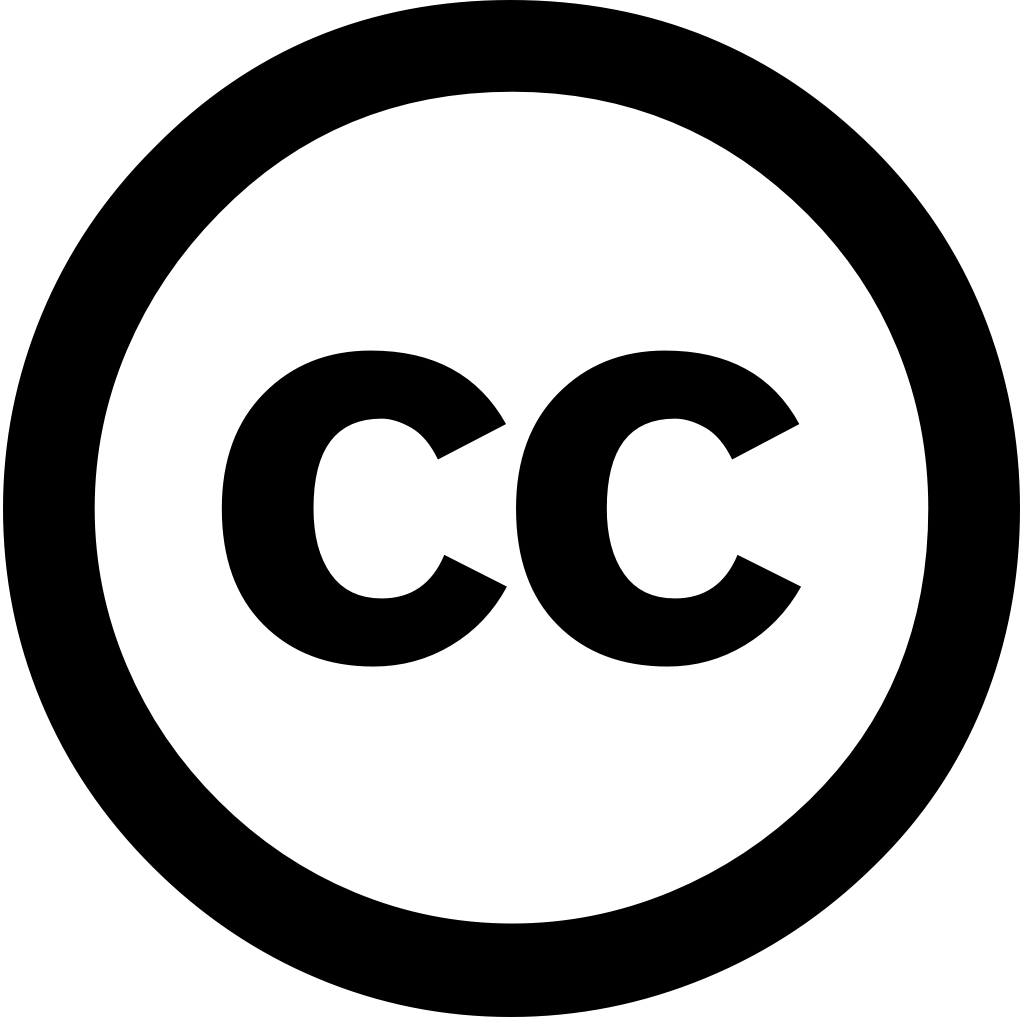
Green Technologies and Sustainability, Journal Year: 2024, Volume and Issue: unknown, P. 100161 - 100161
Published: Dec. 1, 2024
Language: Английский
Green Technologies and Sustainability, Journal Year: 2024, Volume and Issue: unknown, P. 100161 - 100161
Published: Dec. 1, 2024
Language: Английский
Journal of Power Sources, Journal Year: 2025, Volume and Issue: 632, P. 236411 - 236411
Published: Feb. 3, 2025
Language: Английский
Citations
1Journal of Power Sources, Journal Year: 2025, Volume and Issue: 640, P. 236766 - 236766
Published: March 13, 2025
Language: Английский
Citations
0Journal of Energy Storage, Journal Year: 2025, Volume and Issue: 117, P. 116205 - 116205
Published: March 14, 2025
Language: Английский
Citations
0Energies, Journal Year: 2025, Volume and Issue: 18(9), P. 2144 - 2144
Published: April 22, 2025
Accurately estimating the State of Charge (SOC) power batteries is crucial for Battery Management Systems (BMS) in new energy intelligent connected vehicles. It directly influences vehicle range, management efficiency, and safety lifespan battery. However, SOC cannot be measured with instruments; it needs to estimated using external parameters such as current, voltage, internal resistance. Moreover, represent complex nonlinear time-varying systems, various uncertainties—like battery aging, fluctuations ambient temperature, self-discharge effects—complicate accuracy these estimations. This significantly increases complexity estimation process limits industrial applications. To address challenges, this study systematically classifies existing algorithms, performs comparative analyses their computational accuracy, identifies inherent limitations within each category. Additionally, a comprehensive review technologies utilized BMS by automotive OEMs globally conducted. The analysis concludes that advancing multi-fusion frameworks, which offer enhanced universality, robustness, hard real-time capabilities, represents primary research trajectory field.
Language: Английский
Citations
0Scientific Reports, Journal Year: 2024, Volume and Issue: 14(1)
Published: Nov. 23, 2024
Ensuring the long-term safe usage of lithium-ion batteries hinges on accurately estimating State Health $$(\textrm{SOH})$$ and predicting Remaining Useful Life (RUL). This study proposes a novel prediction method based AT-CNN-BiLSTM architecture. Initially, key parameters such as voltage, current, temperature, SOH are extracted averaged for each cycle to ensure uniformity reliability input data. The CNN is utilized extract deep features from data, followed by BiLSTM analyze temporal dependencies in data sequences. Since multidimensional parameter used predict trend batteries, an attention mechanism employed enhance weight highly relevant vectors, improving model's analytical capabilities. Experimental results demonstrate that CNN-BiLSTM-Attention model achieves absolute error 0 RUL prediction, $$R^{2}$$ value greater than 0.9910 , MAPE less 0.9003 . Comparative analysis with hybrid neural network algorithms LSTM, BiLSTM, CNN-LSTM confirms proposed high accuracy stability estimation prediction.
Language: Английский
Citations
1Green Technologies and Sustainability, Journal Year: 2024, Volume and Issue: unknown, P. 100161 - 100161
Published: Dec. 1, 2024
Language: Английский
Citations
0