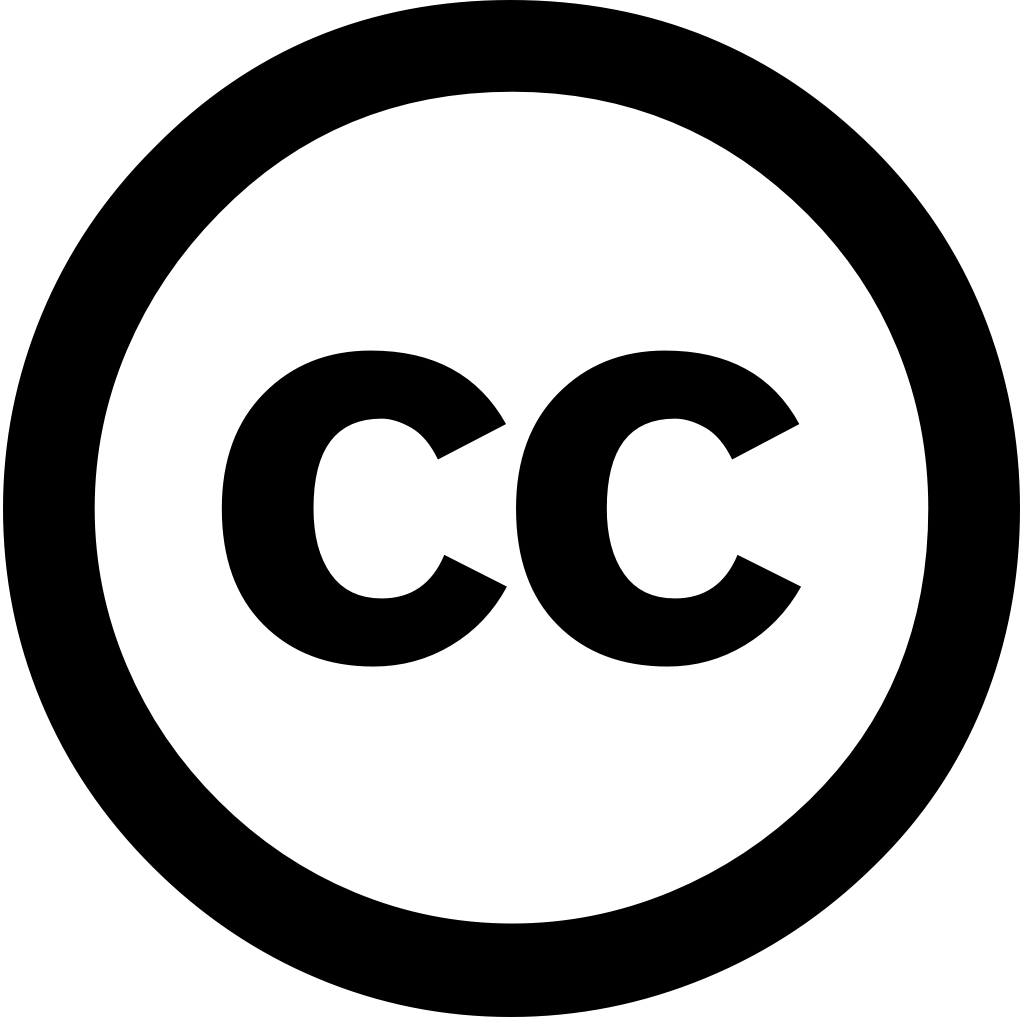
Resources Environment and Sustainability, Journal Year: 2024, Volume and Issue: unknown, P. 100177 - 100177
Published: Nov. 1, 2024
Language: Английский
Resources Environment and Sustainability, Journal Year: 2024, Volume and Issue: unknown, P. 100177 - 100177
Published: Nov. 1, 2024
Language: Английский
Journal of Hydrology, Journal Year: 2024, Volume and Issue: 631, P. 130772 - 130772
Published: Feb. 2, 2024
Language: Английский
Citations
14Journal of Hydrology, Journal Year: 2024, Volume and Issue: 637, P. 131389 - 131389
Published: May 19, 2024
Language: Английский
Citations
14Water, Journal Year: 2024, Volume and Issue: 16(13), P. 1904 - 1904
Published: July 3, 2024
Machine learning (ML) applications in hydrology are revolutionizing our understanding and prediction of hydrological processes, driven by advancements artificial intelligence the availability large, high-quality datasets. This review explores current state ML hydrology, emphasizing utilization extensive datasets such as CAMELS, Caravan, GRDC, CHIRPS, NLDAS, GLDAS, PERSIANN, GRACE. These provide critical data for modeling various parameters, including streamflow, precipitation, groundwater levels, flood frequency, particularly data-scarce regions. We discuss type methods used significant successes achieved through those models, highlighting their enhanced predictive accuracy integration diverse sources. The also addresses challenges inherent applications, heterogeneity, spatial temporal inconsistencies, issues regarding downscaling LSH, need incorporating human activities. In addition to discussing limitations, this article highlights benefits utilizing high-resolution compared traditional ones. Additionally, we examine emerging trends future directions, real-time quantification uncertainties improve model reliability. place a strong emphasis on citizen science IoT collection hydrology. By synthesizing latest research, paper aims guide efforts leveraging large techniques advance enhance water resource management practices.
