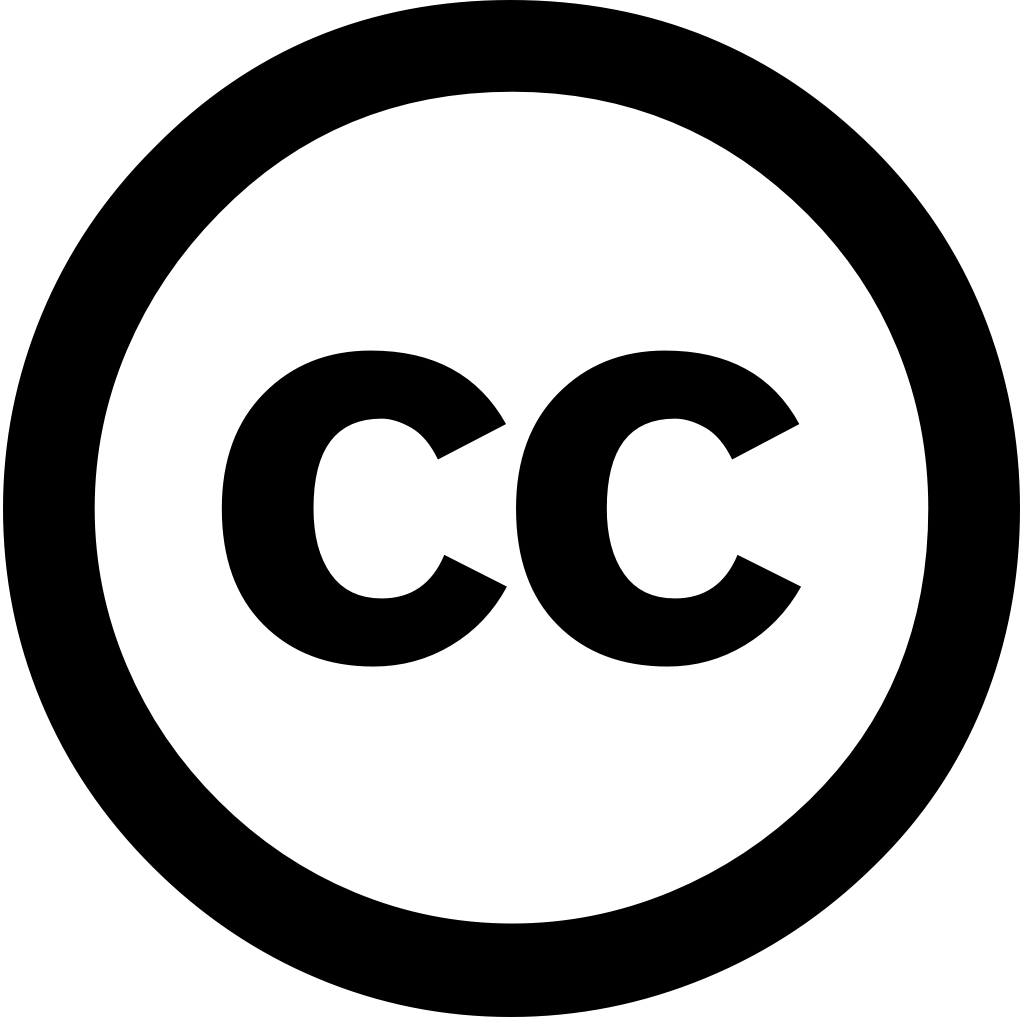
Cleaner Waste Systems, Journal Year: 2025, Volume and Issue: unknown, P. 100296 - 100296
Published: April 1, 2025
Language: Английский
Cleaner Waste Systems, Journal Year: 2025, Volume and Issue: unknown, P. 100296 - 100296
Published: April 1, 2025
Language: Английский
Materials Today Communications, Journal Year: 2025, Volume and Issue: unknown, P. 112017 - 112017
Published: Feb. 1, 2025
Language: Английский
Citations
1Case Studies in Construction Materials, Journal Year: 2024, Volume and Issue: 21, P. e03869 - e03869
Published: Oct. 16, 2024
Language: Английский
Citations
7Sustainability, Journal Year: 2024, Volume and Issue: 16(15), P. 6644 - 6644
Published: Aug. 3, 2024
This study investigates the application of artificial intelligence (AI) to predict compressive strength self-compacting concrete (SCC) through ultrasonic measurements, thereby contributing sustainable construction practices. By leveraging advancements in computational techniques, specifically neural networks (ANNs), we developed highly accurate predictive models forecast SCC based on pulse velocity (UPV) measurements. Our findings demonstrate a clear correlation between higher UPV readings and improved quality, despite general trend decreased with increased air-entraining admixture (AEA) concentrations. The ANN show exceptional effectiveness predicting strength, coefficient (R2) 0.99 predicted actual values, providing robust tool for optimizing mix designs ensuring quality control. AI-driven approach enhances sustainability by improving material efficiency significantly reducing need traditional destructive testing methods, thus offering rapid, reliable, non-destructive alternative assessing properties.
Language: Английский
Citations
5Progress in Additive Manufacturing, Journal Year: 2025, Volume and Issue: unknown
Published: Feb. 21, 2025
Abstract The absence of formwork in 3D-printed concrete, unlike conventional mold-cast introduces greater variability curing conditions, posing significant challenges accurately estimating the early-age mechanical strength. Therefore, common non-destructive techniques such as maturity method fail to deliver a generalized predictive model for strength structures. In this study, multiple machine learning (ML) algorithms, including linear regression (LR), support vector (SVR), and artificial neural network (ANN), were developed estimate flexural beams under varying utilizing data collected from embedded sensors. Six input variables employed ML models, relative permittivity, internal temperature, method. For development, 144 points an extensive experimental statistical metrics evaluate proposed models. ANN outperformed other models predicting strength, achieving coefficient determination 95.1%. Furthermore, variable analysis highlighted most influential factor affecting beams.
Language: Английский
Citations
0Cleaner Waste Systems, Journal Year: 2025, Volume and Issue: unknown, P. 100296 - 100296
Published: April 1, 2025
Language: Английский
Citations
0