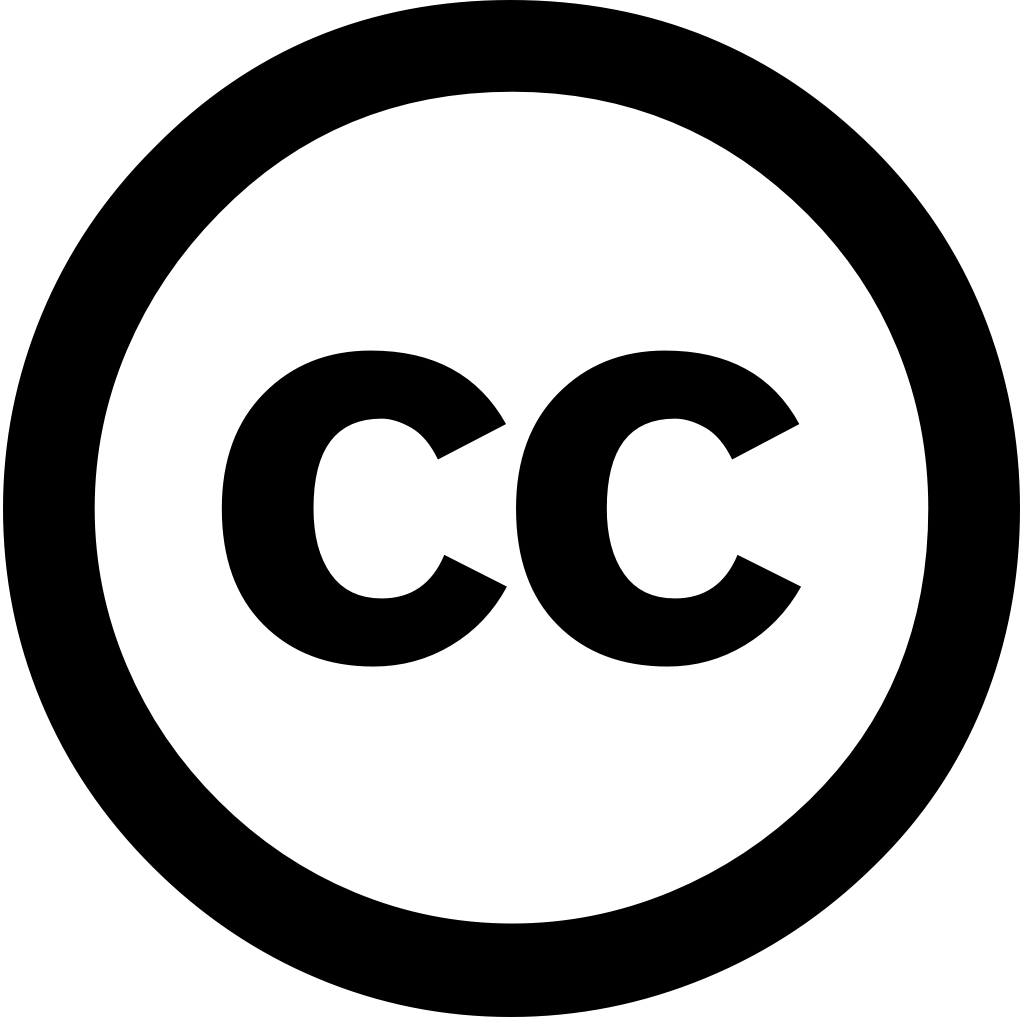
Water Science & Technology, Journal Year: 2024, Volume and Issue: 89(7), P. 1613 - 1629
Published: March 28, 2024
This study develops a novel double-loop contraction and
Language: Английский
Water Science & Technology, Journal Year: 2024, Volume and Issue: 89(7), P. 1613 - 1629
Published: March 28, 2024
This study develops a novel double-loop contraction and
Language: Английский
Engineering Applications of Artificial Intelligence, Journal Year: 2024, Volume and Issue: 133, P. 108573 - 108573
Published: May 11, 2024
Understanding the effects of extreme floods on critical infrastructures such as bridges is paramount for ensuring safety and resilient design in face climate change events. This study develops robust computational predictive modeling tools assessing impacts hydraulic response structural resilience bridges. A fluid dynamic (CFD) model utilizing RANS equations k-ω Shear Stress Transport (SST) simulating supercritical flows adopted to compute hydrodynamic pressures water levels bridge piers cylindrical rectangular shapes during a flood event. The CFD validated based case data obtained from Haj Omran Bridge, built Khorramabad River Iran. numerical simulations consider hydrological conditions exclude geotechnical parameters abutment damages. results are evaluated well-established guidelines. Machine learning techniques, including Extreme Gradient Boosting (XGBoost), Random Forest (RF), Support Vector Regression (SVR), optimized with Grid Search Cross-Validation (GSCV), enhance accuracy pressure forecasting at piers. XGBoost exhibits superior performance (R2 = 0.908, RMSE 0.0279, E 3.41%) compared RF SVR models. All estimated by falls within ±6 percent error lines, highlighting model's robustness out-of-range prediction. Additionally, an Long Short-Term Memory (LSTM) effectively predict free surface flow profiles (i.e. depth) over 0.937 0.083), demonstrating its potential practical applications depth predictions infrastructures. proposed methodological framework outlined this can facilitate bridges, enabling assessment
Language: Английский
Citations
41Frontiers in Built Environment, Journal Year: 2024, Volume and Issue: 10
Published: Feb. 9, 2024
Seawalls are critical defence infrastructures in coastal zones that protect hinterland areas from storm surges, wave overtopping and soil erosion hazards. Scouring at the toe of sea defences, caused by wave-induced accretion bed material imposes a significant threat to structural integrity infrastructures. Accurate prediction scour depths is essential for appropriate efficient design maintenance structures, which serve mitigate risks failure through scouring. However, limited guidance predictive tools available estimating scouring sloping structures. In recent years, Artificial Intelligence Machine Learning (ML) algorithms have gained interest, although they underpin robust models many engineering applications, such yet be applied prediction. Here we develop present ML-based predicting seawall. Four ML algorithms, namely, Random Forest (RF), Gradient Boosted Decision Trees (GBDT), Neural Networks (ANNs), Support Vector Regression (SVMR) utilised. Comprehensive physical modelling measurement data utilised validate models. A Novel framework feature selection, importance, hyperparameter tuning adopted pre- post-processing steps In-depth statistical analyses proposed evaluate performance The results indicate minimum 80% accuracy across all tested this study overall, SVMR produced most accurate predictions with Coefficient Determination ( r 2 ) 0.74 Mean Absolute Error (MAE) value 0.17. algorithm also offered computationally among tested. methodological can datasets rapid assessment facilitating model-informed decision-making.
