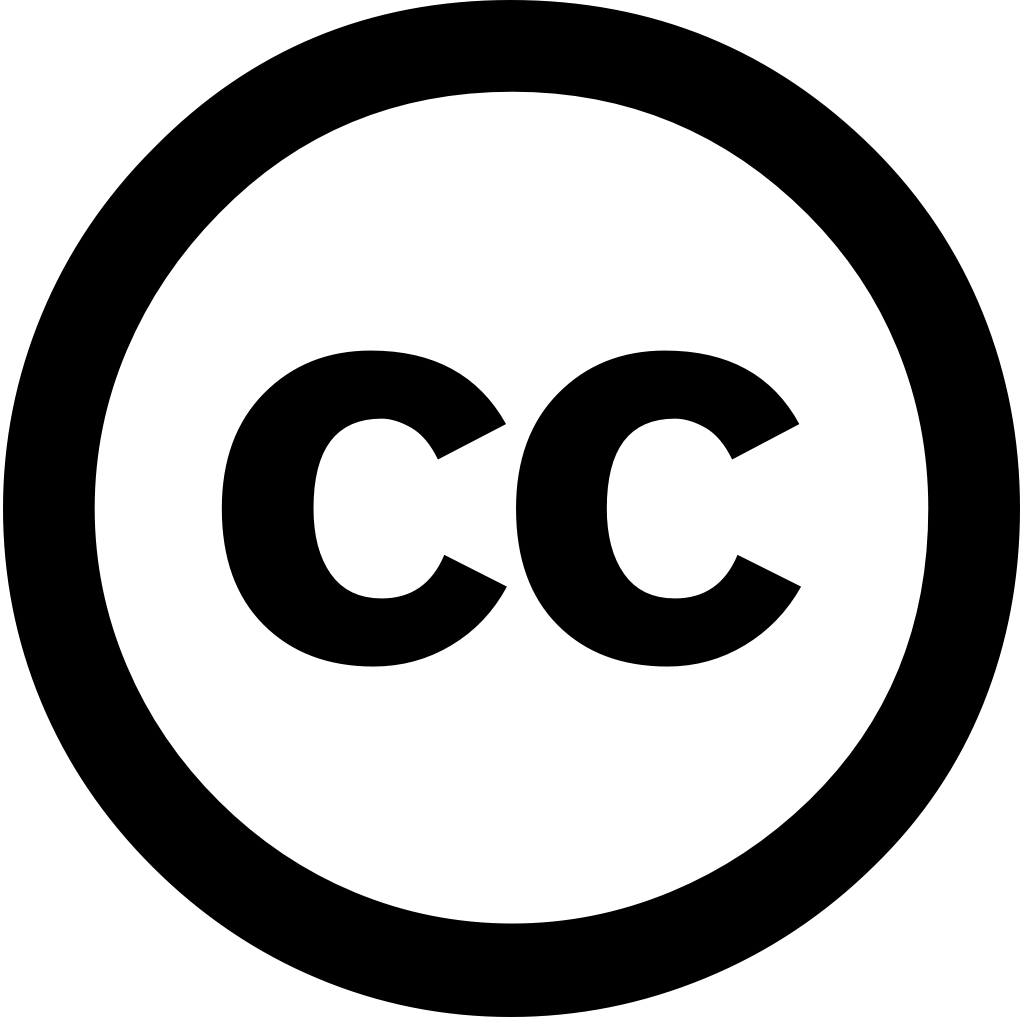
Computers in Biology and Medicine, Journal Year: 2025, Volume and Issue: 192, P. 110160 - 110160
Published: April 22, 2025
The generation of Synthetic Computed Tomography (sCT) images has become a pivotal methodology in modern clinical practice, particularly the context Radiotherapy (RT) treatment planning. use sCT enables calculation doses, pushing towards Magnetic Resonance Imaging (MRI) guided radiotherapy treatments. Moreover, with introduction MRI-Positron Emission (PET) hybrid scanners, derivation from MRI can improve attenuation correction PET images. Deep learning methods for MRI-to-sCT have shown promising results, but their reliance on single-centre training dataset limits generalisation capabilities to diverse settings. creating centralised multi-centre datasets may pose privacy concerns. To address aforementioned issues, we introduced FedSynthCT-Brain, an approach based Federated Learning (FL) paradigm brain imaging. This is among first applications FL MRI-to-sCT, employing cross-silo horizontal that allows multiple centres collaboratively train U-Net-based deep model. We validated our method using real multicentre data four European and American centres, simulating heterogeneous scanner types acquisition modalities, tested its performance independent centre outside federation. In case unseen centre, federated model achieved median Mean Absolute Error (MAE) 102.0 HU across 23 patients, interquartile range 96.7-110.5 HU. (interquartile range) Structural Similarity Index (SSIM) Peak Signal Noise Ratio (PNSR) were 0.89 (0.86-0.89) 26.58 (25.52-27.42), respectively. analysis results showed acceptable performances approach, thus highlighting potential enhance generalisability advancing safe equitable while fostering collaboration preserving privacy.
Language: Английский