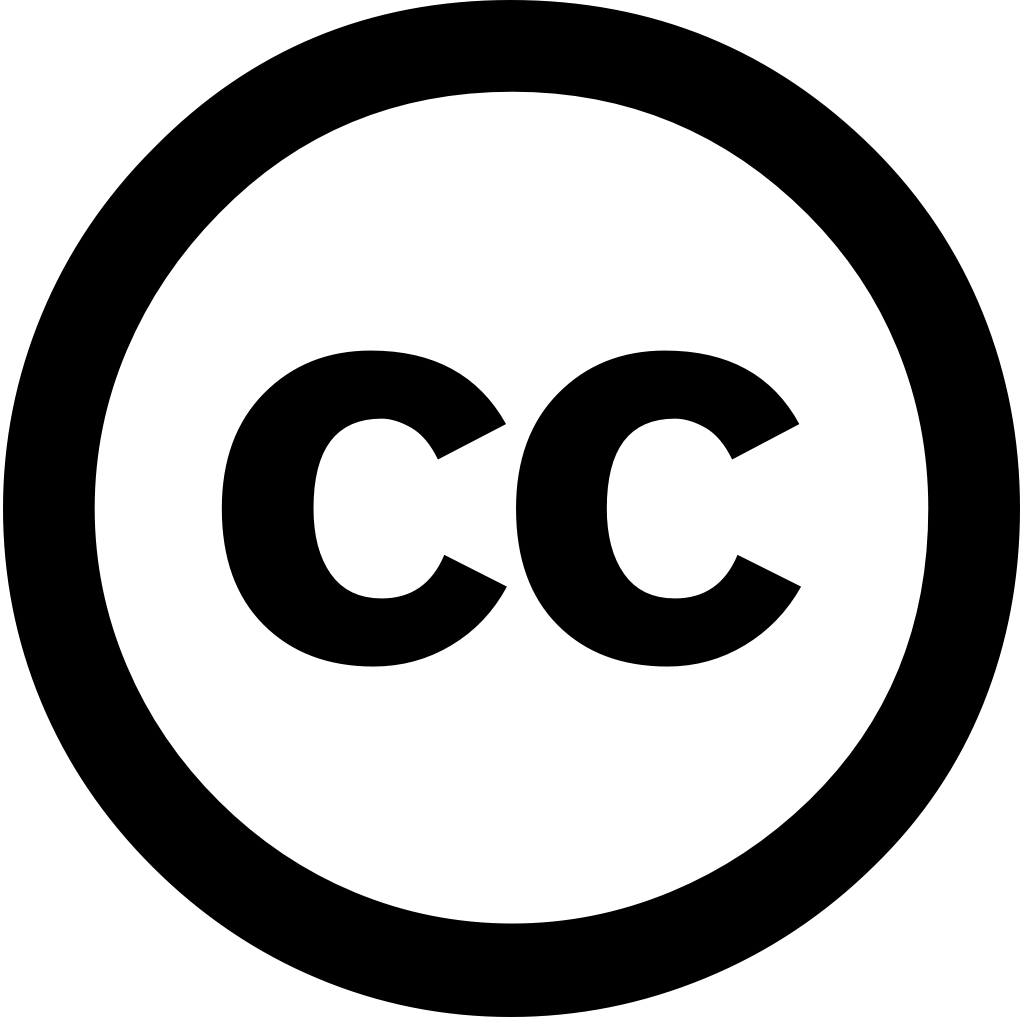
Agronomy, Journal Year: 2024, Volume and Issue: 14(12), P. 2869 - 2869
Published: Dec. 1, 2024
To address the computational complexity and deployment challenges of traditional convolutional neural networks in rice disease identification, this paper proposes an efficient lightweight model: Ghost Channel Spatial Attention ShuffleNet with Mish-ReLU Adaptive Activation Function (GCA-MiRaNet). Based on V2, we effectively reduced model’s parameter count by streamlining layers, decreasing stacking depth, optimizing output channels. Additionally, model incorporates Module as a replacement for 1 × convolutions, further reducing overhead. Innovatively, introduce Mechanism (CSAM) that significantly enhances feature extraction generalization aimed at detection. Through combining advantages Mish ReLU, designed (MAAF), enhancing capacity convergence speed. transfer learning ElasticNet regularization, accuracy has notably improved while avoiding overfitting. Sufficient experimental results indicate GCA-MiRaNet attains precision 94.76% dataset, 95.38% reduction parameters compact size only 0.4 MB. Compared to models such ResNet50 EfficientNet demonstrates significant overall performance, especially embedded devices. This not enables accurate real-time monitoring but also provides viable solution field protection drones Internet Things management systems, advancing process contemporary agricultural smart management.
Language: Английский