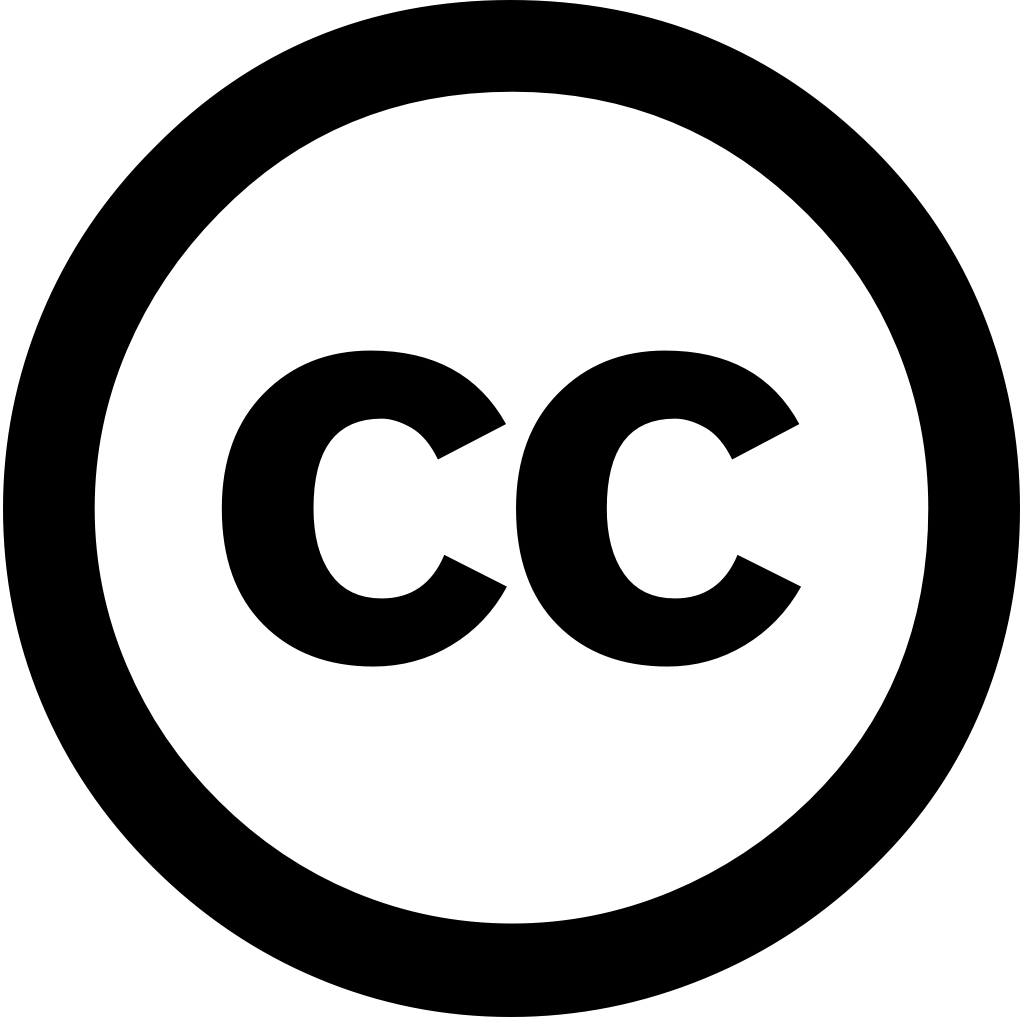
Research Square (Research Square), Journal Year: 2024, Volume and Issue: unknown
Published: Nov. 21, 2024
Language: Английский
Research Square (Research Square), Journal Year: 2024, Volume and Issue: unknown
Published: Nov. 21, 2024
Language: Английский
Environmental Science and Pollution Research, Journal Year: 2025, Volume and Issue: unknown
Published: Feb. 24, 2025
Language: Английский
Citations
4Journal of Hydrology, Journal Year: 2024, Volume and Issue: 632, P. 130907 - 130907
Published: Feb. 16, 2024
Language: Английский
Citations
16Engineered Science, Journal Year: 2024, Volume and Issue: unknown
Published: Jan. 1, 2024
Sheet piles are essential for maintaining the stability and retention of soil in various applications, including railway highway embankments, offshore structures, post-excavation sites, slope stabilization projects.The required depth sheet is contingent upon factors such as characteristics, groundwater conditions, employed construction method.This study focused on predicting embedment cantilever pile walls cohesive with a cohesionless backfill.Artificial intelligence (AI) techniques, specifically deep neural networks (DNNs), recurrent (RNNs), long short-term memory (LSTM) networks, bidirectional (Bi-LSTM) applied this purpose.Performance evaluation conducted through rank analysis, performance parameter determination, comparison actual versus predicted curves, accompanied by an error plot.A triangle diagram introduced graphical representation to assess different datasets or models.External validation was evaluate generalizability
Language: Английский
Citations
6PLoS ONE, Journal Year: 2025, Volume and Issue: 20(2), P. e0317148 - e0317148
Published: Feb. 20, 2025
Cancer, the second-leading cause of mortality, kills 16% people worldwide. Unhealthy lifestyles, smoking, alcohol abuse, obesity, and a lack exercise have been linked to cancer incidence mortality. However, it is hard. Cancer lifestyle correlation analysis mortality prediction in next several years are used guide people's healthy lives target medical financial resources. Two key research areas this paper Data preprocessing sample expansion design Using experimental comparison, study chooses best cubic spline interpolation technology on original data from 32 entry points 420 converts annual into monthly solve problem insufficient prediction. Factor possible because sources indicate changing factors. TSA-LSTM Two-stage attention popular tool with advanced visualization functions, Tableau, simplifies paper's study. Tableau's testing findings cannot analyze predict time series data. LSTM utilized by optimization model. By commencing input feature attention, model technique guarantees that encoder converges subset sequence features during output features. As result, model's natural learning trend quality enhanced. The second step, performance maintains We can choose network improve forecasts based real-time performance. Validating source factor using Most cancers overlapping risk factors, excessive drinking, exercise, obesity breast, colorectal, colon cancer. A poor directly promotes lung, laryngeal, oral cancers, according visual tests. expected climb 18-21% between 2020 2025, 2021. Long-term projection accuracy 98.96 percent, smoking may be main causes.
