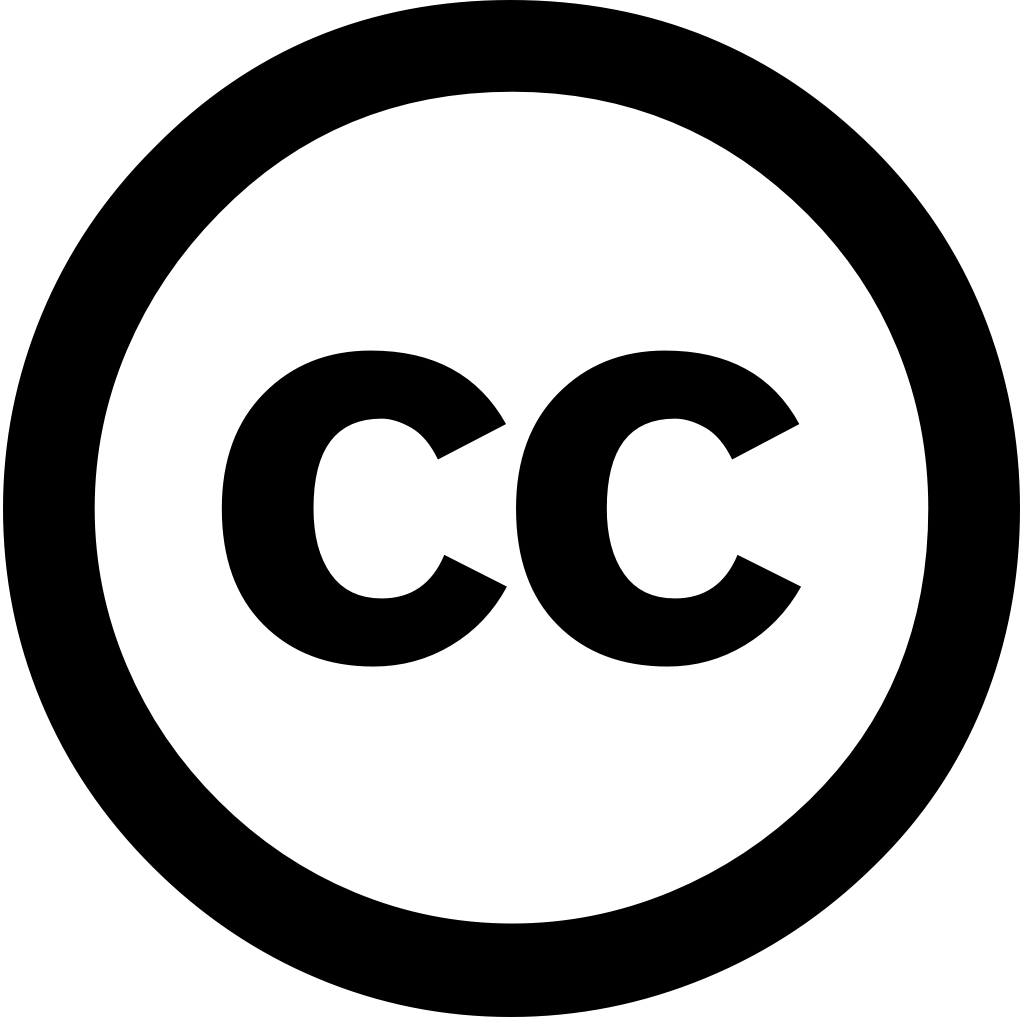
Energy Science & Engineering, Journal Year: 2025, Volume and Issue: unknown
Published: April 29, 2025
ABSTRACT Detection of faults in solar photovoltaic (PV) systems is an important concern to guarantee that renewable energy source generation keeps its efficiency and reliability. It directly affects the development sustainable goals (SDGs), especially those on affordable clean climate action. However, most existing research studies related fault detection methods have suffered from low accuracy, higher error rates, time‐consuming processes when dealing with complex types real‐time. The work therefore targeted at trying address these challenges efficient reliable model could be applicable for PV systems. also proposes Solar FaultNet‐a novel deep learning‐based approach significantly improves performance integrates state‐of‐the‐art ML techniques like CNN LSTM capture inherent patterns interdependencies data. Besides, proposed outperforms conventional machine‐learning algorithms deep‐ learning models better by yielding precision, recall, F1‐score, rate various such as array faults, inverter grid synchronization environmental faults. Experimental results show FaultNet deep‐learning architectures, achieving a precision 99.1%, recall 99%, F1‐score 98.9%, 0.018%. In addition, enjoys high 15 s training time 25 testing handling
Language: Английский