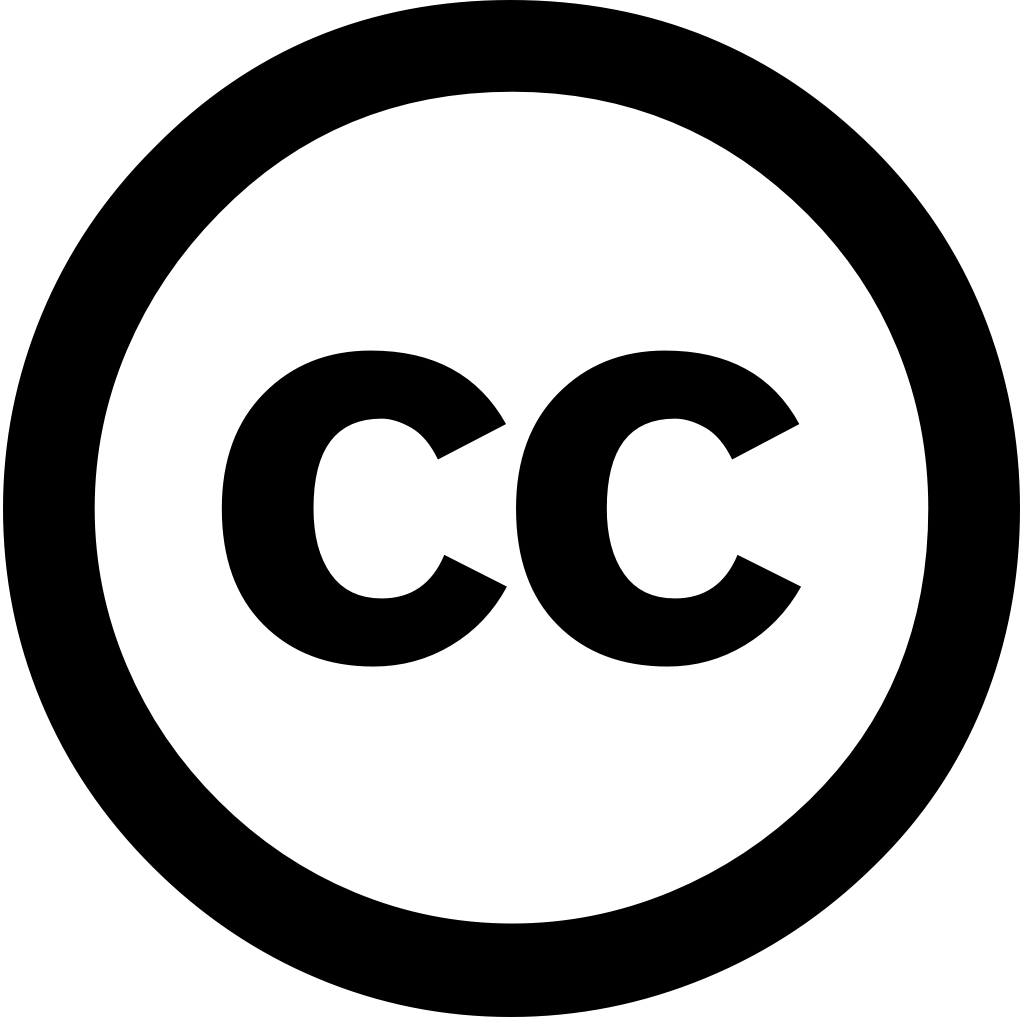
Neurocomputing, Journal Year: 2024, Volume and Issue: 614, P. 128836 - 128836
Published: Nov. 9, 2024
Language: Английский
Neurocomputing, Journal Year: 2024, Volume and Issue: 614, P. 128836 - 128836
Published: Nov. 9, 2024
Language: Английский
Information Fusion, Journal Year: 2025, Volume and Issue: unknown, P. 102928 - 102928
Published: Jan. 1, 2025
Language: Английский
Citations
5Neurocomputing, Journal Year: 2024, Volume and Issue: 573, P. 127218 - 127218
Published: Jan. 5, 2024
Language: Английский
Citations
6Environmental Research, Journal Year: 2024, Volume and Issue: 258, P. 119248 - 119248
Published: May 31, 2024
Language: Английский
Citations
3BMC Medical Genomics, Journal Year: 2025, Volume and Issue: 18(S1)
Published: Jan. 13, 2025
Abstract Background Drug and protein targets affect the physiological functions metabolic effects of body through bonding reactions, accurate prediction drug-protein target interactions is crucial for drug development. In order to shorten development cycle reduce costs, machine learning methods are gradually playing an important role in field drug-target interactions. Results Compared with other methods, regression-based affinity more representative binding ability. Accurate can effectively time cost retargeting new this paper, a model (WPGraphDTA) based on power graph word2vec proposed. Conclusions model, molecular features module extracted by neural network, then obtained Word2vec method. After feature fusion, they input into three full connection layers obtain value. We conducted experiments Davis Kiba datasets, experimental results showed that WPGraphDTA exhibited good performance.
Language: Английский
Citations
0IET Systems Biology, Journal Year: 2025, Volume and Issue: 19(1)
Published: Jan. 1, 2025
Abstract Metal ions are significant ligands that bind to proteins and play crucial roles in cell metabolism, material transport, signal transduction. Predicting the protein‐metal ion ligand binding residues (PMILBRs) accurately is a challenging task theoretical calculations. In this study, authors employed fused amino acids their derived information as feature parameters predict PMILBRs using three classical machine learning algorithms, yielding favourable prediction results. Subsequently, deep algorithm was incorporated prediction, resulting improved results for sets of Ca 2+ Mg compared previous studies. The validation matrix provided optimal model each ionic residue, exhibiting capability effectively predicting sites metal real protein chains.
Language: Английский
Citations
0International Journal of Quantum Chemistry, Journal Year: 2025, Volume and Issue: 125(7)
Published: March 19, 2025
ABSTRACT Machine learning has revolutionized computational chemistry by improving the accuracy of predicting thermodynamic and kinetic properties like activation energies Gibbs free energies, accelerating materials discovery optimizing reaction conditions in both academic industrial applications. This review investigates recent strides applying advanced machine techniques, including transfer learning, for accurately within complex chemical reactions. It thoroughly provides an extensive overview pivotal methods utilized this domain, sophisticated neural networks, Gaussian processes, symbolic regression. Furthermore, prominently highlights commonly adopted frameworks, such as Chemprop, SchNet, DeepMD, which have consistently demonstrated remarkable exceptional efficiency properties. Moreover, it carefully explores numerous influential studies that notably reported substantial successes, particularly focusing on predictive performance, diverse datasets, innovative model architectures profoundly contributed to enhancing methodologies. Ultimately, clearly underscores transformative potential significantly power intricate systems, bearing considerable implications cutting‐edge theoretical research practical
Language: Английский
Citations
0Trends in Food Science & Technology, Journal Year: 2024, Volume and Issue: 153, P. 104700 - 104700
Published: Sept. 5, 2024
Language: Английский
Citations
3Neurocomputing, Journal Year: 2024, Volume and Issue: 594, P. 127833 - 127833
Published: May 9, 2024
Language: Английский
Citations
2Information Sciences, Journal Year: 2024, Volume and Issue: 684, P. 121286 - 121286
Published: Aug. 3, 2024
Language: Английский
Citations
2Innovative Infrastructure Solutions, Journal Year: 2024, Volume and Issue: 10(1)
Published: Dec. 26, 2024
Language: Английский
Citations
2