Language: Английский
Citations
0Stochastic Environmental Research and Risk Assessment, Journal Year: 2025, Volume and Issue: unknown
Published: Feb. 26, 2025
Language: Английский
Citations
0Journal of Geoscience and Environment Protection, Journal Year: 2025, Volume and Issue: 13(03), P. 347 - 363
Published: Jan. 1, 2025
Language: Английский
Citations
0Journal of Hydrology, Journal Year: 2025, Volume and Issue: unknown, P. 133424 - 133424
Published: April 1, 2025
Language: Английский
Citations
0Groundwater for Sustainable Development, Journal Year: 2024, Volume and Issue: 27, P. 101324 - 101324
Published: Aug. 27, 2024
Language: Английский
Citations
2Partial Differential Equations in Applied Mathematics, Journal Year: 2024, Volume and Issue: 11, P. 100861 - 100861
Published: Aug. 10, 2024
The current study modeled groundwater pollution through the utilization of advection–diffusion equation - a versatile differential that is capable modeling variety real-life processes. Indeed, various methods solutions were then proposed to examine governing model after being transformed, starting with Lie's symmetry, semi-analytical, and numerical methods, including explicit implicit finite difference method element method. Further, demonstrated on some test models; featuring forced unforced scenarios forcing function. Analytically, symmetry failed unswervingly reveal required solution problem; however, imposition certain restrictions, generalized closed-form for was acquired. This fact indeed triggered quest deployment more methods. Thus, semi-analytically, adopted decomposition swiftly gave resultant solutions. Numerically, efficiency sought assessed using L2−norm CPU time, upon which schemes found win race. All-in-all, beseeched semi-analytical highly recommended such investigation; at same time advocating effectiveness advection–diffusion-related equations.
Language: Английский
Citations
1Sensors, Journal Year: 2024, Volume and Issue: 24(23), P. 7508 - 7508
Published: Nov. 25, 2024
The precise detection of effluent biological oxygen demand (BOD) is crucial for the stable operation wastewater treatment plants (WWTPs). However, existing methods struggle to meet evolving drainage standards and management requirements. To address this issue, paper proposed a multivariable probability density-based auto-reconstruction bidirectional long short-term memory (MPDAR-Bi-LSTM) soft sensor predicting BOD, enhancing prediction accuracy efficiency. Firstly, selection appropriate auxiliary variables soft-sensor modeling determined through calculation k-nearest-neighbor mutual information (KNN-MI) values between global process BOD. Subsequently, considering existence strong interactions among different reaction tanks, Bi-LSTM neural network model constructed with historical data. Then, multivariate (MPDAR) strategy developed adaptive updating model, thereby its robustness. Finally, effectiveness demonstrated experiments using dataset from Benchmark Simulation Model No.1 (BSM1). experimental results indicate that not only outperforms some traditional models in terms performance but also excels avoiding ineffective reconstructions scenarios involving complex dynamic conditions.
Language: Английский
Citations
1