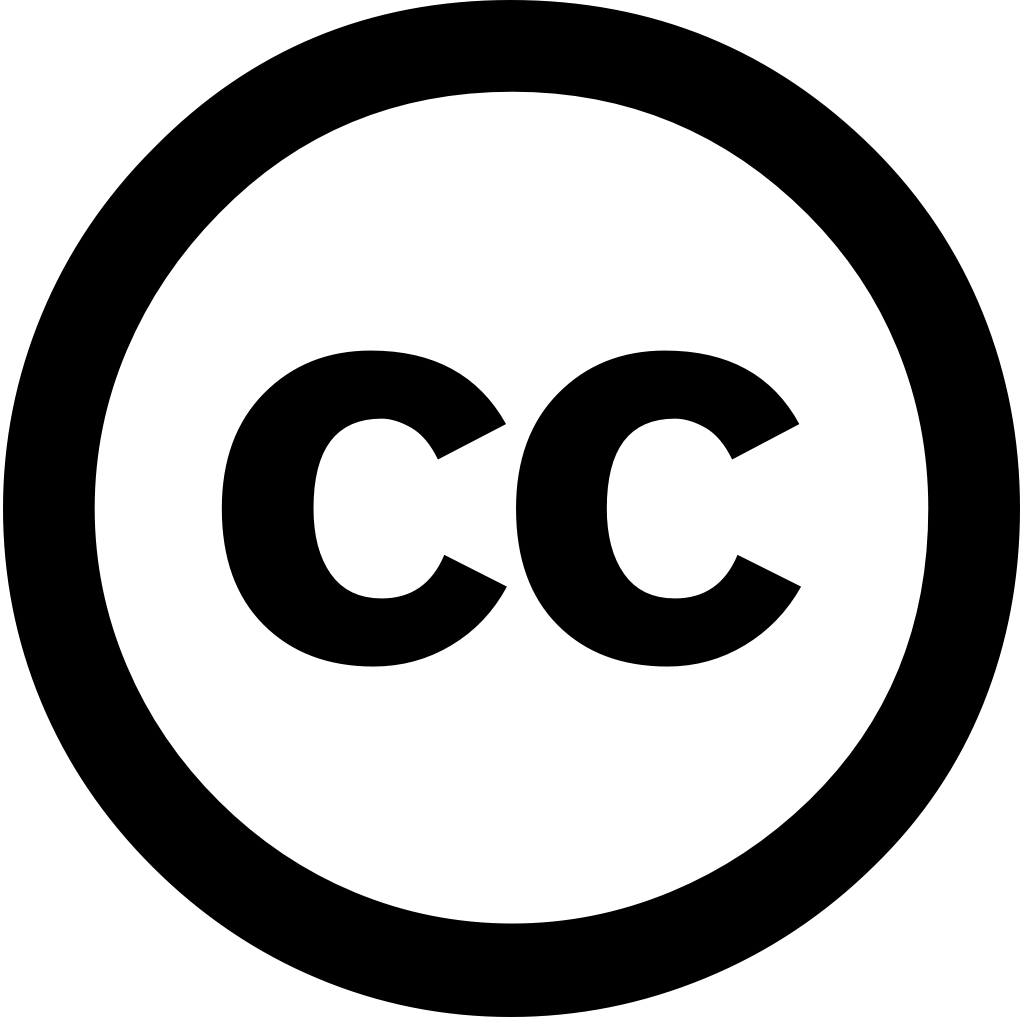
Mathematics, Journal Year: 2025, Volume and Issue: 13(3), P. 342 - 342
Published: Jan. 22, 2025
Ensuring operational reliability and efficiency in steam power plants requires advanced generalized fault detection methodologies capable of addressing diverse scenarios boiler turbine systems. This study presents an autonomous framework that integrates deep feature extraction through Convolutional Autoencoders (CAEs) with the ensemble machine learning technique, Extreme Gradient Boosting (XGBoost). CAEs autonomously extract meaningful nonlinear features from raw sensor data, eliminating need for manual engineering. Principal Component Analysis (PCA) is employed dimensionality reduction, enhancing computational while retaining critical fault-related information. The refined are then classified using XGBoost, a robust algorithm, ensuring accurate detection. proposed model validated real-world case studies on waterwall tube leakage motor-driven oil pump failure turbines. Results demonstrate framework’s ability to generalize across types, detect anomalies at early stage, minimize downtime. highlights transformative potential combining scalable, reliable, efficient plant operations.
Language: Английский