Language: Английский
Citations
9Journal of Hydrology, Journal Year: 2024, Volume and Issue: unknown, P. 132223 - 132223
Published: Oct. 1, 2024
Language: Английский
Citations
9Water, Journal Year: 2024, Volume and Issue: 16(21), P. 3153 - 3153
Published: Nov. 4, 2024
Stream gauge height (water level) is a significant indicator for forecasting future floods. Flooding occurs when the water level exceeds flood stage. Predicting imminent floods can save lives, protect infrastructure, and improve road traffic management transportation. Deep neural networks have been increasingly used in this domain due to their predictive capabilities capturing complex features interdependencies. This study employs four distinct models—Multi-Layer Perceptron (MLP), Long Short-Term Memory (LSTM), transformer, LSTNet—with MLP serving as baseline model forecast levels. The models are trained using data from 20 river gages across state of Missouri ensure consistent performance. Random search optimization employed hyperparameter tuning. prediction intervals set at 4, 6, 8, 10 (each interval equivalent 30 min) that performance results robust not random weight initialization or suboptimal hyperparameters throughout different intervals. findings indicate LSTNet leads better than other models, with median RMSE 0.00724, 0.00959, 0.01204, 0.01230 intervals, respectively. As climate change localized storms driven by atmospheric shifts, fluctuations becoming extreme, further exacerbating drift real-world datasets. demonstrates superior terms RMSE, MAE, correlation coefficient all levels under conditions.
Language: Английский
Citations
8Advances in Water Resources, Journal Year: 2023, Volume and Issue: 179, P. 104520 - 104520
Published: Aug. 12, 2023
Language: Английский
Citations
16Earth Science Informatics, Journal Year: 2024, Volume and Issue: unknown
Published: June 10, 2024
Language: Английский
Citations
5Water, Journal Year: 2022, Volume and Issue: 14(22), P. 3672 - 3672
Published: Nov. 14, 2022
In recent decades, natural calamities such as drought and flood have caused widespread economic social damage. Climate change rapid urbanization contribute to the occurrence of disasters. addition, their destructive impact has been altered, posing significant challenges efficiency, equity, sustainability water resources allocation management. Uncertainty estimation in hydrology is essential for By quantifying associated uncertainty reliable hydrological forecasting, an efficient management plan obtained. Moreover, forecasting provides future information assist risk assessment. Currently, majority forecasts utilize deterministic approaches. Nevertheless, models cannot account intrinsic forecasted values. Using Bayesian deep learning approach, this study developed a probabilistic model that covers pertinent subproblem univariate time series multi-step ahead daily streamflow quantify epistemic aleatory uncertainty. The new implements sampling Long short-term memory (LSTM) neural network by using variational inference approximate posterior distribution. proposed method verified with three case studies USA horizons. LSTM point models, LSTM-BNN, BNN, Monte Carlo (MC) dropout (LSTM-MC), were applied comparison model. results show long (BLSTM) outperforms other terms reliability, sharpness, overall performance. reveal all outperformed lower RMSE value. Furthermore, BLSTM can handle data higher variation peak, particularly long-term compared models.
Language: Английский
Citations
20International Journal of Digital Earth, Journal Year: 2024, Volume and Issue: 17(1)
Published: Aug. 19, 2024
Deep learning (DL) has demonstrated strong potential in addressing key challenges spatiotemporal forecasting across various Earth system science (ESS) domains. This review examines 69 studies applying DL to tasks within climate modeling and weather prediction, disaster management, air quality modeling, hydrological renewable energy forecasting, oceanography, environmental monitoring. We summarize commonly used architectures for ESS, technical innovations, the latest advancements predictive applications. While have proven capable of handling data, remain tackling complexities specific such as complex scale dependencies, model interpretability, integration physical knowledge. Recent innovations demonstrate growing efforts integrate knowledge, improve explainability, adapt domain-specific needs, quantify uncertainties. Finally, this highlights future directions, including (1) developing more interpretable hybrid models that synergize traditional approaches, (2) extending generalizability through techniques like domain adaptation transfer learning, (3) advancing methods uncertainty quantification missing data handling.
Language: Английский
Citations
4Sustainability, Journal Year: 2024, Volume and Issue: 16(19), P. 8699 - 8699
Published: Oct. 9, 2024
The establishment of an accurate and reliable predictive model is essential for water resources planning management. Standalone models, such as physics-based hydrological models or data-driven have their specific applications, strengths, limitations. In this study, a hybrid (namely SWAT-Transformer) was developed by coupling the Soil Water Assessment Tool (SWAT) with Transformer to enhance monthly streamflow prediction accuracy. SWAT first constructed calibrated, then its outputs are used part inputs Transformer. By correcting errors using Transformer, two effectively coupled. Monthly runoff data at Yan’an Ganguyi stations on Yan River, first-order tributary Yellow River Basin, were evaluate proposed model’s performance. results indicated that performed well in predicting high flows but poorly low flows. contrast, able capture low-flow period information more accurately outperformed overall. SWAT-Transformer could correct predictions overcome limitations single model. integrating SWAT’s detailed physical process portrayal Transformer’s powerful time-series analysis, coupled significantly improved offer optimal resource management, which crucial sustainable economic societal development.
Language: Английский
Citations
4