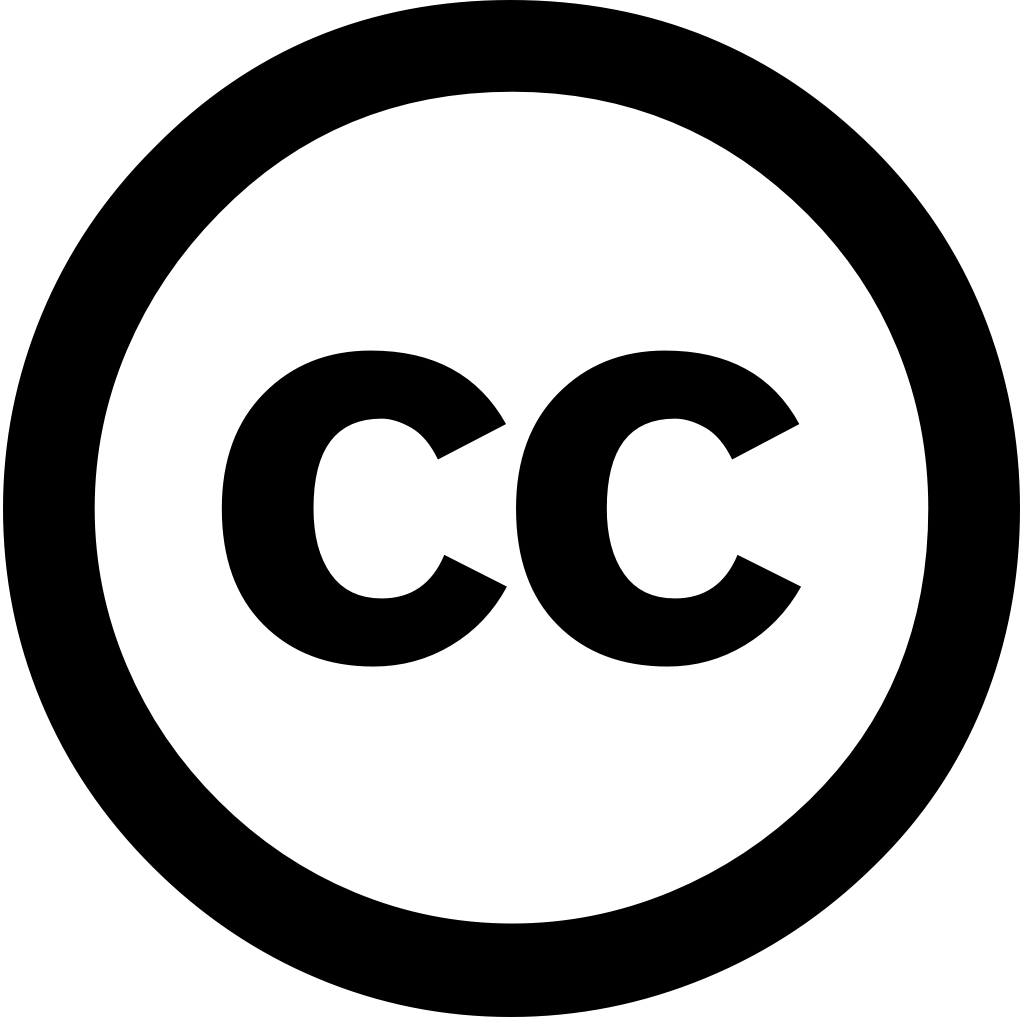
Bulletin du Cancer, Journal Year: 2024, Volume and Issue: unknown
Published: Dec. 1, 2024
Language: Английский
Bulletin du Cancer, Journal Year: 2024, Volume and Issue: unknown
Published: Dec. 1, 2024
Language: Английский
Journal of Cancer Education, Journal Year: 2025, Volume and Issue: unknown
Published: Feb. 18, 2025
Abstract The rapid integration of AI-driven chatbots into oncology education represents both a transformative opportunity and critical challenge. These systems, powered by advanced language models, can deliver personalized, real-time cancer information to patients, caregivers, clinicians, bridging gaps in access availability. However, their ability convincingly mimic human-like conversation raises pressing concerns regarding misinformation, trust, overall effectiveness digital health communication. This review examines the dual-edged role AI chatbots, exploring capacity support patient alleviate clinical burdens, while highlighting risks lack or inadequate algorithmic opacity (i.e., inability see data reasoning used make decision, which hinders appropriate future action), false information, ethical dilemmas posed human-seeming entities. Strategies mitigate these include robust oversight, transparent development, alignment with evidence-based protocols. Ultimately, responsible deployment requires commitment safeguarding core values practice, human-centered care.
Language: Английский
Citations
0Digital Health, Journal Year: 2025, Volume and Issue: 11
Published: March 1, 2025
Objective To conduct a bibliometric analysis using VOSviewer and Citespace to explore the current applications, trends, future directions of artificial intelligence (AI) in obstructive sleep apnea (OSA). Methods On 13 September 2024, computer search was conducted on Web Science Core Collection dataset published between 1 January 2011, 30 August identify literature related application AI OSA. Visualization performed countries, institutions, journal sources, authors, co-cited citations, keywords Vosviewer Citespace, descriptive tables were created by Microsoft Excel 2021 software. Results A total 867 articles included this study. The number publications low stable from 2011 2016, with significant increase after 2017. China had highest publications. Alvarez, Daniel, Hornero, Roberto two most prolific authors. Universidad de Valladolid IEEE Journal Biomedical Health Informatics productive institution journal, respectively. top three authors terms co-citation frequency are Hassan, Ar, Young, T, Vicini, C. “Estimation global prevalence burden apnoea: literature-based analysis” cited frequently. Keywords such as “OSA,” “machine learning,” “Electrocardiography,” “deep learning” dominant. Conclusion AI's OSA research is expanding. This study indicates that AI, particularly deep learning, will continue be key area, focusing diagnosis, identification, personalized treatment, prognosis assessment, telemedicine, management. Future efforts should enhance international cooperation interdisciplinary communication maximize potential advancing research, comprehensively empowering health, bringing more precise, convenient, medical services patients ushering new era health.
