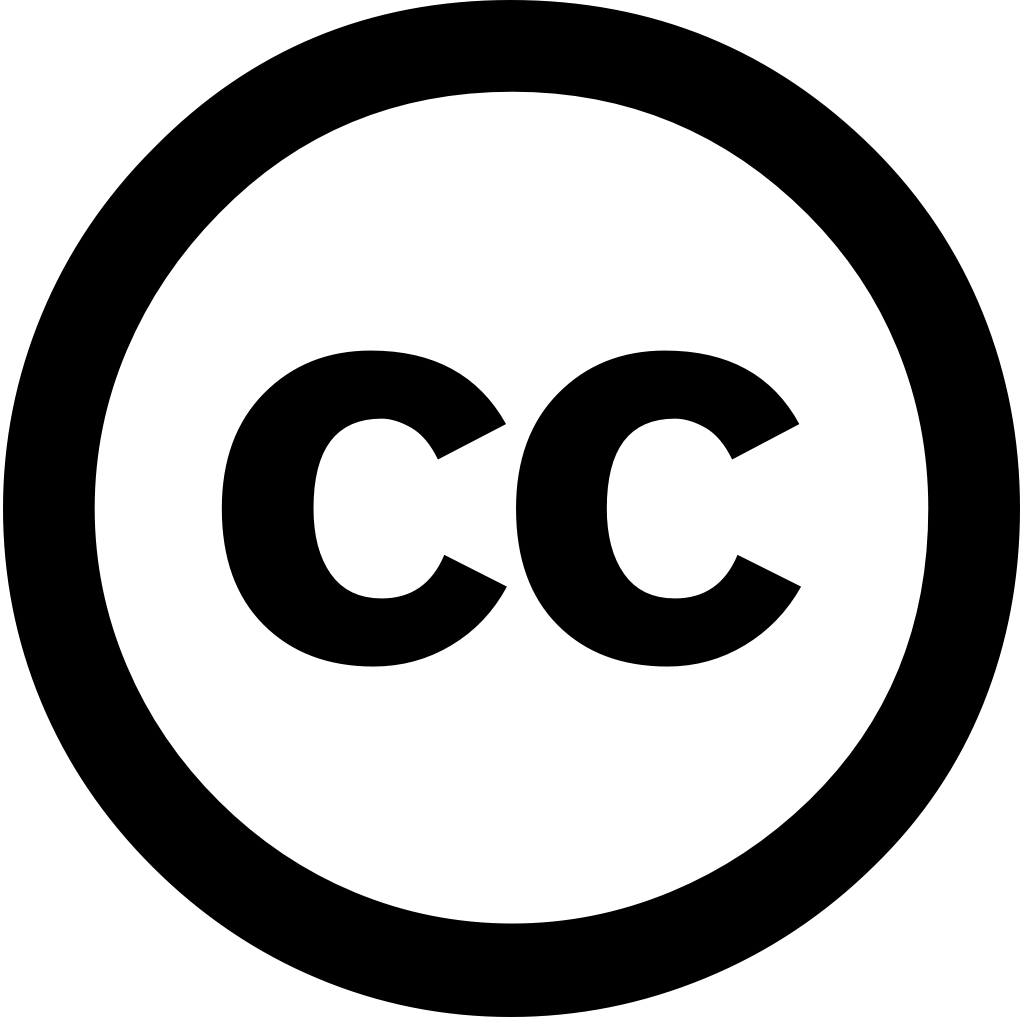
Discover Geoscience, Journal Year: 2024, Volume and Issue: 2(1)
Published: Oct. 3, 2024
Language: Английский
Discover Geoscience, Journal Year: 2024, Volume and Issue: 2(1)
Published: Oct. 3, 2024
Language: Английский
Physics of Fluids, Journal Year: 2025, Volume and Issue: 37(3)
Published: March 1, 2025
The drilling process can result in irregular measurements due to unconsolidated geological formations, affecting the accuracy of wireline logging devices. This impacts precision elastic log measurements, such as velocity and density profiles, which are essential for reservoir characterization. reliability wireline-logging tool is crucial preventing inaccuracies when assessing rock properties. Previous studies have focused on applying machine learning (ML) techniques logging, but these methods limited applicability, particularly outlier detection reconstruction. In response, this study integrates both supervised unsupervised ML enhance responses Initially, density-based spatial clustering applications with noise was applied detection, followed by feature selection identify correlated logs reconstructing log. A random forest regression model, optimized particle swarm optimization (PSO), then trained using selected features. comparative analysis showed a significant improvement porosity estimation from reconstructed compared core data. Specifically, comparison between original bulk yielded an R2 0.95 root mean squared error (RMSE) 0.012. contrast, rebuilt resulted 0.98 RMSE 0.007. integration advanced PSO-optimized models represents considerable advancement field approach enhances also saves time reduces manual effort, highlighting potential petroleum exploration production.
