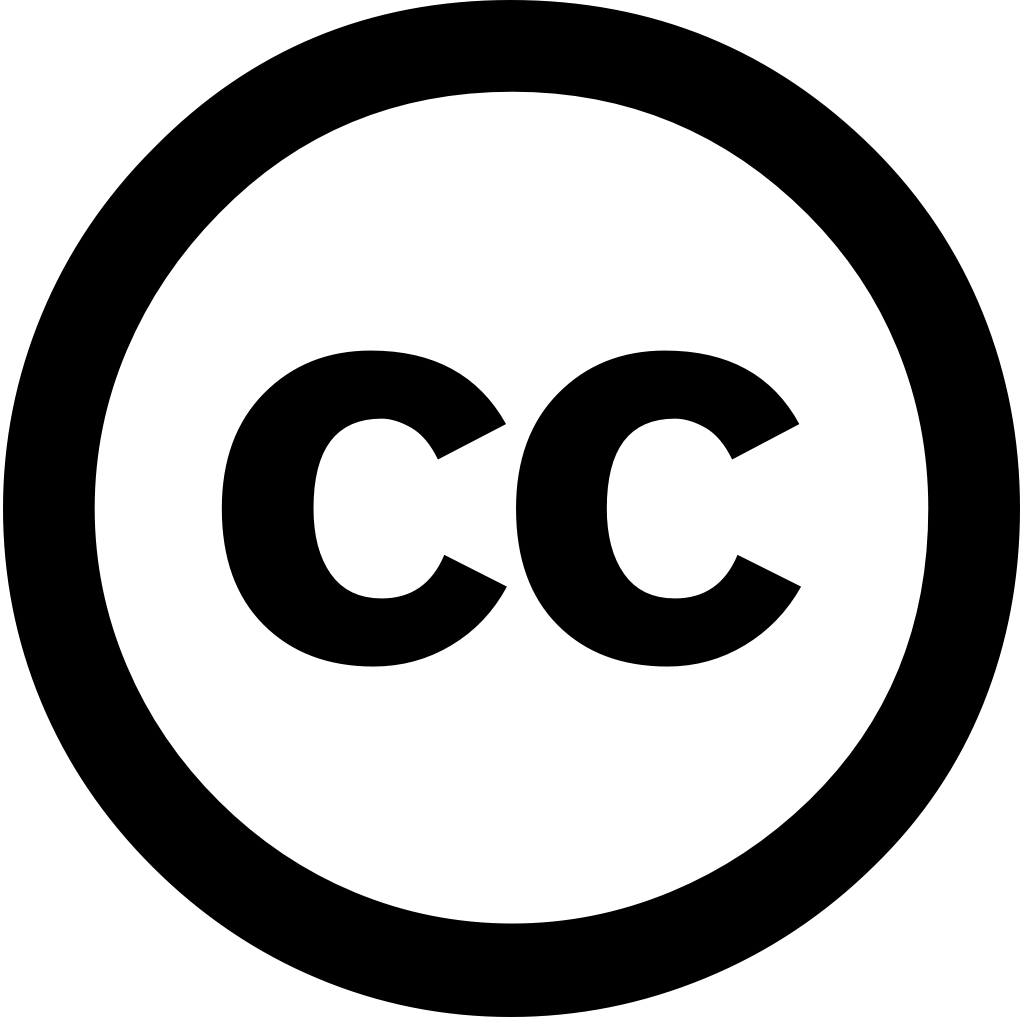
Ecological Indicators, Journal Year: 2024, Volume and Issue: 169, P. 112940 - 112940
Published: Dec. 1, 2024
Language: Английский
Ecological Indicators, Journal Year: 2024, Volume and Issue: 169, P. 112940 - 112940
Published: Dec. 1, 2024
Language: Английский
Water Research, Journal Year: 2025, Volume and Issue: 274, P. 123156 - 123156
Published: Jan. 18, 2025
Language: Английский
Citations
2Environments, Journal Year: 2025, Volume and Issue: 12(3), P. 75 - 75
Published: March 1, 2025
Rising nitrate contamination in water systems poses significant risks to public health and ecosystem stability, necessitating advanced modeling understand dynamics more accurately. This study applies the long short-term memory (LSTM) investigate hydrologic environmental factors influencing concentration rivers aquifers across state of Alabama southeast United States. By integrating dynamic data such as streamflow groundwater levels with static catchment attributes, machine learning model identifies primary drivers fluctuations, offering detailed insights into complex interactions affecting multi-year concentrations natural aquatic systems. In addition, a novel LSTM-based approach utilizes synthetic surface predict levels, helping address monitoring gaps connected these rivers. method reveals potential correlations between dynamics, which is particularly meaningful given lack quality observations many aquifers. Field applications further show that, while LSTM effectively captures seasonal trends, limitations representing extreme events suggest areas for refinement. These findings contribute data-driven management, enhancing understanding behavior interconnected
Language: Английский
Citations
0Frontiers in Environmental Science, Journal Year: 2025, Volume and Issue: 13
Published: March 7, 2025
Nitrogen pollution in aquatic ecosystems, primarily from agricultural sources, presents significant environmental challenges. At the land management decision level, reducing nitrate leaching requires knowledge of loading over time and location, complexity which is amplified by limited data availability, especially poorly gauged watersheds. This issue particularly pronounced cold humid regions where water quality are often collected during growing season only. Large gaps result systematic errors when estimating nitrogen load based on traditional regression methods. In this study, we explore feasibility using process-based hydrologic model to estimate loads sparse temporal a coastal watershed Atlantic Canada compared its performance with three We found that absence available 16% non-growing 10-year study period can lead biases (as high as 21%) estimation contrast, estimates obtained Soil Water Assessment Tool (SWAT) were less sensitive gaps. The results suggest models like SWAT be viable alternative for available. As agri-environmental issues become more pressing, it crucial use appropriate methods availability avoid misleading results.
Language: Английский
Citations
0Ecological Indicators, Journal Year: 2024, Volume and Issue: 169, P. 112853 - 112853
Published: Nov. 16, 2024
Language: Английский
Citations
3Ecological Indicators, Journal Year: 2024, Volume and Issue: 169, P. 112940 - 112940
Published: Dec. 1, 2024
Language: Английский
Citations
0