Language: Английский
Citations
0Advances in Therapy, Journal Year: 2025, Volume and Issue: unknown
Published: April 29, 2025
Language: Английский
Citations
0Frontiers in Education, Journal Year: 2025, Volume and Issue: 10
Published: May 1, 2025
Background In the recent generative artificial intelligence (genAI) era, health sciences students (HSSs) are expected to face challenges regarding their future roles in healthcare. This multinational cross-sectional study aimed confirm validity of novel FAME scale examining themes Fear, Anxiety, Mistrust, and Ethical issues about genAI. The also explored extent apprehension among HSSs genAI integration into careers. Methods was based on a self-administered online questionnaire distributed using convenience sampling. survey instrument scale, while toward assessed through modified State-Trait Anxiety Inventory (STAI). Exploratory confirmatory factor analyses were used construct scale. Results final sample comprised 587 mostly from Jordan (31.3%), Egypt (17.9%), Iraq (17.2%), Kuwait (14.7%), Saudi Arabia (13.5%). Participants included studying medicine (35.8%), pharmacy (34.2%), nursing (10.7%), dentistry (9.5%), medical laboratory (6.3%), rehabilitation (3.4%). Factor analysis confirmed reliability Of constructs, Mistrust scored highest, followed by Ethics. participants showed generally neutral genAI, with mean score 9.23 ± 3.60. multivariate analysis, significant variations observed previous ChatGPT use, faculty, nationality, expressing highest level apprehension, Kuwaiti lowest. Previous use correlated lower levels. higher agreement Ethics constructs statistically associations apprehension. Conclusion revealed notable Arab HSSs, which highlights need for educational curricula that blend technological proficiency ethical awareness. Educational strategies tailored discipline culture needed ensure job security competitiveness an AI-driven future.
Language: Английский
Citations
0npj Precision Oncology, Journal Year: 2025, Volume and Issue: 9(1)
Published: Jan. 27, 2025
Publicly available trial matching tools can improve the access to therapeutic innovations, but errors may expose over-solicitation and disappointment. We performed a pragmatic non-interventional prospective evaluation on sequential patients at Molecular Tumor Board of Centre Leon Berard. During 10 weeks in 2024, we analysed 157 with four clinical from 19 screened: Klineo, ScreenAct, Trialing DigitalECMT. Each patient had 2.19 trials proposed average, 38% no suggested. The mean performances were precision = 0.33, recall 0.32, AP@3 0.45, NDCG@3 0.34. Using all increase 26% options. most frequent error concerned type gene variants required by selection criteria. showed that using Large Language Model patients' molecular reports could performance up 5%. recommend experts supervise results advocate for improved technologies.
Language: Английский
Citations
0Journal of Clinical Medicine, Journal Year: 2025, Volume and Issue: 14(10), P. 3285 - 3285
Published: May 8, 2025
Recently, there has been tremendous interest on the use of large language models (LLMs) in radiology. LLMs have employed for various applications cancer imaging, including improving reporting speed and accuracy via generation standardized reports, automating classification staging abnormal findings incorporating appropriate guidelines, calculating individualized risk scores. Another is their ability to improve patient comprehension imaging reports with simplification medical terms possible translations multiple languages. Additional future include multidisciplinary tumor board standardizations, aiding management, preventing predicting adverse events (contrast allergies, MRI contraindications) research. However, limitations such as hallucinations variable performances could present obstacles widespread clinical implementation. Herein, we a review current well pitfalls limitations.
Language: Английский
Citations
0The Lancet Regional Health - Europe, Journal Year: 2024, Volume and Issue: 46, P. 101081 - 101081
Published: Sept. 20, 2024
Language: Английский
Citations
1Frontiers in Surgery, Journal Year: 2024, Volume and Issue: 11
Published: Oct. 22, 2024
Introduction The prognostic landscape of stage III Lung Adenosquamous Carcinoma (ASC) following primary tumor resection remains underexplored. A thoughtfully developed model has the potential to guide clinicians in patient counseling and formulation effective therapeutic strategies. Methods Utilizing data from Surveillance, Epidemiology, End Results database spanning 2000 2018, this study identified independent factors influencing Overall Survival (OS) ASC using Boruta analysis. Employing Gradient Boosting, Random Forest, Neural Network algorithms, predictive models were constructed. Model performance was assessed through key metrics, including Area Under Receiver Operating Characteristic Curve (AUC), calibration plot, Brier score, Decision Analysis (DCA). Among 241 eligible patients, seven clinical parameters—age, sex, size, N stage, site, chemotherapy, systemic therapy—were as significant predictors OS. Advanced age, male gender, larger absence lack therapy associated with poorer survival. Forest outperformed others, achieving 3- 5-year AUCs 0.80/0.79 (training) 0.74/0.65 (validation). It also demonstrated better calibration, lower scores (training: 0.189/0.171; validation: 0.207/0.199), more favorable DCA. SHAP values enhanced interpretability by highlighting impact each parameter on survival predictions. To facilitate application, deployed a web-based server for accessible assessments. Conclusions This presents robust machine learning tool that assist healthcare practitioners personalized decision-making treatment optimization patients resection.
Language: Английский
Citations
0Bulletin du Cancer, Journal Year: 2024, Volume and Issue: unknown
Published: Dec. 1, 2024
Language: Английский
Citations
0