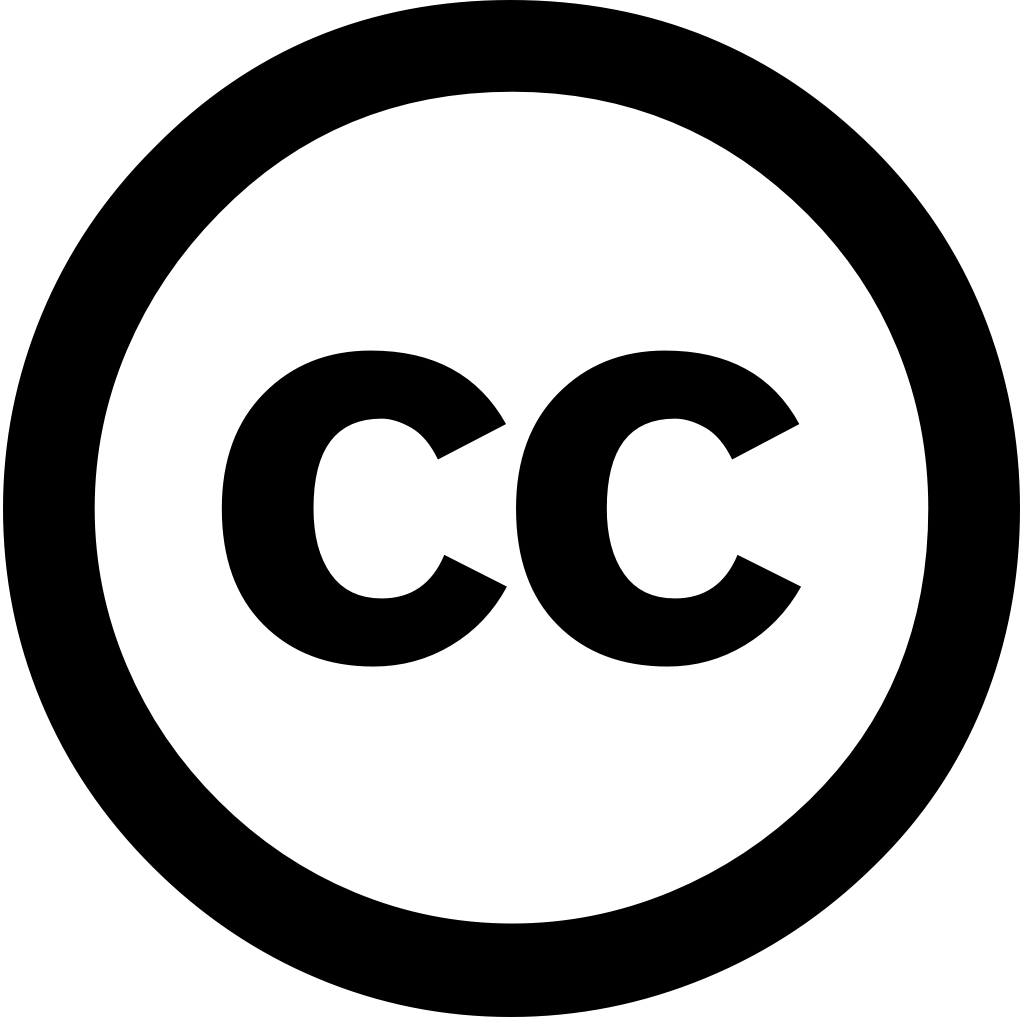
Agriculture, Journal Year: 2025, Volume and Issue: 15(9), P. 1015 - 1015
Published: May 7, 2025
Seed potatoes without sprouts usually need to be manually selected in mechanized production, which has been the bottleneck of efficiency. A fast and efficient object recognition algorithm is required for additional removal process identify unqualified seed potatoes. In this paper, a lightweight deep learning algorithm, YOLOv8_EBG, proposed both improve detection performance reduce model parameters. The ECA attention mechanism was introduced backbone neck more accurately extract fuse sprouting features. To further parameters, Ghost convolution C3ghost were replace normal C2f blocks vanilla YOLOv8n. addition, bi-directional feature pyramid network integrated part multi-scale fusion enhance accuracy. experimental results from an isolated test dataset show that performs better detecting under natural light conditions, achieving mAP0.5 95.7% 91.9% AP bud recognition. Compared YOLOv8n model, improved showed 6.5% increase mAP0.5, 12.9% AP0.5 recognition, 5.6% decrease number Additionally, applied tested on sorting equipment, accuracy potato as high 92.5%, sufficient select sprouted potatoes, indispensable step since only can used study provide technical support subsequent planting intelligence.
Language: Английский