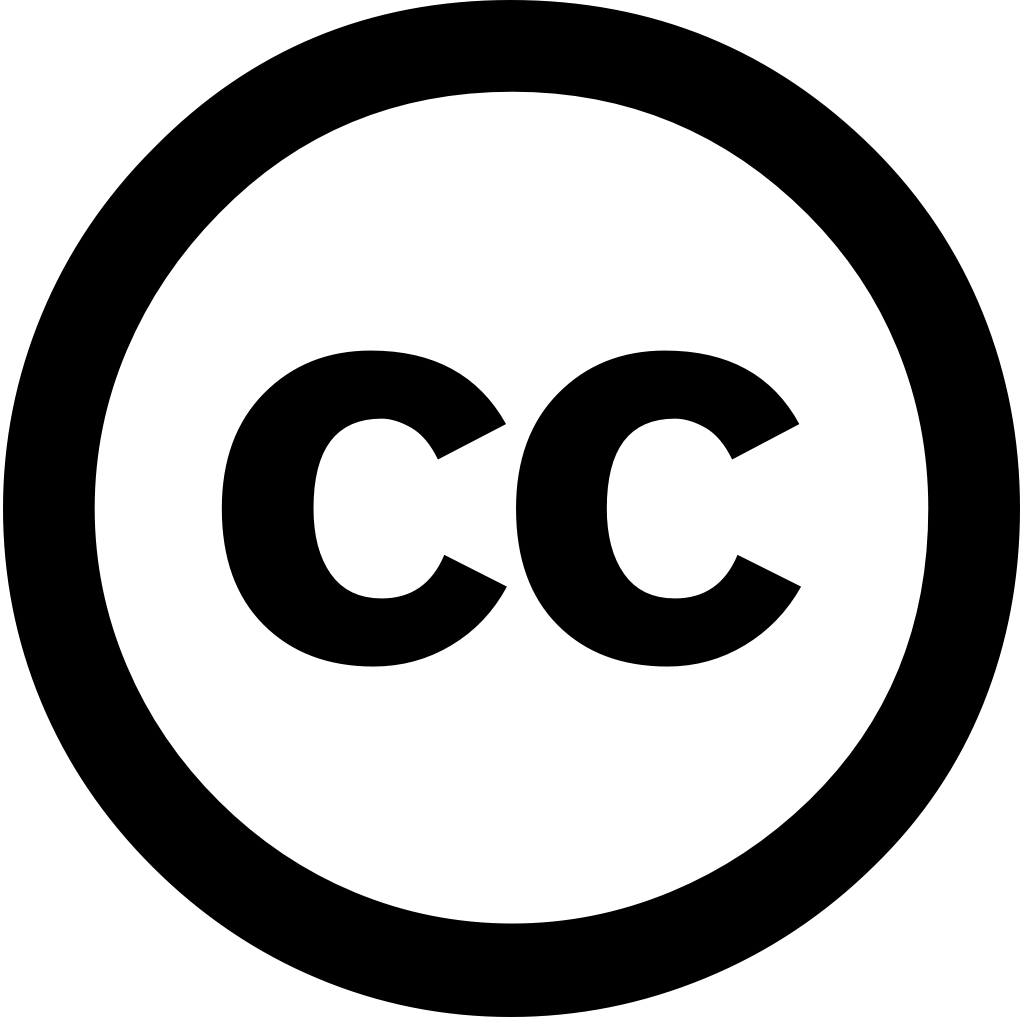
Discover Geoscience, Journal Year: 2025, Volume and Issue: 3(1)
Published: April 28, 2025
Language: Английский
Discover Geoscience, Journal Year: 2025, Volume and Issue: 3(1)
Published: April 28, 2025
Language: Английский
The Science of The Total Environment, Journal Year: 2024, Volume and Issue: 921, P. 171204 - 171204
Published: Feb. 23, 2024
Language: Английский
Citations
26Earth Science Informatics, Journal Year: 2024, Volume and Issue: 17(2), P. 1433 - 1457
Published: Jan. 15, 2024
Language: Английский
Citations
18Remote Sensing, Journal Year: 2025, Volume and Issue: 17(3), P. 524 - 524
Published: Feb. 3, 2025
Climate change has led to an increase in global temperature and frequent intense precipitation, resulting a rise severe urban flooding worldwide. This growing threat is exacerbated by rapid urbanization, impervious surface expansion, overwhelmed drainage systems, particularly regions. As becomes more catastrophic causes significant environmental property damage, there urgent need understand address flood susceptibility mitigate future damage. review aims evaluate remote sensing datasets key parameters influencing provide comprehensive overview of the causative factors utilized mapping. also highlights evolution traditional, data-driven, big data, GISs (geographic information systems), machine learning approaches discusses advantages limitations different mapping approaches. By evaluating challenges associated with current practices, this paper offers insights into directions for improving management strategies. Understanding identifying foundation developing effective resilient practices will be beneficial mitigating
Language: Английский
Citations
2Journal of Hydrology, Journal Year: 2023, Volume and Issue: 624, P. 129961 - 129961
Published: July 19, 2023
Language: Английский
Citations
30Applied Soft Computing, Journal Year: 2023, Volume and Issue: 148, P. 110846 - 110846
Published: Sept. 13, 2023
Language: Английский
Citations
25Remote Sensing, Journal Year: 2024, Volume and Issue: 16(2), P. 336 - 336
Published: Jan. 15, 2024
Flooding is a natural disaster that coexists with human beings and causes severe loss of life property worldwide. Although numerous studies for flood susceptibility modelling have been introduced, notable gap has the overlooked or reduced consideration uncertainty in accuracy produced maps. Challenges such as limited data, due to confidence bounds, overfitting problem are critical areas improving accurate models. We focus on mapping, mainly when there significant variation predictive relevance predictor factors. It also noted receiver operating characteristic (ROC) curve may not accurately depict sensitivity resulting map overfitting. Therefore, reducing was targeted increase improve processing time prediction. This study created spatial repository test models, containing data from historical flooding twelve topographic geo-environmental conditioning variables. Then, we applied random forest (RF) extreme gradient boosting (XGB) algorithms susceptibility, incorporating variable drop-off empirical loop function. The results showed function crucial method resolve model associated factors methods. approximately 8.42% 9.89% Marib City 9.93% 15.69% Shibam were highly vulnerable floods. Furthermore, this significantly contributes worldwide endeavors focused hazards linked disasters. approaches used can offer valuable insights strategies risks, particularly Yemen.
