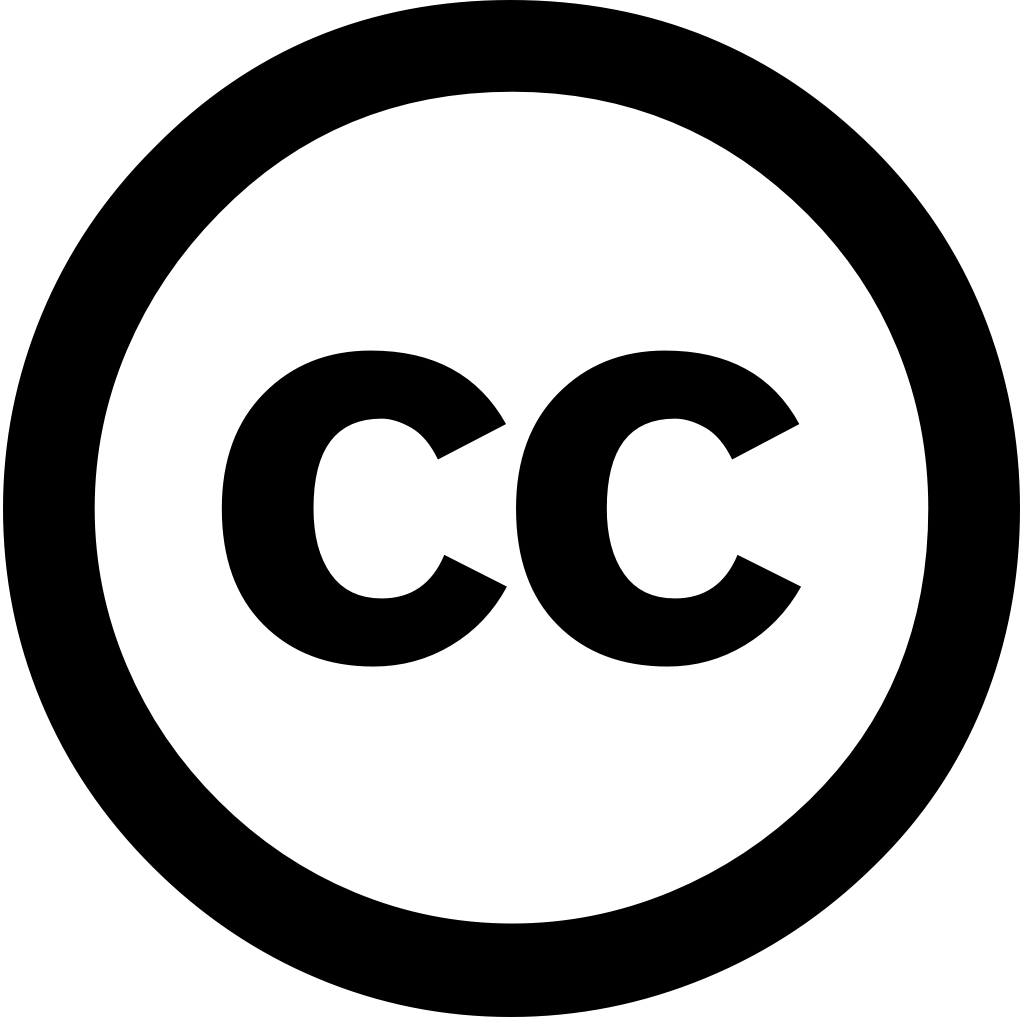
Scientific Reports, Journal Year: 2024, Volume and Issue: 14(1)
Published: Dec. 30, 2024
Accurate estimation of the soil resilient modulus (M
Language: Английский
Scientific Reports, Journal Year: 2024, Volume and Issue: 14(1)
Published: Dec. 30, 2024
Accurate estimation of the soil resilient modulus (M
Language: Английский
International Journal of Hydrogen Energy, Journal Year: 2025, Volume and Issue: 111, P. 781 - 797
Published: Feb. 27, 2025
Language: Английский
Citations
2Composite Structures, Journal Year: 2024, Volume and Issue: 346, P. 118437 - 118437
Published: July 31, 2024
Language: Английский
Citations
6Energy and Buildings, Journal Year: 2024, Volume and Issue: 318, P. 114444 - 114444
Published: June 24, 2024
Language: Английский
Citations
6Superconductor Science and Technology, Journal Year: 2024, Volume and Issue: 37(7), P. 075008 - 075008
Published: May 21, 2024
Abstract The advent of superconducting bulks, due to their compactness and performance, offers new perspectives opportunities in many applications sectors, such as magnetic field shielding, motors/generators, NMR/MRI, bearings, flywheel energy storage, Maglev trains, among others. investigation characterization bulks typically relies on time-consuming expensive experimental campaigns; hence the development effective surrogate models would considerably speed up research progress around them. In this study, we first produced an dataset containing levitation lateral forces between different MgB 2 one permanent magnet under operating conditions. Next, have exploited develop based Artificial Intelligence (AI) techniques, namely Extremely Gradient Boosting, Support Vector Regressor (SVR), Kernel Ridge Regression. After tuning hyperparameters AI models, results demonstrated that SVR is superior technique can predict with a worst-case accuracy scenario 99.86% terms goodness fit data. Moreover, response time these for estimation datapoints ultra-fast.
Language: Английский
Citations
5Materials, Journal Year: 2025, Volume and Issue: 18(1), P. 139 - 139
Published: Jan. 1, 2025
The evaluation of the mechanical performance fly ash-recycled mortar (FARM) is a necessary condition to ensure efficient utilization recycled fine aggregates. This article describes design nine mix proportions FARMs with low water/cement ratio and screens six reasonable flowability. compressive strengths were tested, influence (w/c) age on strength was analyzed. Meanwhile, backpropagation neural network (BPNN) model optimized by grey wolf optimizer (GWO), namely GWO-BPNN model, established predict FARM. input layer consisted w/c, cement/sand ratio, water reducer, age, ash content, while output strength. data set 150 sets from this existing research in literature, which 70% used for training 30% validation. results show that compared traditional BPNN, coefficient determination (R2) increases 0.85 0.93, mean squared error (MSE) decreases 0.018 0.015. convergence iterations validation decrease 108 65. indicates GWO improved prediction accuracy computational efficiency BPNN. characteristic heat, kernel density estimation, scatter matrix, SHAP value all indicated w/c strongly negatively correlated strength, sand/cement positively However, relationship between contents ash, not obvious.
Language: Английский
Citations
0Structures, Journal Year: 2025, Volume and Issue: 77, P. 109096 - 109096
Published: May 3, 2025
Language: Английский
Citations
0Engineering Structures, Journal Year: 2024, Volume and Issue: 322, P. 119193 - 119193
Published: Oct. 30, 2024
Language: Английский
Citations
3Multiscale and Multidisciplinary Modeling Experiments and Design, Journal Year: 2024, Volume and Issue: 8(1)
Published: Dec. 18, 2024
Language: Английский
Citations
3Structures, Journal Year: 2024, Volume and Issue: 71, P. 107999 - 107999
Published: Dec. 20, 2024
Language: Английский
Citations
3Alexandria Engineering Journal, Journal Year: 2024, Volume and Issue: 108, P. 1011 - 1031
Published: Sept. 30, 2024
Language: Английский
Citations
1