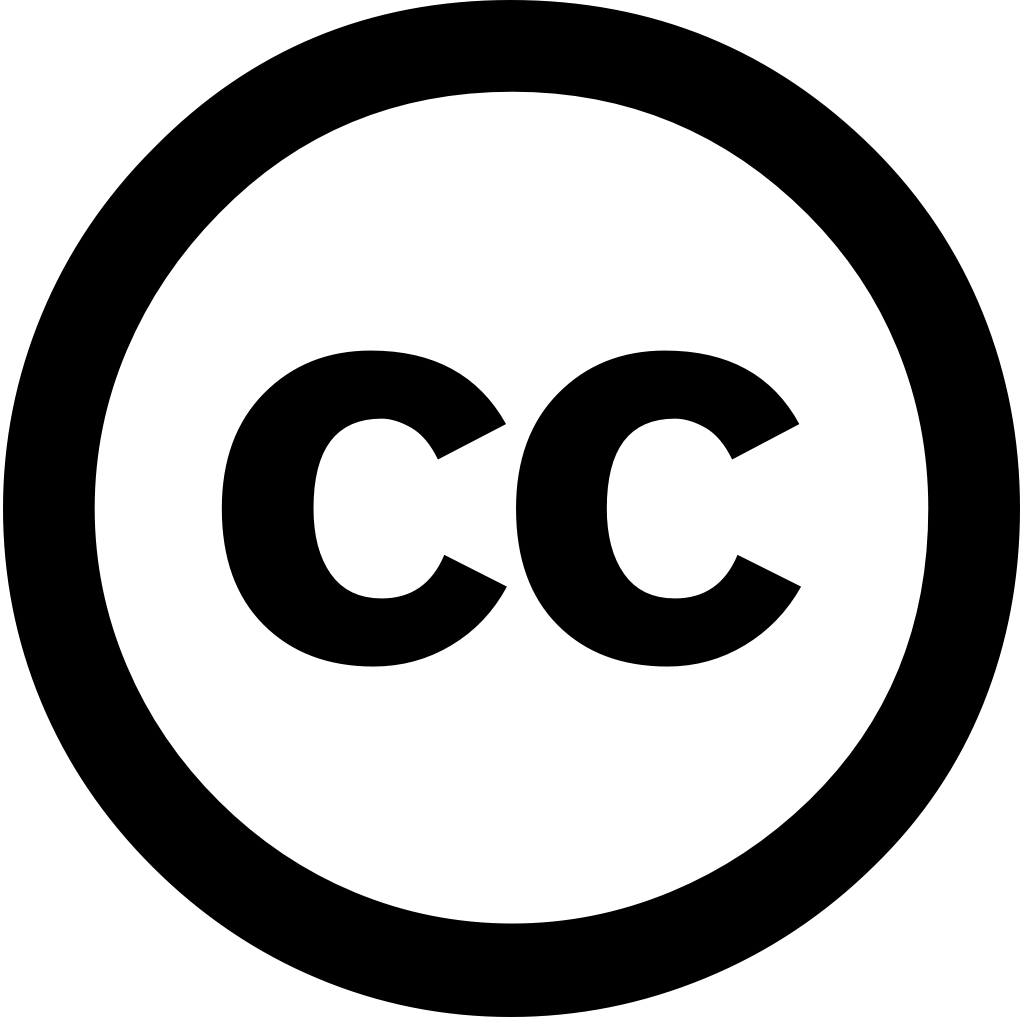
IEEE Journal of Selected Topics in Applied Earth Observations and Remote Sensing, Journal Year: 2024, Volume and Issue: 17, P. 10770 - 10780
Published: Jan. 1, 2024
Language: Английский
IEEE Journal of Selected Topics in Applied Earth Observations and Remote Sensing, Journal Year: 2024, Volume and Issue: 17, P. 10770 - 10780
Published: Jan. 1, 2024
Language: Английский
Artificial Intelligence Review, Journal Year: 2023, Volume and Issue: 56(S3), P. 2917 - 2970
Published: Oct. 4, 2023
Language: Английский
Citations
81Earth s Future, Journal Year: 2025, Volume and Issue: 13(1)
Published: Jan. 1, 2025
Abstract Effective wildfire prevention includes actions to deliberately target different causes. However, the cause of an increasing number wildfires is unknown, hindering targeted efforts. We developed a machine learning model ignition across western United States on basis physical, biological, social, and management attributes associated with wildfires. Trained from 1992 2020 12 known causes, overall accuracy our exceeded 70% when applied out‐of‐sample test data. Our more accurately separated ignited by natural versus human causes (93% accuracy), discriminated among 11 classes human‐ignited 55% accuracy. attributed greatest percentage 150,247 for which source was unknown equipment vehicle use (21%), lightning (20%), arson incendiarism (18%).
Language: Английский
Citations
4Applied System Innovation, Journal Year: 2023, Volume and Issue: 6(4), P. 61 - 61
Published: June 25, 2023
Automated classification of satellite images is a challenging task that enables the use remote sensing data for environmental modeling Earth’s landscapes. In this document, we implement GRASS GIS-based framework discriminating land cover types to identify changes in endorheic basins ephemeral salt lakes Chott Melrhir and Merouane, Algeria; employ embedded algorithms image processing. This study presents dataset nine Landsat 8–9 OLI/TIRS obtained from USGS 9-year period, 2014 2022. The were analyzed detect water levels experience temporal fluctuations; these are dry most time fed with during rainy periods. unsupervised was performed using GIS through several modules: ‘i.cluster’ used generate classes; ‘i.maxlik’ maximal likelihood discriminant analysis, auxiliary modules, such as ‘i.group’, ‘r.support’, ‘r.import’, etc., used. document includes technical descriptions scripts processing detailed comments on functionalities modules. results include identified variations within Algerian part Sahara over period (2014–2022), series multispectral classified GIS. main strengths high speed, accuracy, effectiveness programming codes monitoring. presented GitHub repository, which contains serves reference interpretation monitoring arid semi-arid areas Africa.
