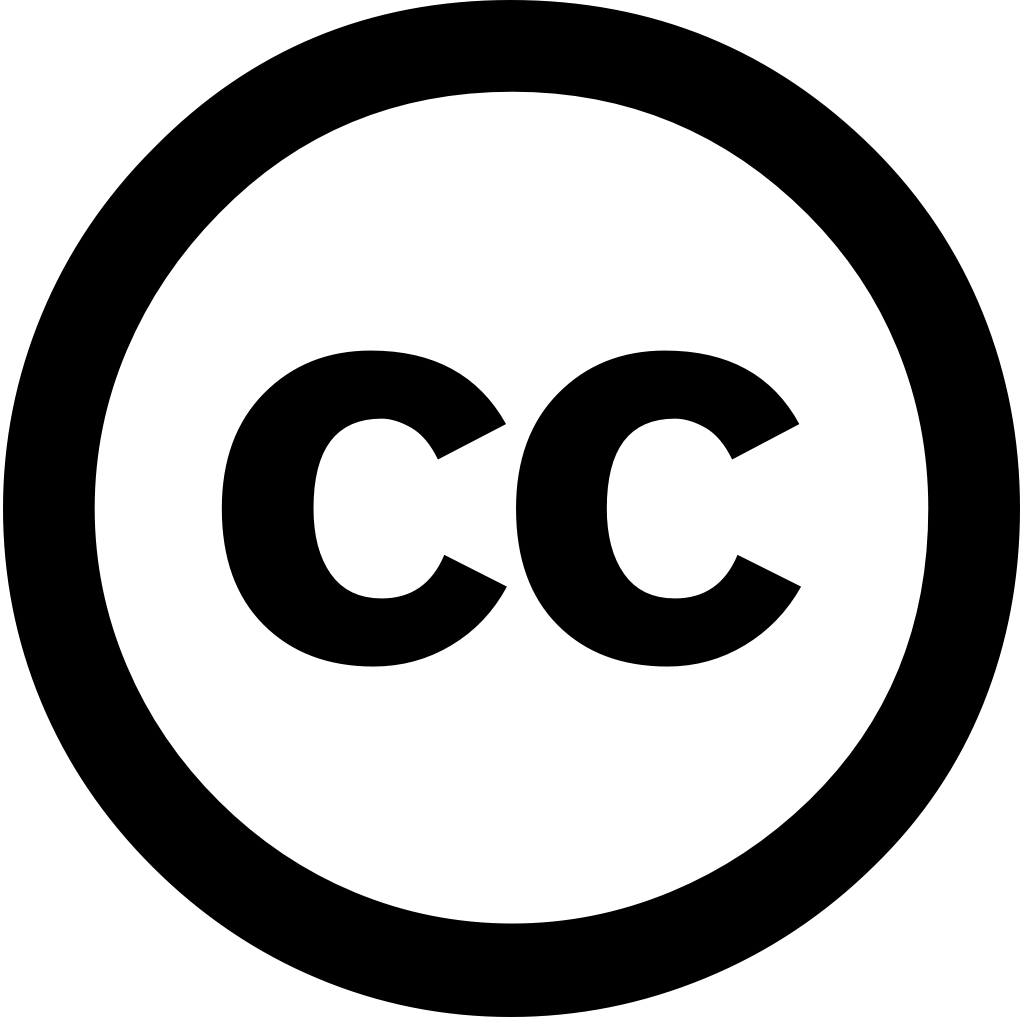
Geoenvironmental Disasters, Journal Year: 2024, Volume and Issue: 11(1)
Published: Sept. 5, 2024
Language: Английский
Geoenvironmental Disasters, Journal Year: 2024, Volume and Issue: 11(1)
Published: Sept. 5, 2024
Language: Английский
Transportation Infrastructure Geotechnology, Journal Year: 2025, Volume and Issue: 12(1)
Published: Jan. 1, 2025
Language: Английский
Citations
0Natural Hazards, Journal Year: 2025, Volume and Issue: unknown
Published: March 13, 2025
Language: Английский
Citations
0Scientific Reports, Journal Year: 2025, Volume and Issue: 15(1)
Published: April 1, 2025
Developing accurate predictive models for pile bearing capacity on rock is crucial optimizing foundation design and ensuring structural stability. This research presents an advanced data-driven framework that integrates multiple machine learning algorithms to predict the of piles based geotechnical in-situ test parameters. A comprehensive dataset comprising key influencing factors such as dimensions, geological characteristics, penetration resistance was utilized train validate various models, including Kstar, M5Rules, ElasticNet, XNV, Decision Trees. The Taylor diagram statistical evaluations demonstrated superiority proposed in capturing complex nonlinear relationships, with high correlation coefficients low root mean square errors indicating robust capabilities. Sensitivity analyses using Hoffman Gardener's approach SHAP values identified most influential parameters, revealing resistance, embedment depth, conditions significantly impact capacity. findings underscore effectiveness engineering applications, offering a reliable efficient alternative traditional empirical analytical methods. developed provides engineers practitioners powerful tool improving accuracy, reducing uncertainties, construction practices. Future should focus expanding diverse exploring hybrid modeling techniques enhance prediction accuracy further.
Language: Английский
Citations
0Engineering Analysis with Boundary Elements, Journal Year: 2025, Volume and Issue: 176, P. 106264 - 106264
Published: April 12, 2025
Citations
0Geomatics Natural Hazards and Risk, Journal Year: 2025, Volume and Issue: 16(1)
Published: April 15, 2025
Language: Английский
Citations
0Geotechnical and Geological Engineering, Journal Year: 2025, Volume and Issue: 43(2)
Published: Jan. 11, 2025
Language: Английский
Citations
0Indian geotechnical journal, Journal Year: 2025, Volume and Issue: unknown
Published: Feb. 7, 2025
Language: Английский
Citations
0Bulletin of Engineering Geology and the Environment, Journal Year: 2025, Volume and Issue: 84(4)
Published: March 6, 2025
Language: Английский
Citations
0Artificial Intelligence Review, Journal Year: 2025, Volume and Issue: 58(6)
Published: March 17, 2025
Language: Английский
Citations
0Transportation Infrastructure Geotechnology, Journal Year: 2025, Volume and Issue: 12(4)
Published: March 18, 2025
Language: Английский
Citations
0