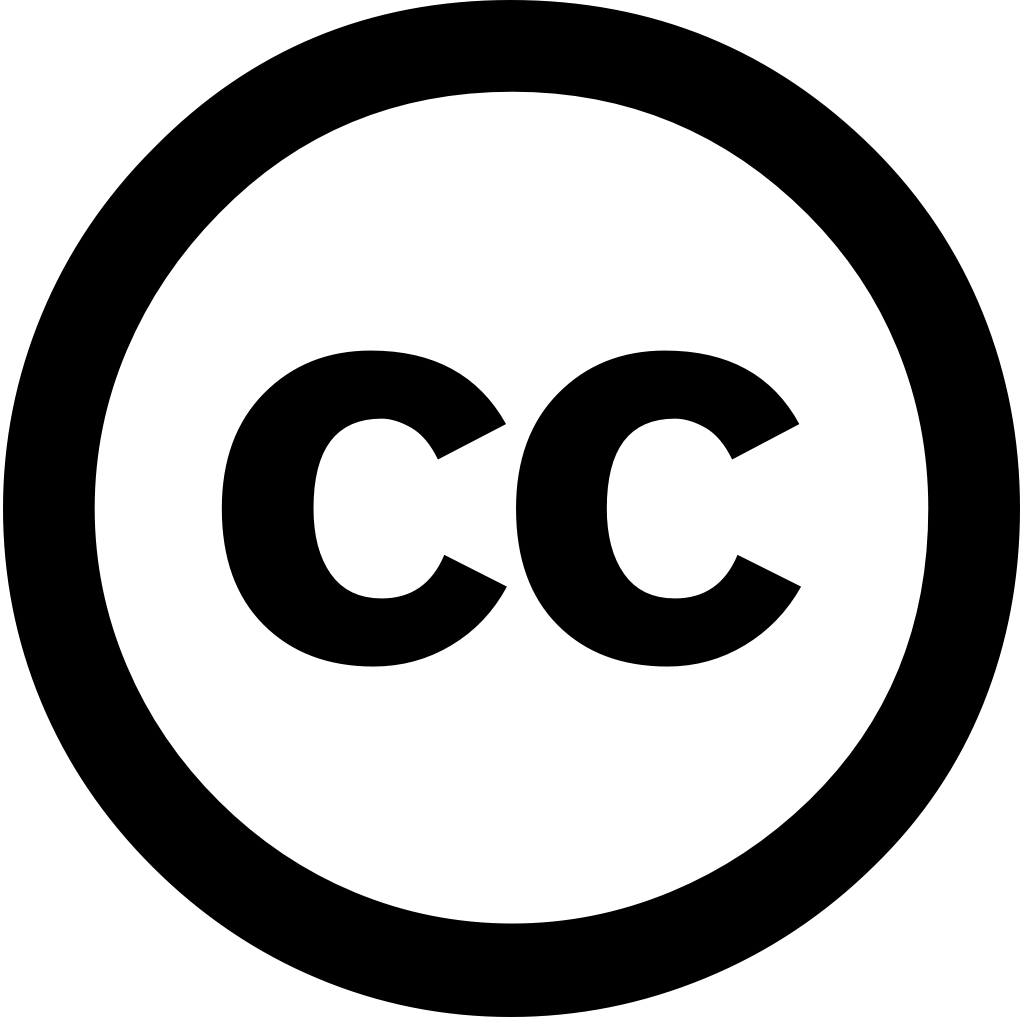
Agronomy, Journal Year: 2025, Volume and Issue: 15(4), P. 788 - 788
Published: March 23, 2025
Broccoli is a highly nutritious vegetable that favored worldwide. Assessing and predicting the shelf life of broccoli holds considerable importance for effective resource optimization management. The physicochemical parameters spectral characteristics are important indicators partially reflecting its life. However, few studies have used image information to predict evaluate broccoli. In this study, multispectral imaging combined with multi-feature data fusion was Spectral textural features were extracted from images fused analysis. Savitzky–Golay (SG) convolution smoothing standard normal variate (SNV) normalization (Norm) preprocessing methods employed preprocess original features, while successive projection algorithm (SPA) extract relevant feature bands. predicted using three methods: support vector regression (SVR), random forest classification (RF), 2D convolutional neural network (2D-CNN) models. prediction models evaluated RF, 1D-CNN, 2D-CNN. results demonstrate that, among evaluating broccoli, SPA+SG+RF model employing Type C achieves highest accuracy. Specifically, method accuracies 88.98% 88.64% training validation sets, respectively. Multi-feature physical chemical different machine learning
Language: Английский