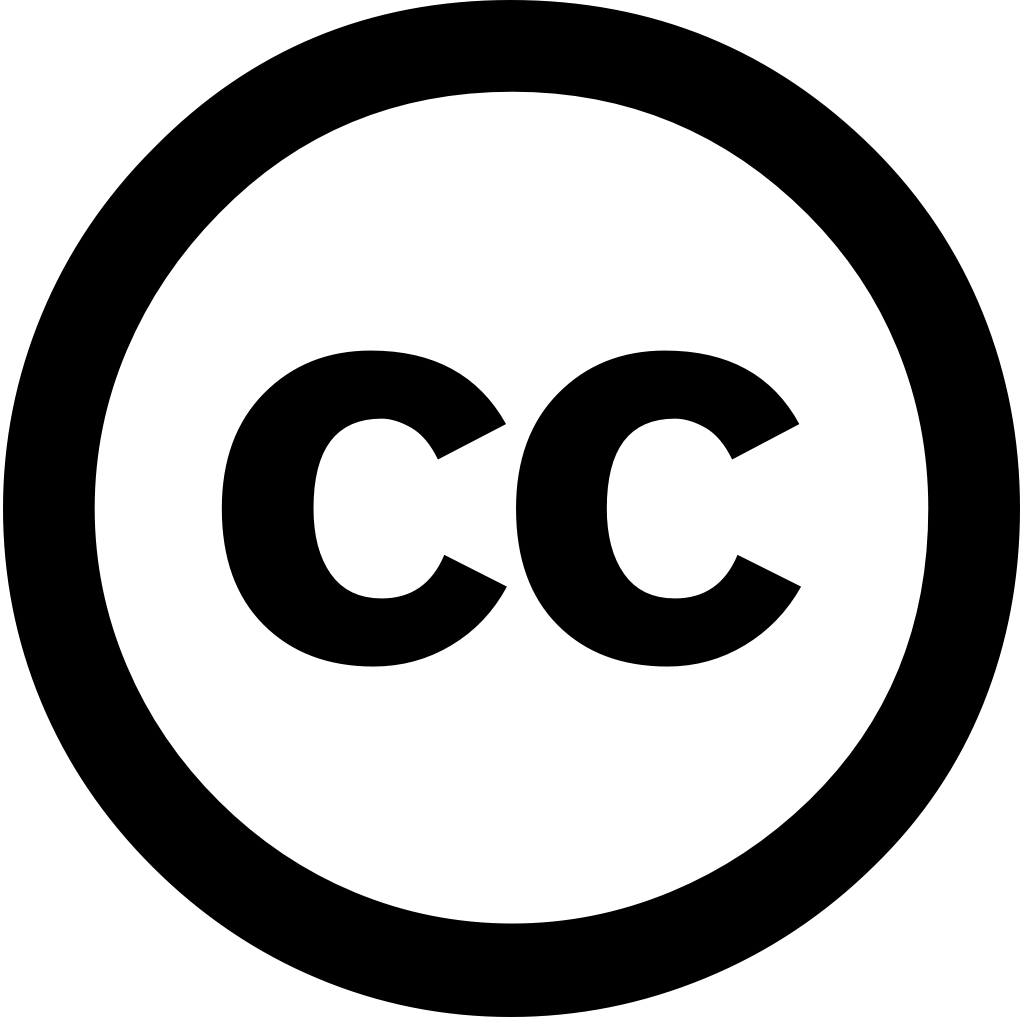
Energy Conversion and Management X, Journal Year: 2024, Volume and Issue: unknown, P. 100767 - 100767
Published: Oct. 1, 2024
Language: Английский
Energy Conversion and Management X, Journal Year: 2024, Volume and Issue: unknown, P. 100767 - 100767
Published: Oct. 1, 2024
Language: Английский
e-Prime - Advances in Electrical Engineering Electronics and Energy, Journal Year: 2025, Volume and Issue: unknown, P. 100997 - 100997
Published: April 1, 2025
Language: Английский
Citations
0Climate Dynamics, Journal Year: 2024, Volume and Issue: 62(5), P. 4041 - 4061
Published: Jan. 19, 2024
Language: Английский
Citations
3Applied Energy, Journal Year: 2024, Volume and Issue: 368, P. 123365 - 123365
Published: May 20, 2024
Power grid damage and blackouts are increasing with climate change. Load forecasting methods that integrate resilience therefore essential to facilitate timely accurate network reconfiguration during periods of extreme stress. Our paper proposes a generalised Wildfire Resilient Forecasting Model (WRLFM) predict electricity load based on operational data Distribution Network (DN) in Australia wildfire seasons 2015–2020. We demonstrate is more challenging than non-wildfire seasons, motivating an imperative need improve forecast performance seasons. To develop the robust WRLFM, comprehensive comparative analyses were conducted determine proper Machine Learning (ML) structures for incorporating multiple factors. Bi-directional Gated Recurrent Unit (Bi-GRU) Vision Transformer (ViT) selected as they performed best among all 13 recently trending ML methods. Multi-factors incorporated contribute performance, including input sequence structures, calendar information, flexible correlation-based temperature conditions, categorical Fire Weather Index (FWI). High-resolution FWI was used build model first time, significantly enhancing average stability performances by 42%. A sensitivity analysis compared set patterns The improvement rate two times greater This indicates significance effectiveness applying WRLFM accuracy under weather risks. Overall, reduces Mean Absolute Percentage Error (MAPE) 14.37% 20.86% Bi-GRU ViT-based models, respectively, achieving MAPE around 3%.
Language: Английский
Citations
3IEEE Transactions on Power Delivery, Journal Year: 2024, Volume and Issue: 40(1), P. 75 - 87
Published: Oct. 24, 2024
Consumer energy forecasting is essential for managing consumption and planning, directly influencing operational efficiency, cost reduction, personalized management, sustainability efforts. In recent years, deep learning techniques, especially LSTMs transformers, have been greatly successful in the field of forecasting. Nevertheless, these techniques difficulties capturing complex sudden variations, and, moreover, they are commonly examined only on a specific type consumer (e.g., offices, schools). Consequently, this paper proposes HyperEnergy, strategy that leverages hypernetworks improved modeling patterns applicable across diversity consumers. Hypernetwork responsible predicting parameters primary prediction network, our case LSTM. A learnable adaptable kernel, comprised polynomial radial basis function kernels, incorporated to enhance performance. The proposed HyperEnergy was evaluated diverse consumers including, student residences, detached homes, home with electric vehicle charging, townhouse. Across all types, consistently outperformed 10 other including state-of-the-art models such as LSTM, AttentionLSTM, transformer.
Language: Английский
Citations
3Energy Conversion and Management X, Journal Year: 2024, Volume and Issue: unknown, P. 100767 - 100767
Published: Oct. 1, 2024
Language: Английский
Citations
3