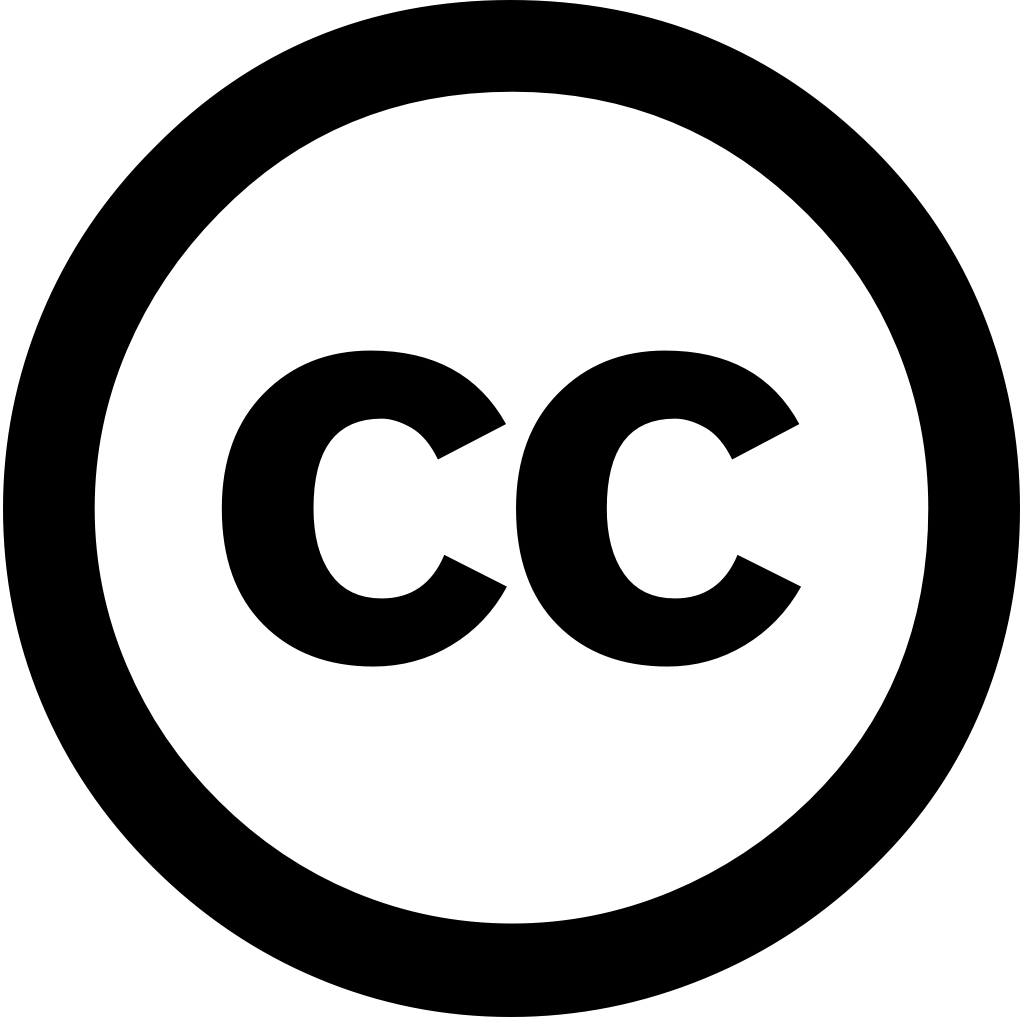
Energies, Journal Year: 2024, Volume and Issue: 17(24), P. 6222 - 6222
Published: Dec. 10, 2024
Solar radiation forecasting is the basis of building a robust solar power system. Most ground-based methods are unable to consider impact cloud changes on future radiation. To alleviate this limitation, study develops hybrid network which relies convolutional neural extract motion patterns from time series satellite observations and long short-term memory establish relationship between information, as well antecedent measurements. We carefully select optimal scales spatial temporal correlations design test experiments at ten stations check model performance in various climate zones. The results demonstrate that accuracy considerably improved, particularly cloudy conditions, compared with purely models. maximum magnitude improvements reaches up 50 W/m2 (15%) terms (relative) root mean squared error (RMSE) for 1 h ahead forecasts. achieves superior forecasts correlation coefficients varying 0.96 0.85 6 ahead. Forecast errors related regimes, amount leads relative RMSE difference about 50% an additional 5% variability. This ascertains multi-source data fusion contributes better simulation impacts combination different deep learning techniques enables more reliable In addition, multi-step low latency make advance planning management energy possible practical applications.
Language: Английский