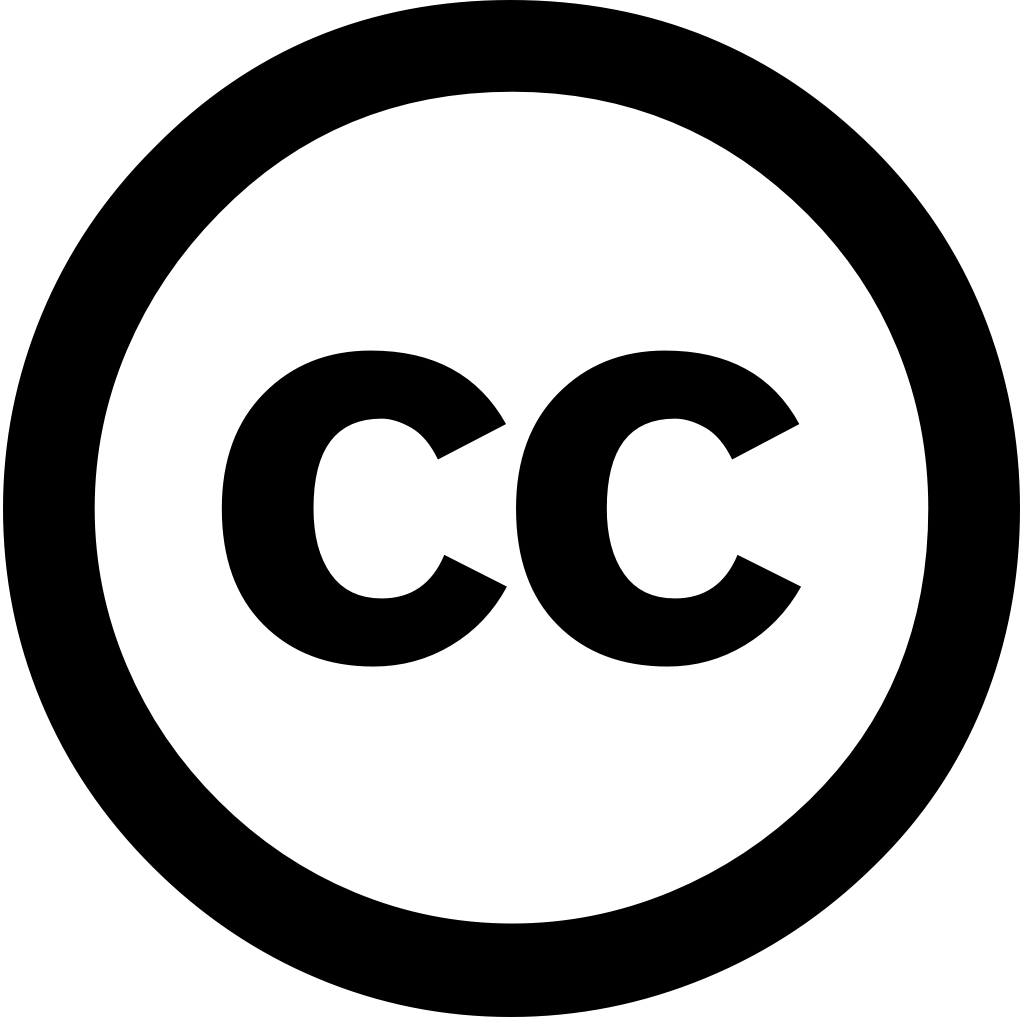
Scientific Reports, Journal Year: 2024, Volume and Issue: 14(1)
Published: Dec. 30, 2024
Accurate estimation of the soil resilient modulus (M
Language: Английский
Scientific Reports, Journal Year: 2024, Volume and Issue: 14(1)
Published: Dec. 30, 2024
Accurate estimation of the soil resilient modulus (M
Language: Английский
International Journal of Hydrogen Energy, Journal Year: 2025, Volume and Issue: 101, P. 1421 - 1438
Published: Jan. 8, 2025
Language: Английский
Citations
3Construction and Building Materials, Journal Year: 2025, Volume and Issue: 460, P. 139811 - 139811
Published: Jan. 1, 2025
Language: Английский
Citations
2Sustainability, Journal Year: 2025, Volume and Issue: 17(5), P. 2048 - 2048
Published: Feb. 27, 2025
Natural gas, as a sustainable and cleaner energy source, still holds crucial position in the transition stage. In shale gas exploration, total organic carbon (TOC) content plays role, with log data proving beneficial predicting reservoirs. However, complex coal-bearing layers like marine–continental transitional Shanxi Formation, traditional prediction methods exhibit significant errors. Therefore, this study proposes an advanced, cost- time-saving deep learning approach to predict TOC shale. Five well records from area were used evaluate five machine models: K-Nearest Neighbors (KNNs), Random Forest (RF), Gradient Boosting Decision Tree (GBDT), Extreme (XGB), Deep Neural Network (DNN). The predictive results compared conventional for accurate predictions. Through K-fold cross-validation, ML models showed superior accuracy over models, DNN model displaying lowest root mean square error (RMSE) absolute (MAE). To enhance accuracy, δR was integrated new parameter into models. Comparative analysis revealed that improved DNN-R reduced MAE RMSE by 57.1% 70.6%, respectively, on training set, 59.5% 72.5%, test original model. Williams plot permutation importance confirmed reliability effectiveness of enhanced indicate potential technology valuable tool parameters, especially reservoirs lacking sufficient core samples relying solely basic well-logging data, signifying its effective assessment development.