Language: Английский
Citations
14Remote Sensing, Journal Year: 2024, Volume and Issue: 16(5), P. 884 - 884
Published: March 2, 2024
Forest fires are caused by various climatic and anthropogenic factors. In Republic of Korea, forest occur frequently during spring when the humidity is low. During past decade, number fire incidents extent damaged area have increased. Satellite imagery can be applied to assess damage from these unpredictable fires. Despite increasing threat, there a lack comprehensive analysis effective strategies for addressing fires, particularly considering diverse topography Korea. Herein, we present an approach automated detection using Sentinel-2 images 14 areas affected in Korea 2019–2023. The performance deep learning (DL), machine learning, spectral index methods was analyzed, optimal model detecting derived. To evaluate independent models, two different burned exhibiting distinct characteristics were selected as test subjects. increase classification accuracy, tests conducted on combinations input channels DL. combination false-color RNG (B4, B8, B3) damage. Consequently, among DL HRNet achieved excellent results both regions with intersection over union scores 89.40 82.49, confirming that proposed method applicable Korean landscapes. Thus, suitable mitigation measures promptly designed based rapid areas.
Language: Английский
Citations
5Expert Systems with Applications, Journal Year: 2024, Volume and Issue: 259, P. 125321 - 125321
Published: Sept. 6, 2024
Language: Английский
Citations
4Remote Sensing Applications Society and Environment, Journal Year: 2025, Volume and Issue: unknown, P. 101513 - 101513
Published: Feb. 1, 2025
Language: Английский
Citations
0IEEE Journal of Selected Topics in Applied Earth Observations and Remote Sensing, Journal Year: 2024, Volume and Issue: 17, P. 7801 - 7824
Published: Jan. 1, 2024
Language: Английский
Citations
2International Journal of Applied Earth Observation and Geoinformation, Journal Year: 2024, Volume and Issue: 132, P. 104034 - 104034
Published: July 30, 2024
The fusion of remote sensing and artificial intelligence, particularly deep learning, offers substantial opportunities for developing innovative methods in rapid disaster mapping damage assessment. However, current models wildfire burnt area detection are mostly constrained handling imbalanced training samples with non-burnt areas oversampled, boundary a mix unburnt pixels, regions varying environmental contexts, leading to poor model generalizability. In response, this paper proposes novel U-Net based model, known as BiAU-Net, which incorporates attention mechanisms well-designed loss function, enabling the focus on improve accuracy efficiency, especially detecting edges small areas. Unlike traditional single-input image segmentation, proposed BiAU-Net considers temporal changes two inputs, pre- post-fire Sentinel-2 imagery, enhancing performance across diverse Five independent from different continents selected study cases, one all five testing, demonstrate generalizability model. We used Fire Disturbance Climate Change Initiative v5.1 product European Space Agency baseline evaluation. experiment results indicate that BiAU-Net: (1) significantly outperformed improvements 11.56% Overall Accuracy, 29.08% Precision, 7.06% Recall, 19.90% F1-score, 15.44% Balanced 29.90% Kappa Coefficient, 28.29% Matthews Correlation Coefficient (MCC); (2) largely surpassed its variants most areas; (3) demonstrated good testing continents; (4) achieved highest overall compared state-of-the-art models, evidenced by F1-score MCC values.
Language: Английский
Citations
2GEOMATICA, Journal Year: 2024, Volume and Issue: 76(1), P. 100008 - 100008
Published: July 1, 2024
Wildfires pose an increasing risk to expanding urban population centers, and critical habitats for plant animal species. Improving current wildland management strategies are vital mitigating loss of global biodiversity preventing the displacement residents. Accurate maps areas burned by wildfires is a primary source information required developing strategies. Advancements in underlying technologies mapping comes from three key areas: 1) remotely sensed data, 2) cloud geoprocessing platforms, 3) emerging image processing algorithms. Trends across these were explored this review, addition in-depth discussion comparison optimal usage scenarios. This review provides crucial insights researchers practitioners keen on exploring methods that hold potential improve wildfire area procedures.
Language: Английский
Citations
2Hydrological Sciences Journal, Journal Year: 2023, Volume and Issue: 69(1), P. 139 - 148
Published: Nov. 15, 2023
Addressing post-fire impacts largely depends on burn "severity." A singular severity classification that encompasses the holistic effects of fire all ecosystem processes does not currently exist. Lumping vegetation and soil into one metric, or using them interchangeably, can induce large inaccuracies uncertainties in intended response to forcing. Often, "severity" reflects vegetation, which be measured through remote sensing. Vegetation is likely more apropos for ecological research, whereas relevant hydrological analyses. This paper reviews different remotely sensed products (mis)used modeling, provides examples when may (not) match severity, summarizes potential synergistic future sensing with situ metrics. While focus this western United States, lessons principles apply universally.
Language: Английский
Citations
5