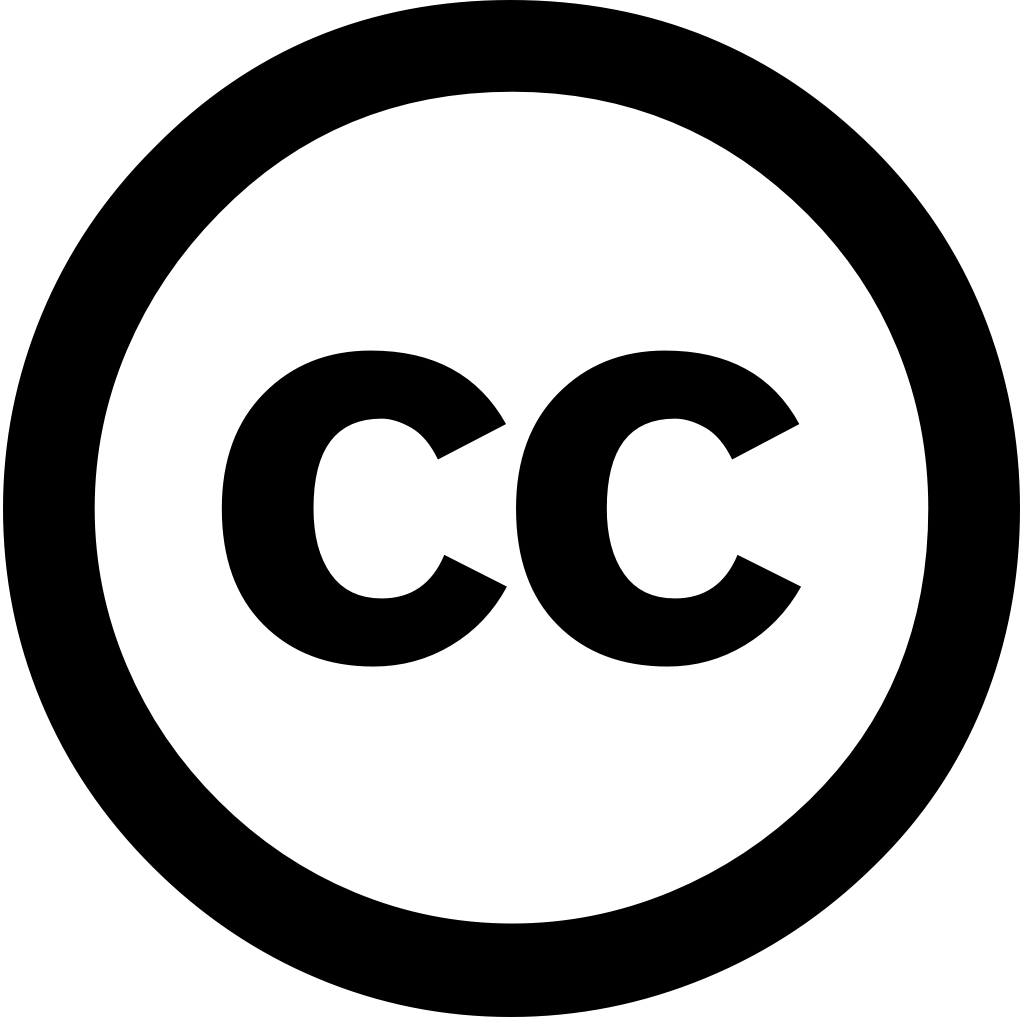
Results in Engineering, Journal Year: 2024, Volume and Issue: 23, P. 102759 - 102759
Published: Aug. 23, 2024
Language: Английский
Results in Engineering, Journal Year: 2024, Volume and Issue: 23, P. 102759 - 102759
Published: Aug. 23, 2024
Language: Английский
Journal of Computing in Civil Engineering, Journal Year: 2025, Volume and Issue: 39(2)
Published: Jan. 11, 2025
Language: Английский
Citations
2Lecture notes in mechanical engineering, Journal Year: 2025, Volume and Issue: unknown, P. 81 - 95
Published: Jan. 1, 2025
Language: Английский
Citations
0Ocean Engineering, Journal Year: 2025, Volume and Issue: 323, P. 120410 - 120410
Published: Feb. 6, 2025
Language: Английский
Citations
0Water Resources Management, Journal Year: 2025, Volume and Issue: unknown
Published: Feb. 11, 2025
Language: Английский
Citations
0Ocean Engineering, Journal Year: 2025, Volume and Issue: 327, P. 121005 - 121005
Published: March 22, 2025
Language: Английский
Citations
0Sustainable civil infrastructures, Journal Year: 2025, Volume and Issue: unknown, P. 272 - 278
Published: Jan. 1, 2025
Language: Английский
Citations
0Particulate Science And Technology, Journal Year: 2025, Volume and Issue: unknown, P. 1 - 15
Published: April 24, 2025
Language: Английский
Citations
0Advances in Structural Engineering, Journal Year: 2025, Volume and Issue: unknown
Published: March 19, 2025
Local scour is one of the main reasons for bridge collapse. To solve difficult problem detecting local depth underwater pier structures, this paper explores an optimal method predicting structures based on various ensemble learning methods. Firstly, collects 487 sets data samples containing nine input parameters with corresponding depths from open-source database in practical project. Secondly, employs five algorithms commonly used learning, that is, Random Forest (RF), Gradient Boosted Decision Tree (GBDT), Extreme Boosting (XGBoost), Adaptive (AdaBoost), and Light Machine (LightGBM), to build a prediction model depth. In addition, Bayesian hyperparameter optimization applied search best combination model. Then, eight evaluation indices, including Mean Absolute Error (MAE), Bias (MBE), Percentage (MAPE), Root Square (RMSE), coefficient determination (R 2 ), Nash-Sutcliffe Efficiency (NSE), Percent (Pbias), Willmott Index (WI), were compare analyse established model, importance coefficients each parameter evaluated Finally, Conditional Generative Adversarial Network (CGAN) was augment supplement existing database, verify its effectiveness. The results show parameter-optimized LightGBM achieves performance. Moreover, CGAN can effectively insufficient lack specific sample data.
