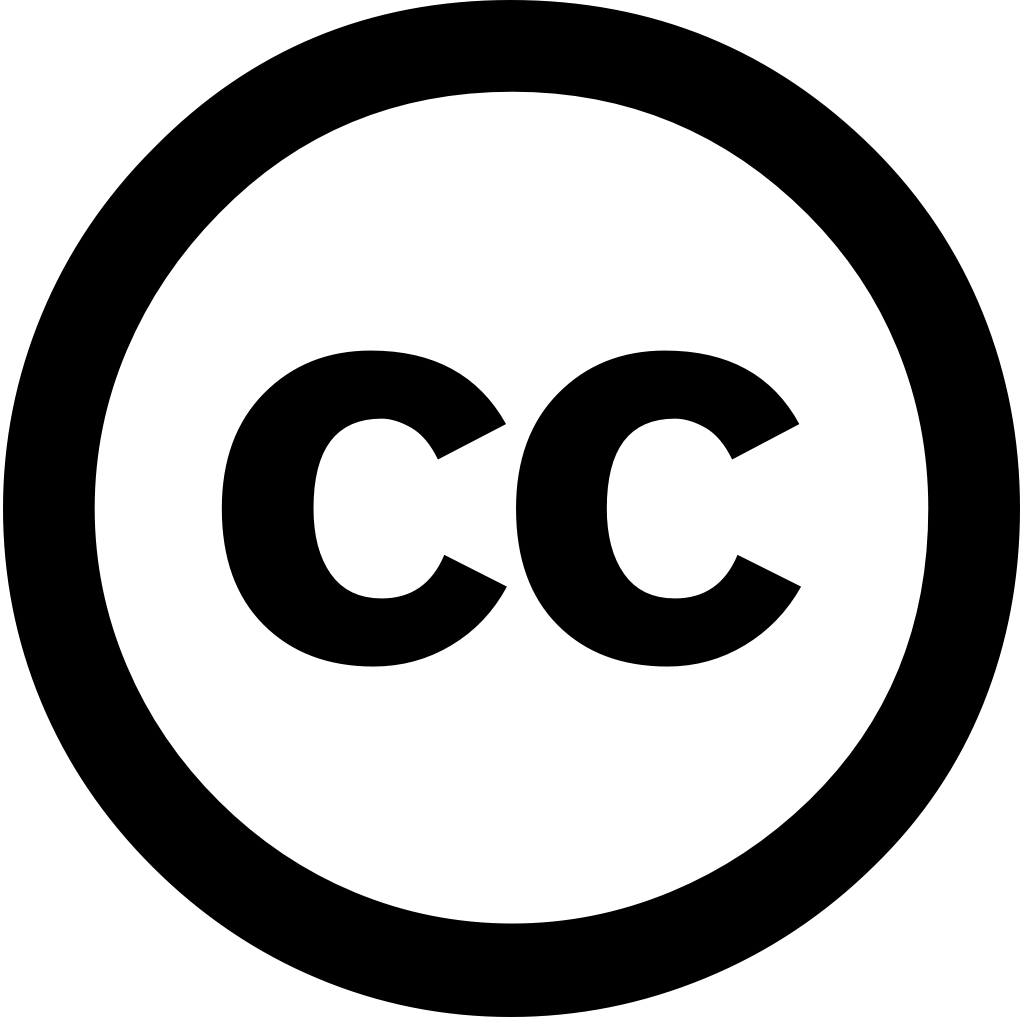
Machine Learning Science and Technology, Journal Year: 2024, Volume and Issue: 6(1), P. 015005 - 015005
Published: Dec. 17, 2024
Abstract The complexity and fusion dynamism of the modern industrial chemical sectors have been increasing with rapid progress IR 4.0–5.0. transformative characteristics Industry 4.0–5.0 not fully explored in terms fundamental importance explainability. Traditional monitoring techniques for automatic anomaly detection, identifying potential variables, root cause analysis fault information are intelligent enough to tackle intricate problems real-time practices sectors. This study presents a novel dynamic machine learning based explainable approach address issues process systems. methodology aims detect faults, identify their key causes feature analyze path propagation time magnitude one variable another impact. proposed using domain multivariate granger-entropy-aided independent component (DICA)—distributed canonical correlation approach, incorporating dynamics wrapping supported delay-signed directed graph. utilized application processes verified continuous stirred tank reactor Tennessee Eastman as practical benchmarks. framework’s validations efficiency evaluated established such classic computed ICA DICA standard model scenarios. outcomes results showed that newly developed strategy is preferable previous approaches regarding explainability robust detection identification actual high FDRs low FARs.
Language: Английский