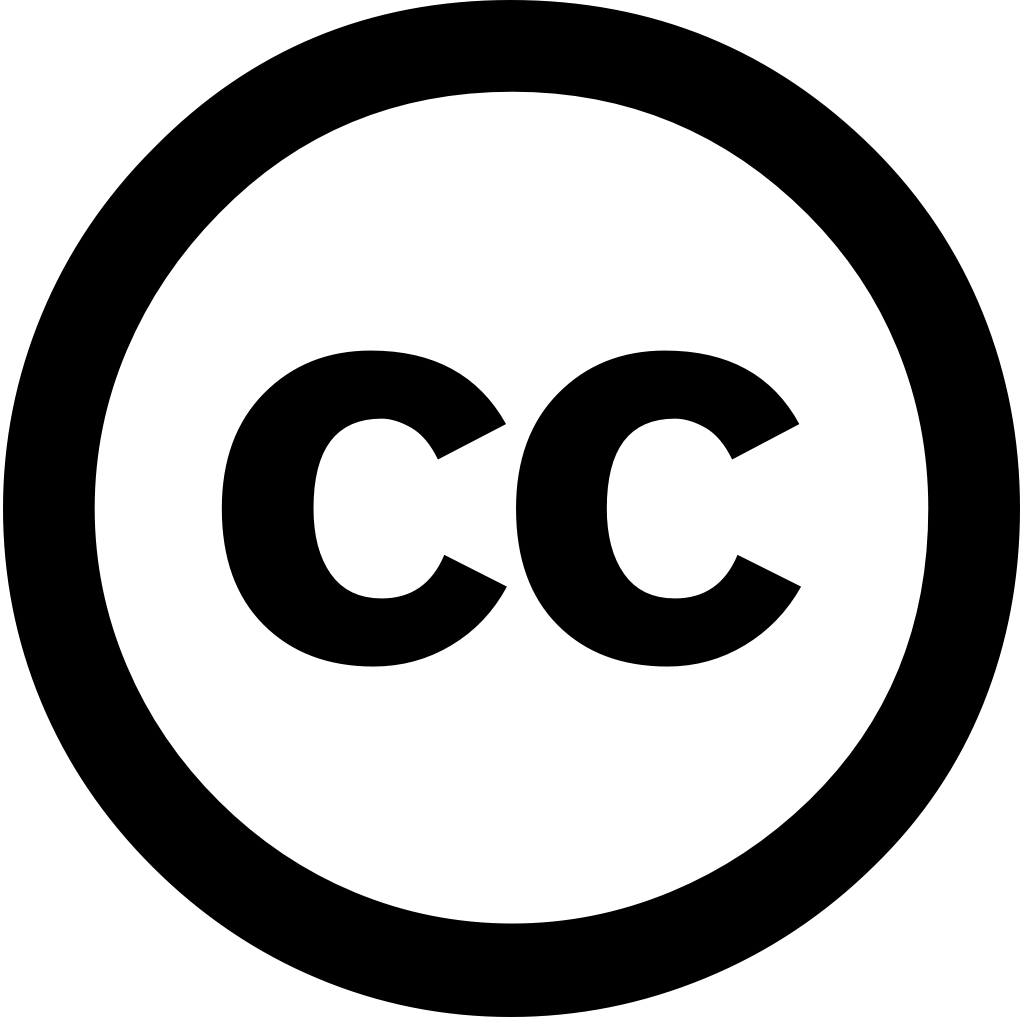
Applied Food Research, Journal Year: 2025, Volume and Issue: unknown, P. 100872 - 100872
Published: April 1, 2025
Language: Английский
Applied Food Research, Journal Year: 2025, Volume and Issue: unknown, P. 100872 - 100872
Published: April 1, 2025
Language: Английский
Applied Mathematics and Nonlinear Sciences, Journal Year: 2025, Volume and Issue: 10(1)
Published: Jan. 1, 2025
Abstract Traditional tobacco adulteration manual detection methods have problems such as complex operation and low precision, which cannot meet the increasingly refined needs of detection. In order to realize discrimination different varieties tobacco, this study combines hyperspectral imaging technology with deep learning design a method that can quickly accurately identify adulteration. Hyperspectral images were collected, region interest for collecting image data samples was selected means obtaining spectral leaves. Then original preprocessed using SNV method, followed by application PCA processing dimensionality reduction data. Finally, last 5 layers GoogLeNet removed 15 new added, ReLU activation function changed LeakyReLU in improve CNN model enhance its expressiveness. Data preprocessing, reduction, recognition based on improved convolutional neural network established. The experimental carried out. results show accuracy paper reaches 97.56% after processing. modeling time reduced 30% method. It shows scheme combining preprocessing effectively recognize And it also play an excellent performance problem origin identification, indicates combination machine provide powerful field identification adulterated has wide range prospects identification.
Language: Английский
Citations
0Journal of Trace Elements and Minerals, Journal Year: 2025, Volume and Issue: unknown, P. 100239 - 100239
Published: March 1, 2025
Language: Английский
Citations
0Applied Food Research, Journal Year: 2025, Volume and Issue: unknown, P. 100872 - 100872
Published: April 1, 2025
Language: Английский
Citations
0