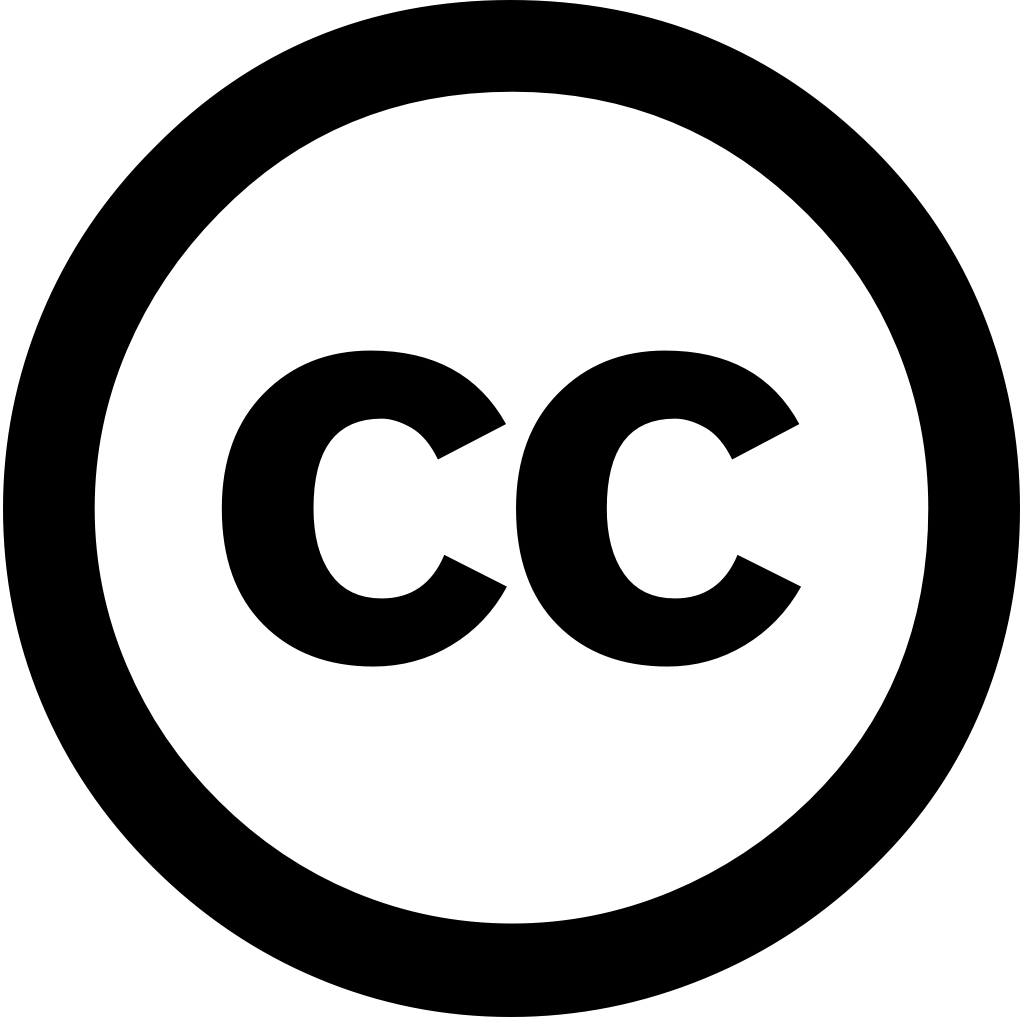
Applied Sciences, Journal Year: 2025, Volume and Issue: 15(9), P. 4905 - 4905
Published: April 28, 2025
Assessing the visual quality of rural landscapes is pivotal for quantifying ecological services and preserving cultural heritage; however, conventional indicators neglect emotional cognitive dimensions. To address this gap, present study proposes a novel assessment method that integrates multimodal sentiment classification models to strengthen sustainability metrics. Four landscape types were selected from three representative villages in Dalian City, China, physiological signals (EEG, EOG) subjective evaluations (Beauty Assessment SAM Scales) students teachers recorded. Binary, ternary, five-category emotion then developed. Results indicate binary ternary achieve superior accuracy valence arousal, whereas model performs least effectively. Furthermore, an ensemble learning approach outperforms individual classifiers both tasks, yielding 16.54% increase mean accuracy. Integrating objective data further enhances by 7.7% compared existing studies, confirming value multi-source features. These findings demonstrate computing framework can serve as robust quantitative tool evaluating promoting their sustainable development.
Language: Английский