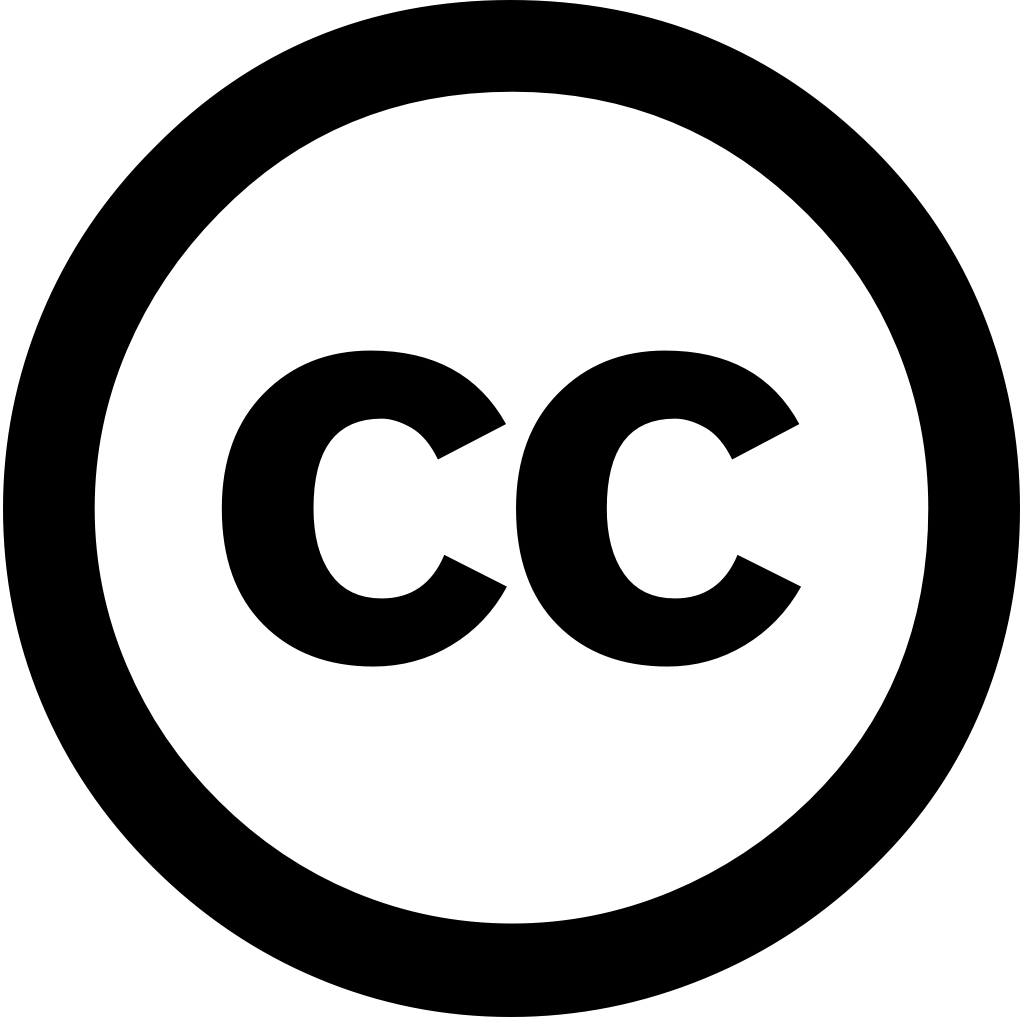
Journal of Marine Science and Engineering, Journal Year: 2024, Volume and Issue: 12(12), P. 2315 - 2315
Published: Dec. 17, 2024
In the field of research into vessel type recognition utilizing trajectory data, researchers have primarily concentrated on developing models based sequences to extract relevant information. However, this approach often overlooks crucial significance spatial dependency relationships among points, posing challenges for comprehensively capturing intricate features travel patterns. To address limitation, our study introduces a novel multi-graph fusion representation method that integrates both and optimize task recognition. The proposed initially extracts spatiotemporal behavioral semantic from trajectories. By these features, key nodes within exhibit dependencies are identified. Subsequently, graph structures constructed represent between points. These then processed through convolutional networks (GCNs), which integrate various sources information graphs obtain representations Finally, applied experimental validation. results indicate significantly enhances performance when compared other baseline methods. Additionally, ablation experiments been conducted validate effectiveness each component method. This innovative not only delves deeply trajectories but also contributes advancements in intelligent water traffic control.
Language: Английский