Language: Английский
Citations
0Physics of Fluids, Journal Year: 2025, Volume and Issue: 37(4)
Published: April 1, 2025
In this research, an assessment of scour depth prediction in meandering channels with spur dikes is made employing machine learning approaches. Efficient determination the therefore vital morphologic aspects and structural stability. The input parameters include sinuosity (S), dike locations (Ld), porosity (P) experimental data from sinusoidal flumes. Four models; Extreme Gradient Boosting (XGBoost) Particle Swarm Optimization (PSO) XGBoost-PSO, Random Forest (RF), k-Nearest Neighbors (k-NN), Decision Tree-Neural Network (DT-NN) were used compared. findings demonstrate R-value 0.995 case RF model while XGBoost-PSO gave second-best accuracy R = 0.988. results SHAP analysis illustrated that are significant factors affecting (Ds/Yn, Ds: depth, Yn: water depth) had moderate importance assigned to location. Kernel density plots further supported regarding error distribution consistency. Even though, both yielded better because hyperparameter tuning, k-NN DT-NN less precise outcomes specifically predicted for progressive hydraulic procedures. Taylor's diagram even revealed greater by RF. Hence, a proper selection appropriate models remains first step estimating sufficiently flood erosion control.
Language: Английский
Citations
0Frontiers in Earth Science, Journal Year: 2025, Volume and Issue: 13
Published: April 25, 2025
Accurate prediction of water inrush volumes is essential for safeguarding tunnel construction operations. This study proposes a method predicting volumes, leveraging the eXtreme Gradient Boosting (XGBoost) model optimized with Bayesian techniques. To maximize utility available data, 654 datasets missing values were imputed and augmented, forming robust dataset training validation XGBoost (BO-XGBoost) model. Furthermore, SHapley Additive explanations (SHAP) was employed to elucidate contribution each input feature predictive outcomes. The results indicate that: (1) constructed BO-XGBoost exhibited exceptionally high accuracy on test set, root mean square error (RMSE) 7.5603, absolute (MAE) 3.2940, percentage (MAPE) 4.51%, coefficient determination (R 2 ) 0.9755; (2) Compared performance support vector mechine (SVR), decision tree (DT), random forest (RF) models, demonstrates highest R smallest error; (3) importance yielded by SHAP groundwater level ( h > water-producing characteristics W burial depth H rock mass quality index RQD ). proposed volume dataset, thereby aiding managers in making informed decisions mitigate risks ensuring safe efficient advancement projects.
Language: Английский
Citations
0