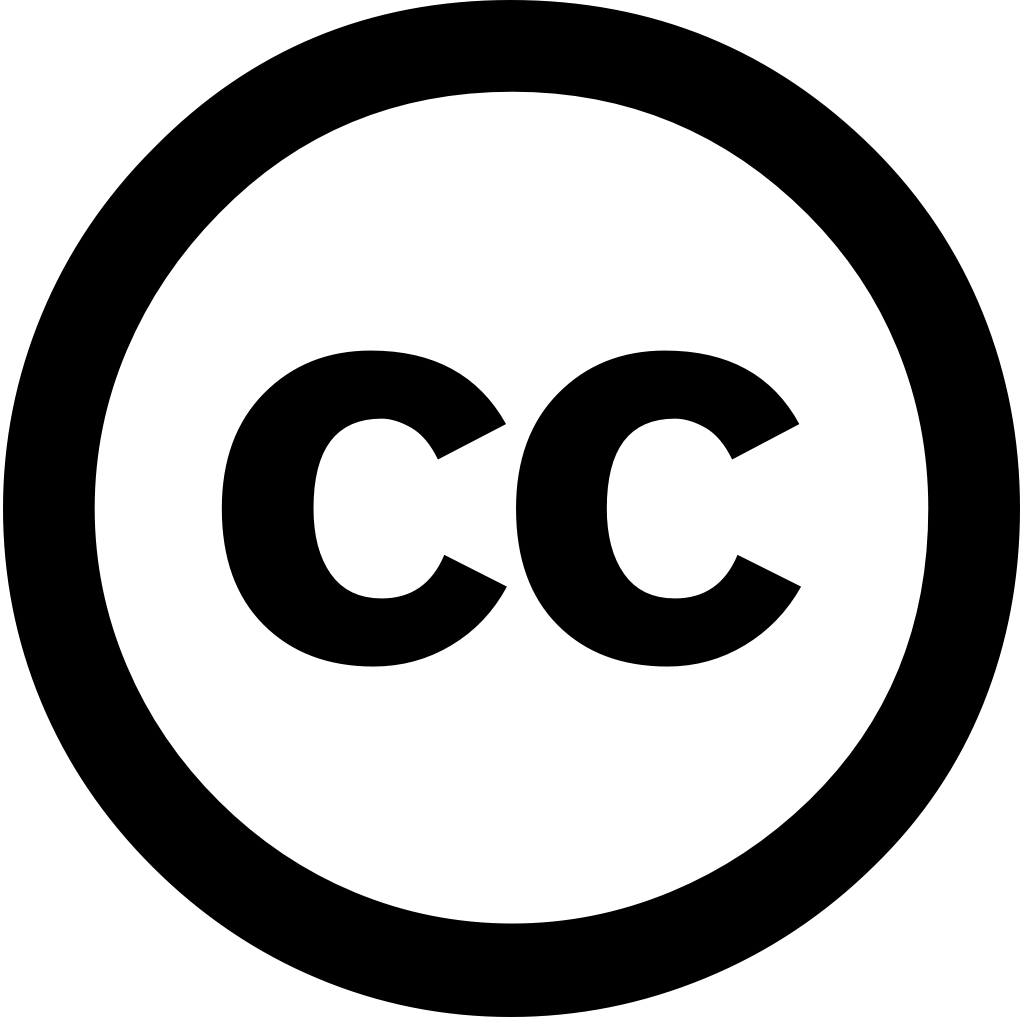
Quaternary Science Advances, Journal Year: 2024, Volume and Issue: unknown, P. 100263 - 100263
Published: Dec. 1, 2024
Language: Английский
Quaternary Science Advances, Journal Year: 2024, Volume and Issue: unknown, P. 100263 - 100263
Published: Dec. 1, 2024
Language: Английский
Natural Hazards, Journal Year: 2025, Volume and Issue: unknown
Published: May 17, 2025
Language: Английский
Citations
0Geological Journal, Journal Year: 2025, Volume and Issue: unknown
Published: May 19, 2025
ABSTRACT Landslides pose a serious threat, especially in mountainous regions, where they can severely impact human life, property and economic activities. This hazard has been particularly challenging the Himalayan region, Sikkim Himalayas. In this study, hybrid models are used to generate landslide susceptibility maps (LSMs) South Himalaya region of India that could significantly help reducing risk. To construct an accurate map, detailed 163 inventories were created using historical data, Google Earth field investigations. A Random Forest (RF) model was employed identify relevant factors revealed 15 significant variables, out which, distance from road rainfall emerged as primary drivers The Stacking Ensemble combines three classic machine learning models, such Artificial Neural Networks (ANNs), Support Vector Machines (SVMs) Gradient Boosting Decision Trees (GBDTs) avoid overfitting improve generalisation. For modelling, data randomly divided into training (70%) validation (30%) subsets. evaluated receiver operating characteristic (ROC) curve statistical metrics. results showed ANN had highest individual prediction performance with area under (AUC) value 0.92, followed by GBDT (0.89), SVM (0.83) method (0.89). However, performed best generalisation class‐specific accuracy, very high (0.54%) compared other models. study highlight model's promise reliable tool for mapping showing it performs better than alternative methods precisely identifying landslide‐prone locations. combination many inside framework markedly improves dependability accuracy these maps. utilisation enhances understanding dynamics establishes scientific foundation formulating efficient risk reduction methods. improvement offers critical insights policy‐making focused on risks supports long‐term regional growth sustainability, geologically vulnerable areas.
Language: Английский
Citations
0Quaternary Science Advances, Journal Year: 2024, Volume and Issue: unknown, P. 100263 - 100263
Published: Dec. 1, 2024
Language: Английский
Citations
1