Language: Английский
Citations
25Heliyon, Journal Year: 2024, Volume and Issue: 10(20), P. e37965 - e37965
Published: Sept. 30, 2024
Accurate prediction of daily river flow (Q t ) remains a challenging yet essential task in hydrological modeling, particularly crucial for flood mitigation and water resource management. This study introduces an advanced M5 Prime (M5P) predictive model designed to estimate Q as well one- two-day-ahead forecasts (i.e. t+1 t+2 ). The performance M5P ensembles incorporating Bootstrap Aggregation (BA), Disjoint Aggregating (DA), Additive Regression (AR), Vote (V), Iterative classifier optimizer (ICO), Random Subspace (RS), Rotation Forest (ROF) were comprehensively evaluated. proposed models applied case data Tuolumne County, US, using dataset comprising measured precipitation (P ), evaporation (E t), . A wide range input scenarios explored predicting , t+1, t+2. Results indicate that P significantly influence accuracy. Notably, relying solely on the most correlated variable (e.g., t-1) does not guarantee robust However, extending forecast horizon mitigates low-correlation variables Performance metrics DA-M5P achieves superior results, with Nash-Sutcliff Efficiency 0.916 root mean square error 23 m3/s, followed by ROF-M5P, BA-M5P, AR-M5P, RS-M5P, V-M5P, ICO-M5P, standalone model. ensemble modeling framework enhanced capability stand-alone algorithm 1.2 %-22.6 %, underscoring its efficacy potential advancing forecasting.
Language: Английский
Citations
18Environmental Science and Pollution Research, Journal Year: 2025, Volume and Issue: unknown
Published: Feb. 24, 2025
Language: Английский
Citations
3Journal of Hydrology, Journal Year: 2025, Volume and Issue: unknown, P. 132905 - 132905
Published: Feb. 1, 2025
Language: Английский
Citations
2Ecological Informatics, Journal Year: 2024, Volume and Issue: 85, P. 102933 - 102933
Published: Dec. 7, 2024
Language: Английский
Citations
11Journal of Water Process Engineering, Journal Year: 2024, Volume and Issue: 63, P. 105512 - 105512
Published: May 24, 2024
Nitrous oxide (N2O) is a significant contributor to global greenhouse gas emissions, with an increasing attention for its mitigation. Machine learning (ML) models hold promise as alternative mechanistic in N2O prediction from wastewater treatment plants (WWTPs). Although more complex ML can sometimes enhance performance, they may also yield little no improvement. Thus, balancing model complexity performance essential effective models, aspect that often overlooked. Carefully these elements optimizing efficacy without unnecessarily complexity. Hence, this study exhaustively investigates the broadly adopted hyperparameter optimization (HPO), grid search optimization, which showed limited ability consider and it only focuses on leading potential overfitting. This emphasizes crucial balance between presenting new algorithm combines input feature selection HPO efficiency accuracy. Consequently, AdaBoost achieved same accuracy crafted through separate HPO, holding R2 of 0.94 but marginal increase RMSE 27.25 26.27. It simplified by using fewer estimators shallower trees, thereby lowering risk overfitting suggesting better generalizability. The employs multi-objective NSGA-II genetic (GA), outperforming Nelder-Mead algorithm. approach effectively balances accuracy, enabling development computationally efficient, online tools emission potentially other applications.
Language: Английский
Citations
9Applied Soft Computing, Journal Year: 2025, Volume and Issue: 170, P. 112652 - 112652
Published: Jan. 8, 2025
Language: Английский
Citations
1Ecological Indicators, Journal Year: 2024, Volume and Issue: 165, P. 112230 - 112230
Published: June 12, 2024
Language: Английский
Citations
6Ecological Indicators, Journal Year: 2024, Volume and Issue: 166, P. 112392 - 112392
Published: July 23, 2024
Language: Английский
Citations
6