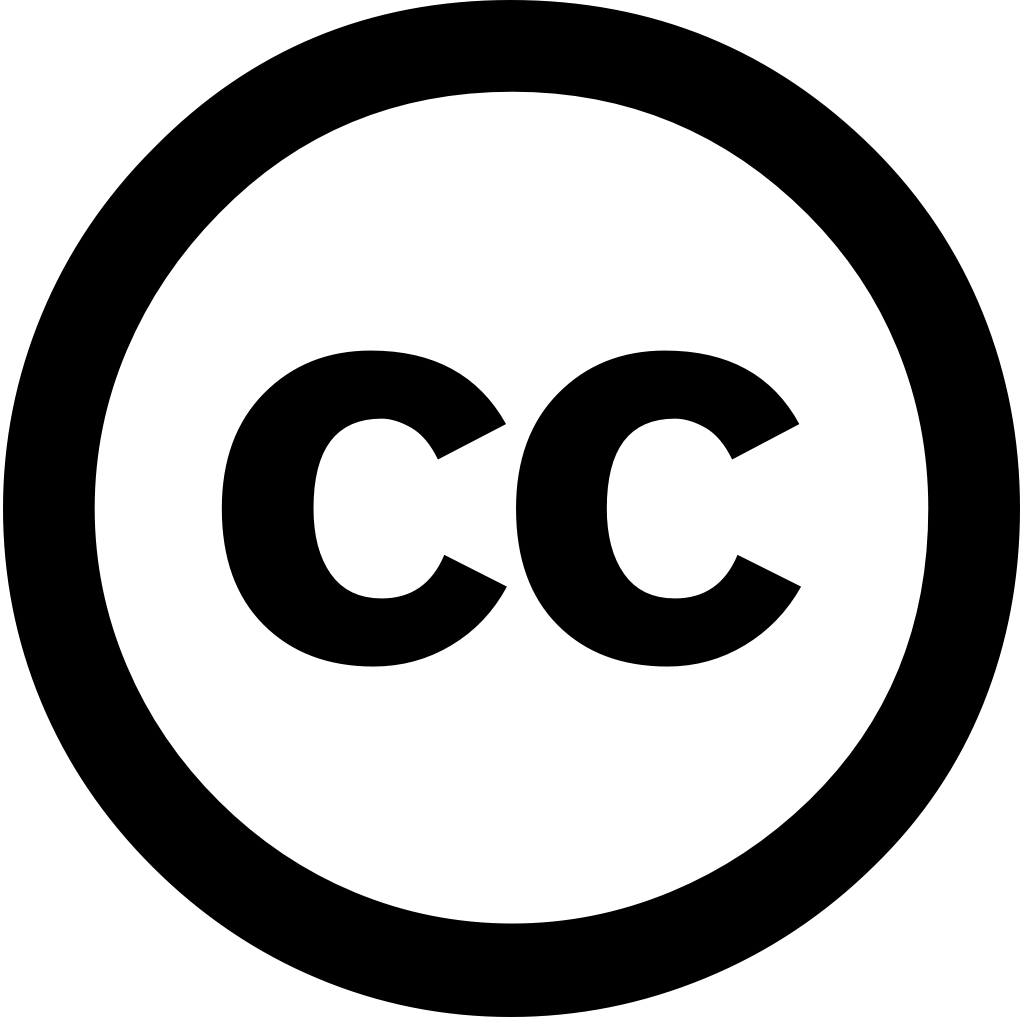
Sensors, Journal Year: 2025, Volume and Issue: 25(5), P. 1401 - 1401
Published: Feb. 25, 2025
The governing Partial Differential Equation (PDE) for wave propagation or the equation involves multi-scale and multi-dimensional oscillatory phenomena. Wave PDE challenges traditional computational methods due to high costs with rigid assumptions. advent of scientific machine learning (SciML) presents a novel paradigm by embedding physical laws within neural network architectures, enabling efficient accurate solutions. This study explores evolution SciML approaches, focusing on PINNs, evaluates their application in modeling acoustic, elastic, guided propagation. PINN is gray-box predictive model that offers strong capabilities data-driven models but also adheres laws. Through theoretical analysis problem-driven examples, findings demonstrate PINNs address key limitations methods, including discretization errors inefficiencies, while offering robust capabilities. Despite current challenges, such as optimization difficulties scalability constraints, hold transformative potential advancing modeling. comprehensive underscores PINN, followed recommendations why how it could advance sets stage future research field Structural Health Monitoring (SHM)/Nondestructive Evaluation (NDE).
Language: Английский