Language: Английский
Citations
2Mathematical Geosciences, Journal Year: 2025, Volume and Issue: unknown
Published: Jan. 14, 2025
Language: Английский
Citations
1Physics of Fluids, Journal Year: 2025, Volume and Issue: 37(3)
Published: March 1, 2025
This study focuses on the classification of Hydraulic Flow Units (HFUs) within Lower Goru reservoir using a hybrid modeling approach for more precise and data-driven characterization. The methodology begins with K-means clustering, which groups into distinct HFUs based properties. To enhance accuracy this classification, Particle Swarm Optimization (PSO) is employed to optimize clustering process. flow capacity rock quality each HFU are then assessed two key indicators: zone indicator (FZI) index (RQI). results reveal four HFUs: Clean Sandstone, Clayey Shaly Shale. Among these, 1 (Clean Sandstone) exhibits highest FZI RQI values, indicating excellent capacity, while 2 (Clayey demonstrates moderate suggesting good potential. In contrast, 3 (Shaly 4 (Shale) show progressively lower reflecting poorer reduced integrated significantly improves precision characterization by combining PSO optimization, petrophysical indicators such as RQI. study's findings not only provide valuable understanding dynamics fluid potential but also our comprehension spatial distribution properties HFU, offering solid foundation optimizing hydrocarbon recovery enhancing management approaches.
Language: Английский
Citations
1Minerals, Journal Year: 2025, Volume and Issue: 15(3), P. 240 - 240
Published: Feb. 26, 2025
The lithofacies of a reservoir contain key information such as rock lithology, sedimentary structures, and mineral composition. Accurate prediction shale is crucial for identifying sweet spots oil gas development. However, obtaining through core sampling during drilling challenging, the accuracy traditional logging curve intersection methods insufficient. To efficiently accurately predict lithofacies, this study proposes hybrid model called Stacking, which combines four classifiers: Random Forest, HistGradient Boosting, Extreme Gradient Categorical Boosting. employs Grid Search Method to automatically search optimal hyperparameters, using classifiers base learners. predictions from these learners are then used new features, Logistic Regression serves final meta-classifier prediction. A total 3323 data points were collected six wells train test model, with performance evaluated on two blind that not involved in training process. results indicate stacking predicts achieving an Accuracy, Recall, Precision, F1 Score 0.9587, 0.959, respectively, set. This achievement provides technical support evaluation spot exploration.
Language: Английский
Citations
0Carbonates and Evaporites, Journal Year: 2025, Volume and Issue: 40(2)
Published: May 6, 2025
Language: Английский
Citations
0Journal of Sedimentary Environments, Journal Year: 2025, Volume and Issue: unknown
Published: May 13, 2025
Language: Английский
Citations
0International Journal of Coal Geology, Journal Year: 2024, Volume and Issue: 296, P. 104644 - 104644
Published: Nov. 4, 2024
Language: Английский
Citations
1Discover Geoscience, Journal Year: 2024, Volume and Issue: 2(1)
Published: Oct. 3, 2024
Language: Английский
Citations
0