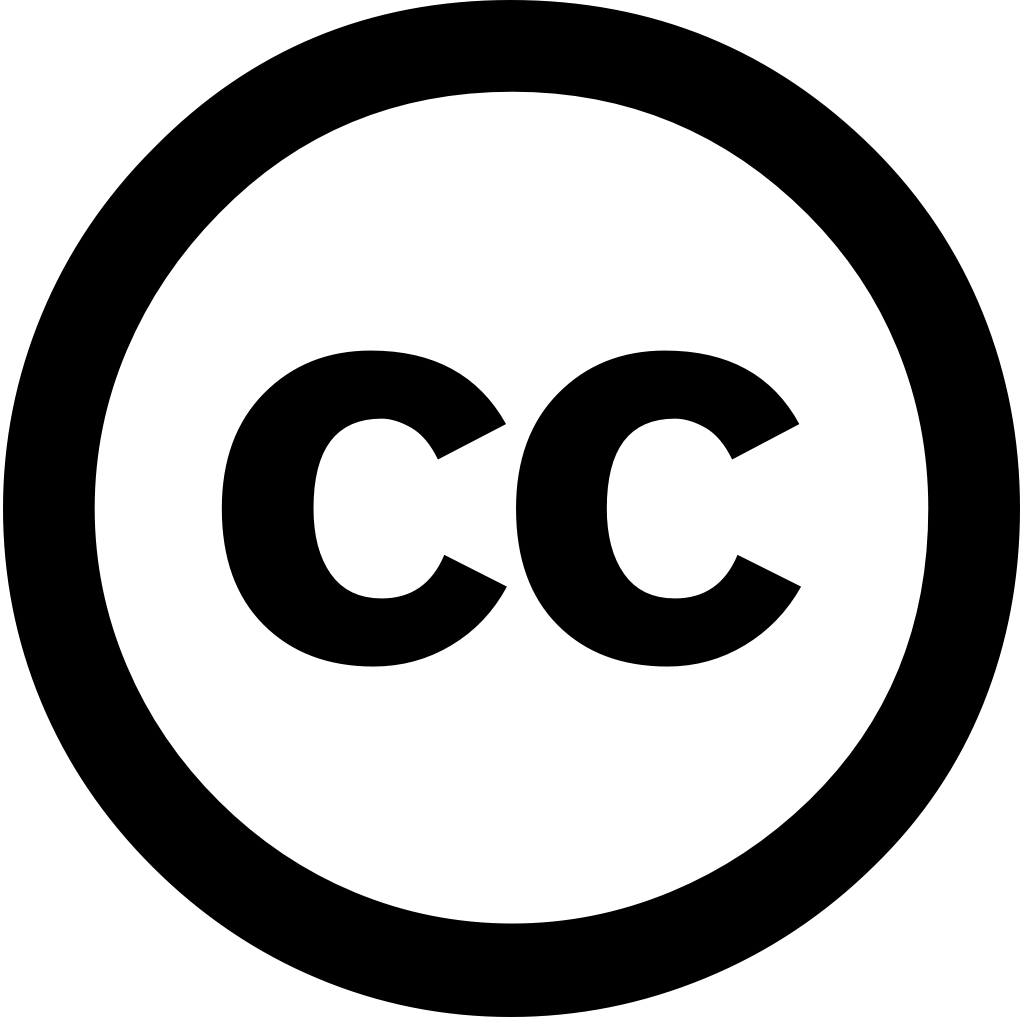
Water Resources Research, Journal Year: 2024, Volume and Issue: 60(8)
Published: Aug. 1, 2024
Abstract The depth‐wise distribution of root water uptake is typically inferred through linear mixing models that utilize knowledge stable isotopes in soil and plants. However, these existing often represent the profile discrete segments, potentially introducing significant uncertainty bias into results. In this study, we introduced a novel model combines Bayesian framework with continuous pattern, named CrisPy. To evaluate performance CrisPy, conducted virtual field‐based tests under several types prior information. CrisPy showed accurate robust reconstruction true various information settings test. By contrast, model, MixSIAR was greatly influenced by deviated from profile. mean squared error proportions ranged 3.6% to 7.4%, while exhibited values 6.3%–15.2%. Furthermore, posterior predictive checking indicated effectively reconstructed standard deviations plant isotopic compositions both tests. MixSIAR, however, underestimated overestimated deviation compositions. These findings collectively support enhanced accuracy, greater robustness, reduced comparison MixSIAR. Therefore, provides powerful tool for partitioning sources.
Language: Английский