Language: Английский
Citations
1Scientific Reports, Journal Year: 2024, Volume and Issue: 14(1)
Published: Sept. 6, 2024
Language: Английский
Citations
4Journal of African Earth Sciences, Journal Year: 2025, Volume and Issue: unknown, P. 105631 - 105631
Published: March 1, 2025
Language: Английский
Citations
0Minerals, Journal Year: 2025, Volume and Issue: 15(3), P. 240 - 240
Published: Feb. 26, 2025
The lithofacies of a reservoir contain key information such as rock lithology, sedimentary structures, and mineral composition. Accurate prediction shale is crucial for identifying sweet spots oil gas development. However, obtaining through core sampling during drilling challenging, the accuracy traditional logging curve intersection methods insufficient. To efficiently accurately predict lithofacies, this study proposes hybrid model called Stacking, which combines four classifiers: Random Forest, HistGradient Boosting, Extreme Gradient Categorical Boosting. employs Grid Search Method to automatically search optimal hyperparameters, using classifiers base learners. predictions from these learners are then used new features, Logistic Regression serves final meta-classifier prediction. A total 3323 data points were collected six wells train test model, with performance evaluated on two blind that not involved in training process. results indicate stacking predicts achieving an Accuracy, Recall, Precision, F1 Score 0.9587, 0.959, respectively, set. This achievement provides technical support evaluation spot exploration.
Language: Английский
Citations
0Scientific Reports, Journal Year: 2025, Volume and Issue: 15(1)
Published: March 27, 2025
An accurate assessment of shale gas resources is highly important for the sustainable development these energy resources. Total organic carbon (TOC) analysis thus becomes fundamental understanding distribution and quality hydrocarbon source rocks within a reservoir. The elevation TOC often associated with presence rocks, indicating potential oil production. performed using laboratory methods, which can be time-consuming costly. Data-driven models have been successfully applied to model relationship between other constituents predict content. However, methods depend on extensive parameter adjustments that must carefully conducted in different sedimentary environments. In this context, Automated Machine Learning (AutoML) an alternative accurately predicting TOCs, saving fine-tuning steps development. This study aims develop AutoML strategy estimating well log data. procedure automatically preprocesses search best method parameters, reducing execution time. Among evaluated, Extremely Randomized Trees (XT) (R = 0.8632, MSE 0.1806) test set. proposed provides powerful data-driven method, allows real-world use assist data subsequent decision-making.
Language: Английский
Citations
0Marine and Petroleum Geology, Journal Year: 2025, Volume and Issue: 179, P. 107436 - 107436
Published: April 29, 2025
Language: Английский
Citations
0Geophysics, Journal Year: 2024, Volume and Issue: 89(5), P. MR265 - MR280
Published: May 30, 2024
Tight sandstone reservoirs exhibit strong vertical heterogeneity and complex pore structures, challenging conventional permeability evaluation methods based on well-logging data. Although rising machine-learning (ML) techniques have demonstrated excellent accuracy for industrial applications, the physics rationality within such a powerful “black box” remain less clear. Hence, reliable prediction would benefit from an interpretable ML-based workflow that could reveal controlling factors. To compare models examine underlying features, 16 different ML submodels are tested after data preprocessing, feature selection, hyperparameter optimization. By comparing fitting tuning time, light gradient boosting machine optimized by whale optimization algorithm, referred to as LGB-WOA, is determined be optimal model with best relatively short time. A field application demonstrates even in highly heterogeneous reservoir sections, LGB-WOA outperformed petrophysical being most consistent directly measured core samples ([Formula: see text]). The Shapley additive explanation values then used interpret predictions of our model. As expected, porosity curve exhibits highest importance among all input significantly contributing predictions. Conversely, wellbore diameter compensated neutron log contribute least need not subsequent improvements. These experiments provide method accurately assessing broader understanding characterization, paving way establishing more models.
Language: Английский
Citations
2Scientific Reports, Journal Year: 2024, Volume and Issue: 14(1)
Published: Nov. 28, 2024
Deep and ultra-deep carbonate reservoirs in China, which account for 34% of the country's oil gas reserves, pose significant challenges porosity prediction due to their complex geological features, including extensive burial depth, weak seismic signals, high heterogeneity. To address these challenges, this study develops an advanced deep learning approach specifically designed ultra-deep, fault-controlled, fractured-vuggy Tarim Basin. The utilizes a three-dimensional dataset applies Principal Component Analysis (PCA) select five key features from eight attributes. Additionally, phase-controlled constraints are incorporated into model. Using technology, model has been constructed. Validation using blind wells Shunbei oilfield shows that achieves 76% reduction Mean Square Error (MSE) compared traditional impedance inversion techniques, highlighting its predictive accuracy. Through SHapley Additive exPlanations (SHAP) analysis, attributes LAMBDA_AAGFIL PHASE_ANT identified as most influential, importance representing karst cave fracture structures within reservoir. These findings underscore innovation substantial improvement proposed method over conventional offering robust high-precision reservoirs.
Language: Английский
Citations
1