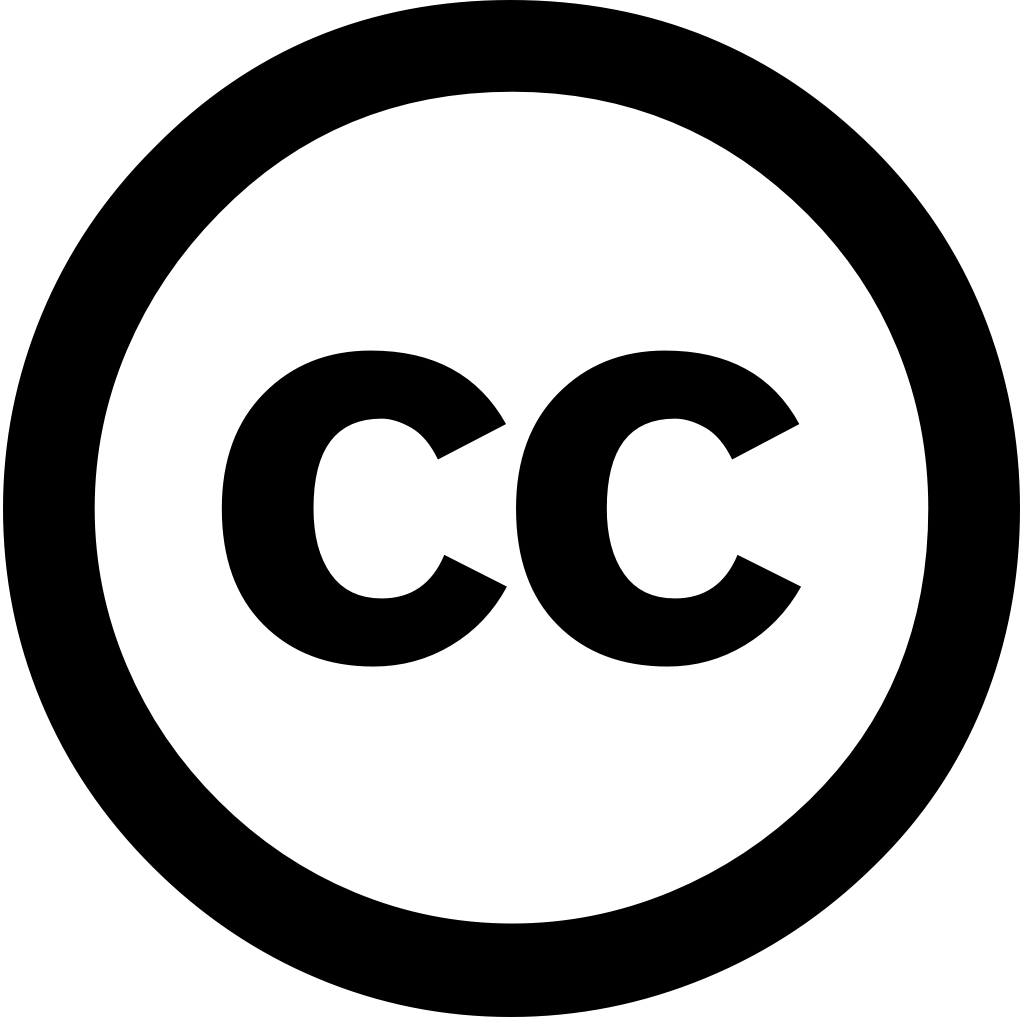
Scientific Reports, Journal Year: 2024, Volume and Issue: 14(1)
Published: Oct. 1, 2024
Language: Английский
Scientific Reports, Journal Year: 2024, Volume and Issue: 14(1)
Published: Oct. 1, 2024
Language: Английский
International Journal of Human-Computer Interaction, Journal Year: 2025, Volume and Issue: unknown, P. 1 - 10
Published: Feb. 12, 2025
Language: Английский
Citations
0Scientific Reports, Journal Year: 2025, Volume and Issue: 15(1)
Published: March 5, 2025
To effectively navigate a crowded and dynamic visual world, our neurocognitive system possesses the remarkable ability to extract learn its statistical regularities implicitly guide allocation of spatial attention resources in immediate future. The way through which we deploy space has been consistently outlined by "center-surround inhibition" pattern, wherein ring sustained inhibition is projected around center attentional focus optimize signal-noise ratio between goal-relevant targets interfering distractors. While it observed that experience-dependent mechanisms could disrupt inhibitory ring, whether learning contingencies an effect on such surround - if any exact unravels are hitherto unexplored questions. Therefore, search psychophysical experiment, aimed fill this gap entirely mapping visuo-spatial profile, asking subjects (N = 26) detect report orientation 'C' letter appearing either as color singleton (Baseline Condition) or non-salient probe (Probe among other irrelevant objects at progressively increasing probe-to-singleton distances. Critically, manipulated contingency so make appear more frequently adjacent probe, specifically distance where attending generates surround-inhibition hindering performance. Results showed markedly reshaped focus, transforming center-surround profile into non-linear gradient one performance gain over high probability distance. Noteworthy, reshaping was uneven time asymmetric, varied across blocks appeared only within quadrants, leaving unaltered unmanipulated ones. Our findings offer insights theoretical interest understanding how environmental orchestrate allocate plastic re-weighting priority maps. Additionally, going beyond physical dimension, data provide interesting implications about information coded working memory representations, especially under scenarios heightened uncertainty.
Language: Английский
Citations
0Published: April 10, 2025
Language: Английский
Citations
0Scientific Reports, Journal Year: 2024, Volume and Issue: 14(1)
Published: Oct. 1, 2024
Language: Английский
Citations
1