Language: Английский
Citations
16Natural Hazards, Journal Year: 2024, Volume and Issue: 120(11), P. 10365 - 10393
Published: April 23, 2024
Language: Английский
Citations
11Geomatics Natural Hazards and Risk, Journal Year: 2024, Volume and Issue: 15(1)
Published: May 28, 2024
Frequent floods caused by monsoons and rainstorms have significantly affected the resilience of human natural ecosystems in Nam Ngum River Basin, Lao PDR. A cost-efficient framework integrating advanced remote sensing machine learning techniques is proposed to address this issue enhancing flood susceptibility understanding informed decision-making. This study utilizes geo-datasets algorithms (Random Forest, Support Vector Machine, Artificial Neural Networks, Long Short-Term Memory) generate comprehensive maps. The results highlight Random Forest's superior performance, achieving highest train test Area Under Curve Receiver Operating Characteristic (AUROC) (1.00 0.993), accuracy (0.957), F1-score (0.962), kappa value (0.914), with lowest mean squared error (0.207) Root Mean Squared Error (0.043). Vulnerability particularly pronounced low-elevation low-slope southern downstream areas (Central part PDR). reveal that 36%–53% basin's total area highly susceptible flooding, emphasizing dire need for coordinated floodplain management strategies. research uses freely accessible data, addresses data scarcity studies, provides valuable insights disaster risk sustainable planning
Language: Английский
Citations
8Watershed Ecology and the Environment, Journal Year: 2024, Volume and Issue: 6, P. 26 - 40
Published: Jan. 1, 2024
Flash flood causes severe damage to the environment and human life across world, no exception is Bangladesh. Severe flash floods affect northeastern portion of Bangladesh in early monsoon pose a serious threat every aspect socioeconomic development environmental sustainability. To manage reduce loss, map susceptible zones plays key role. Thus, aim this research flood-susceptible areas haor utilizing GIS-based bivariate statistical models. The models utilized are frequency ratio (FR), weights evidence (WoE), certainty factor (CF), Shanon's entropy (SE) information value (IV). Among 250 identified locations, 80% data was used for training purposes 20% testing purposes. Eleven selected conditioning factors include elevation, slope, aspect, curvature, TWI, TRI, SPI, distance stream, stream density, rainfall physiography. calculated assigned using ArcGIS prepare final maps. Results AUC ROC indicate WoE (success rate = 0.833 prediction =0.925) best model susceptibility mapping followed by FR 0.828 =0.928) SE 0.827 =0.923). According models, topographic (flat area) hydrologic significantly control occurrence study area. prepared maps will be helpful disaster managers master planners
Language: Английский
Citations
7Remote Sensing, Journal Year: 2024, Volume and Issue: 16(14), P. 2595 - 2595
Published: July 16, 2024
Flooding is a recurrent hazard occurring worldwide, resulting in severe losses. The preparation of flood susceptibility map non-structural approach to management before its occurrence. With recent advances artificial intelligence, achieving high-accuracy model for mapping (FSM) challenging. Therefore, this study, various intelligence approaches have been utilized achieve optimal accuracy modeling address challenge. By incorporating the grey wolf optimizer (GWO) metaheuristic algorithm into models—including neural networks (RNNs), support vector regression (SVR), and extreme gradient boosting (XGBoost)—the objective generate maps evaluate variation performance. tropical Manimala River Basin India, severely battered by flooding past, has selected as test site. This 15 conditioning factors such aspect, enhanced built-up bareness index (EBBI), slope, elevation, geomorphology, normalized difference water (NDWI), plan curvature, profile soil adjusted vegetation (SAVI), stream density, texture, power (SPI), terrain ruggedness (TRI), land use/land cover (LULC) topographic wetness (TWI). Thus, six are produced applying RNN, SVR, XGBoost, RNN-GWO, SVR-GWO, XGBoost-GWO models. All models exhibited outstanding (AUC above 0.90) performance, performance ranks following order: RNN-GWO (AUC: 0.968) > 0.961) SVR-GWO 0.960) RNN 0.956) XGBoost 0.953) SVR 0.948). It was discovered that hybrid GWO optimization improved three RNN-GWO-based shows 8.05% MRB very susceptible floods. found SPI, LULC, TWI top five influential factors.
Language: Английский
Citations
7