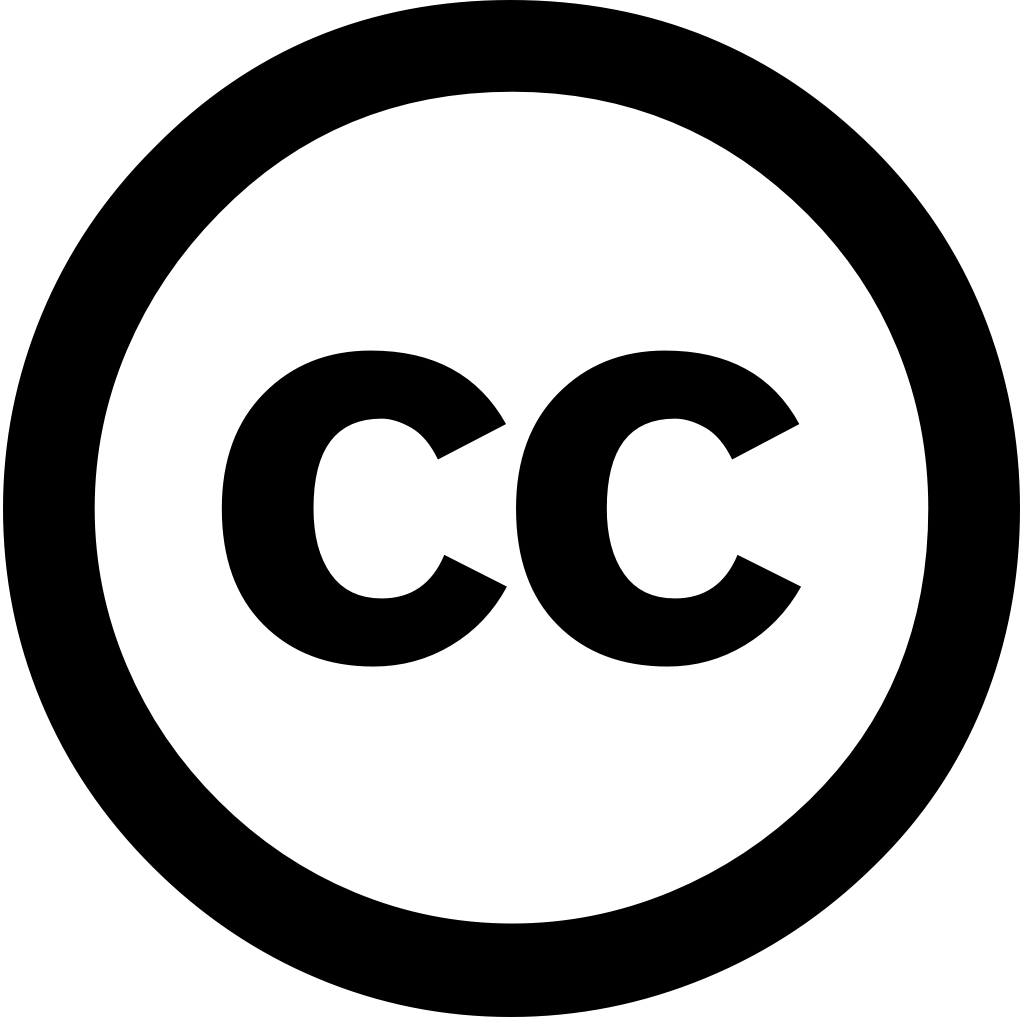
Ain Shams Engineering Journal, Journal Year: 2024, Volume and Issue: 16(1), P. 103209 - 103209
Published: Dec. 28, 2024
Language: Английский
Ain Shams Engineering Journal, Journal Year: 2024, Volume and Issue: 16(1), P. 103209 - 103209
Published: Dec. 28, 2024
Language: Английский
Scientific Reports, Journal Year: 2024, Volume and Issue: 14(1)
Published: Aug. 6, 2024
Accurately predicting the Modulus of Resilience (MR) subgrade soils, which exhibit non-linear stress–strain behaviors, is crucial for effective soil assessment. Traditional laboratory techniques determining MR are often costly and time-consuming. This study explores efficacy Genetic Programming (GEP), Multi-Expression (MEP), Artificial Neural Networks (ANN) in forecasting using 2813 data records while considering six key parameters. Several Statistical assessments were utilized to evaluate model accuracy. The results indicate that GEP consistently outperforms MEP ANN models, demonstrating lowest error metrics highest correlation indices (R2). During training, achieved an R2 value 0.996, surpassing (R2 = 0.97) 0.95) models. Sensitivity SHAP (SHapley Additive exPlanations) analysis also performed gain insights into input parameter significance. revealed confining stress (21.6%) dry density (26.89%) most influential parameters MR. corroborated these findings, highlighting critical impact on predictions. underscores reliability as a robust tool precise prediction applications, providing valuable performance significance across various machine-learning (ML) approaches.
Language: Английский
Citations
9Materials Today Communications, Journal Year: 2025, Volume and Issue: 43, P. 111627 - 111627
Published: Jan. 15, 2025
Language: Английский
Citations
1Construction and Building Materials, Journal Year: 2025, Volume and Issue: 467, P. 140376 - 140376
Published: Feb. 12, 2025
Language: Английский
Citations
0Materials Today Communications, Journal Year: 2025, Volume and Issue: unknown, P. 111882 - 111882
Published: Feb. 1, 2025
Language: Английский
Citations
0REVIEWS ON ADVANCED MATERIALS SCIENCE, Journal Year: 2025, Volume and Issue: 64(1)
Published: Jan. 1, 2025
Abstract The performance and durability of conventional concrete (CC) are significantly influenced by its weak tensile strength strain capacity (TSC). Thus, the intrusion fibers in cementitious matrix forms ductile engineered composites (ECCs) that can cater to this area CC. Moreover, ECCs have become a reasonable substitute for brittle plain due their increased flexibility, ductility, greater TSC. prediction ECC is crucial without need laborious experimental procedures. achieve this, machine learning approaches (MLAs), namely light gradient boosting (LGB) approach, extreme (XGB) artificial neural network (ANN), k -nearest neighbor (KNN), were developed. data gathered from literature comprise input parameters which fiber content, length, cement, diameter, water-to-binder ratio, fly ash (FA), age, sand, superplasticizer, TSC as output utilized. assessment models gauged with coefficient determination ( R 2 ), statistical measures, uncertainty analysis. In addition, an analysis feature importance carried out further refinement model. result demonstrates ANN XGB perform well train test sets > 0.96. Statistical measures show all give fewer errors higher , depict robust performance. Validation via K -fold confirms showing correlation determination. reveals FA major contribution ECC. graphical user interface also developed help users/researchers will facilitate them estimate practical applications.
Language: Английский
Citations
0Construction and Building Materials, Journal Year: 2025, Volume and Issue: 473, P. 140900 - 140900
Published: March 28, 2025
Language: Английский
Citations
0Sustainable civil infrastructures, Journal Year: 2025, Volume and Issue: unknown, P. 106 - 126
Published: Jan. 1, 2025
Language: Английский
Citations
0Ain Shams Engineering Journal, Journal Year: 2024, Volume and Issue: 16(1), P. 103209 - 103209
Published: Dec. 28, 2024
Language: Английский
Citations
2