Moreover, maintained computational efficiency, enabling high‐frequency monitoring practical deployment scenarios.
Language: Английский
Citations
0Energy and AI, Journal Year: 2024, Volume and Issue: 18, P. 100418 - 100418
Published: Aug. 23, 2024
Language: Английский
Citations
3