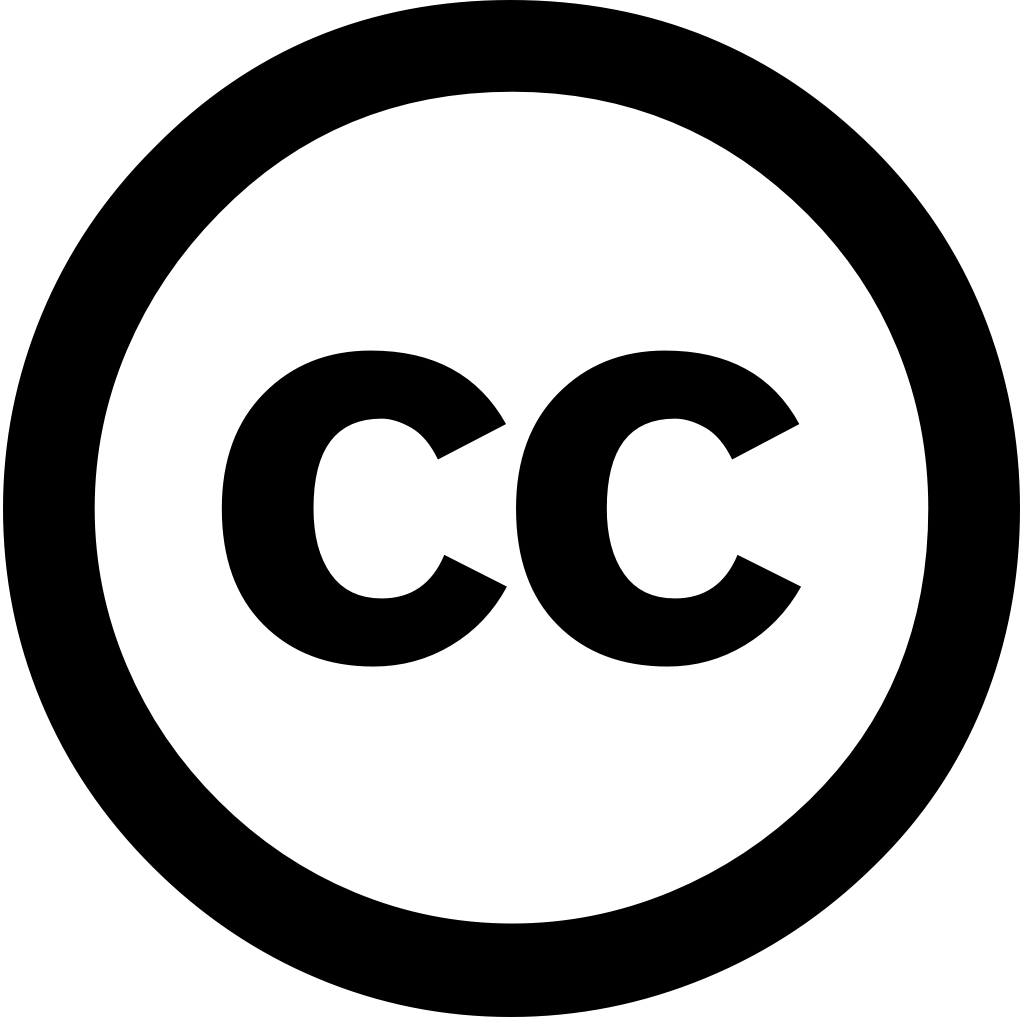
Smart Agricultural Technology, Journal Year: 2024, Volume and Issue: unknown, P. 100728 - 100728
Published: Dec. 1, 2024
Language: Английский
Smart Agricultural Technology, Journal Year: 2024, Volume and Issue: unknown, P. 100728 - 100728
Published: Dec. 1, 2024
Language: Английский
Journal of Hazardous Materials Advances, Journal Year: 2025, Volume and Issue: 17, P. 100604 - 100604
Published: Jan. 15, 2025
Language: Английский
Citations
1Agriculture, Journal Year: 2024, Volume and Issue: 14(11), P. 2053 - 2053
Published: Nov. 14, 2024
Cadmium (Cd) is a highly toxic metal that difficult to completely eliminate from soil, despite advancements in modern agricultural and environmental technologies have successfully reduced Cd levels. However, rice remains key source of exposure for humans. Even small amounts absorbed by can pose potential health risk the human body. Laser-induced breakdown spectroscopy (LIBS) has advantages simple sample preparation fast analysis, which, combined with transfer learning method, expected realize real-time rapid detection low-level heavy metals rice. In this work, 21 groups naturally matured samples potentially Cd-contaminated environments were collected. These processed into husk, brown rice, polished groups, reference content was measured ICP-MS. The XGBoost algorithm, known its excellent performance handling high-dimensional data nonlinear relationships, applied construct both base model XGBoost-based predict By pre-training on husk data, learn abundant information available improve quantification grain. For achieved RC2 0.9852 RP2 0.8778, which improved 0.9885 0.9743, respectively, model. case 0.9838 0.8683, while enhanced these 0.9883 0.9699, respectively. results indicate method not only improves capability low but also provides new insights food safety detection.
Language: Английский
Citations
0Smart Agricultural Technology, Journal Year: 2024, Volume and Issue: unknown, P. 100728 - 100728
Published: Dec. 1, 2024
Language: Английский
Citations
0