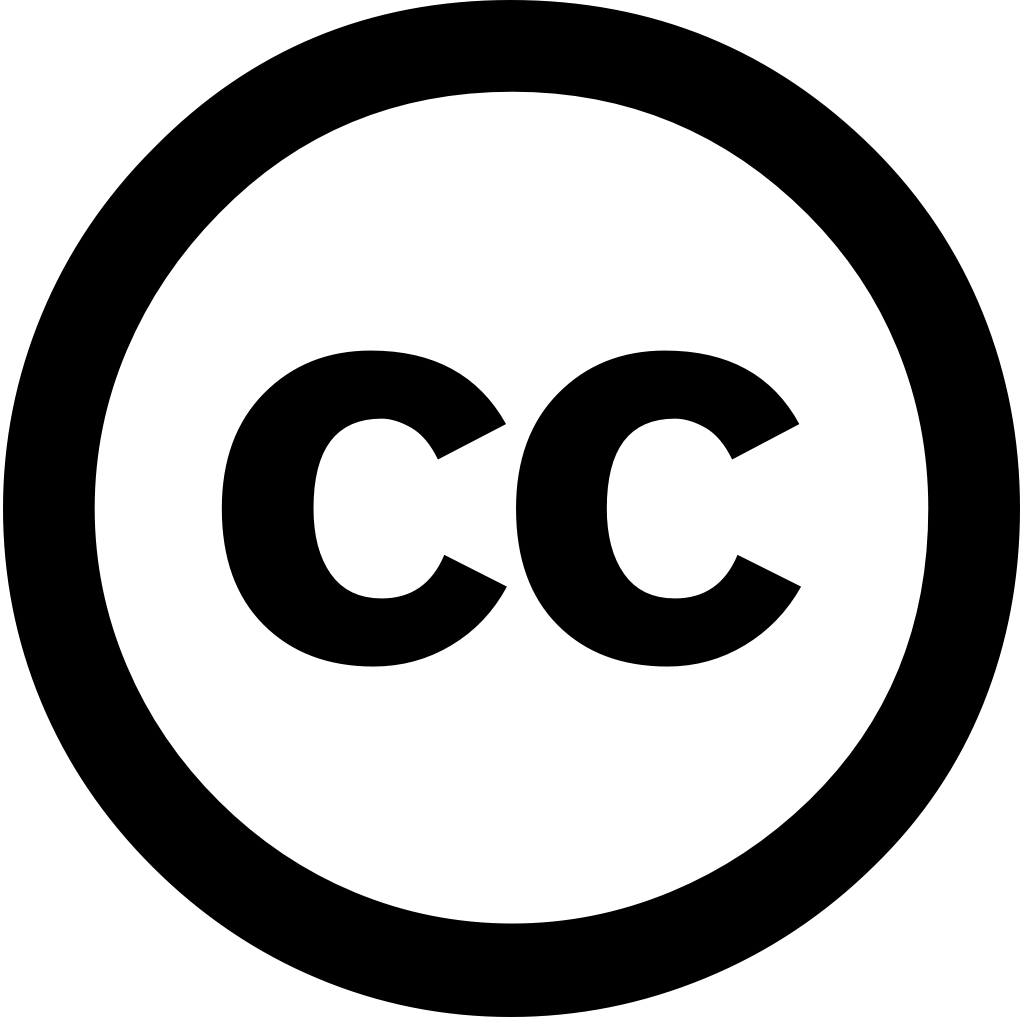
Journal of Hydrology Regional Studies, Journal Year: 2024, Volume and Issue: 57, P. 102119 - 102119
Published: Dec. 13, 2024
Language: Английский
Journal of Hydrology Regional Studies, Journal Year: 2024, Volume and Issue: 57, P. 102119 - 102119
Published: Dec. 13, 2024
Language: Английский
Journal of Hydrology, Journal Year: 2024, Volume and Issue: 643, P. 131996 - 131996
Published: Sept. 16, 2024
Language: Английский
Citations
14Agronomy, Journal Year: 2024, Volume and Issue: 14(9), P. 1998 - 1998
Published: Sept. 2, 2024
Soil, a non-renewable resource, requires continuous monitoring to prevent degradation and support sustainable agriculture. Visible-near-infrared (Vis-NIR) spectroscopy is rapid cost-effective method for predicting soil properties. While traditional machine learning methods are commonly used modeling Vis-NIR spectral data, large datasets may benefit more from advanced deep techniques. In this study, based on the library LUCAS, we aimed enhance regression model performance in property estimation by combining Transformer convolutional neural network (CNN) techniques predict 11 properties (clay, silt, pH CaCl2, H2O, CEC, OC, CaCO3, N, P, K). The Transformer-CNN accurately predicted most properties, outperforming other (partial least squares (PLSR), random forest (RFR), vector (SVR), Long Short-Term Memory (LSTM), ResNet18) with 10–24 percentage point improvement coefficient of determination (R2). excelled N (R2 = 0.94–0.96, RPD > 3) performed well clay, sand, K 0.77–0.85, 2 < 3). This study demonstrates potential enhancing prediction, although future work should aim optimize computational efficiency explore wider range applications ensure its utility different agricultural settings.
Language: Английский
Citations
6Applied Sciences, Journal Year: 2025, Volume and Issue: 15(2), P. 503 - 503
Published: Jan. 7, 2025
Soil Organic Matter (SOM) is crucial for soil fertility, and effective detection methods are of great significance the development agriculture forestry. This study uses 206 hyperspectral samples from state-owned Yachang Huangmian Forest Farms in Guangxi, using SPXY algorithm to partition dataset a 4:1 ratio, provide an spectral data preprocessing method novel SOM content prediction model area similar regions. Three denoising (no denoising, Savitzky–Golay filter discrete wavelet transform denoising) were combined with nine mathematical transformations (original reflectance (R), first-order differential (1DR), second-order (2DR), MSC, SNV, logR, (logR)′, 1/R, ((1/R)′) form 27 combinations. Through Pearson heatmap analysis modeling accuracy comparison, SG-1DR combination was found effectively highlight features. A CNN-SVM based on Black Kite Algorithm (BKA) proposed. leverages powerful parameter tuning capabilities BKA, CNN feature extraction, SVM classification regression, further improving prediction. The results RMSE = 3.042, R2 0.93, MAE 4.601, MARE 0.1, MBE 0.89, PRIQ 1.436.
Language: Английский
Citations
0Journal of Hydrology, Journal Year: 2025, Volume and Issue: unknown, P. 132737 - 132737
Published: Jan. 1, 2025
Language: Английский
Citations
0Journal of Hydrology, Journal Year: 2025, Volume and Issue: unknown, P. 132887 - 132887
Published: Feb. 1, 2025
Language: Английский
Citations
0Journal of Hydrology, Journal Year: 2025, Volume and Issue: unknown, P. 133328 - 133328
Published: April 1, 2025
Language: Английский
Citations
0Journal of Hydrology, Journal Year: 2024, Volume and Issue: 640, P. 131668 - 131668
Published: July 17, 2024
Language: Английский
Citations
1Journal of Hydrology, Journal Year: 2024, Volume and Issue: unknown, P. 132047 - 132047
Published: Sept. 1, 2024
Language: Английский
Citations
1Journal of Hydrology Regional Studies, Journal Year: 2024, Volume and Issue: 57, P. 102119 - 102119
Published: Dec. 13, 2024
Language: Английский
Citations
0