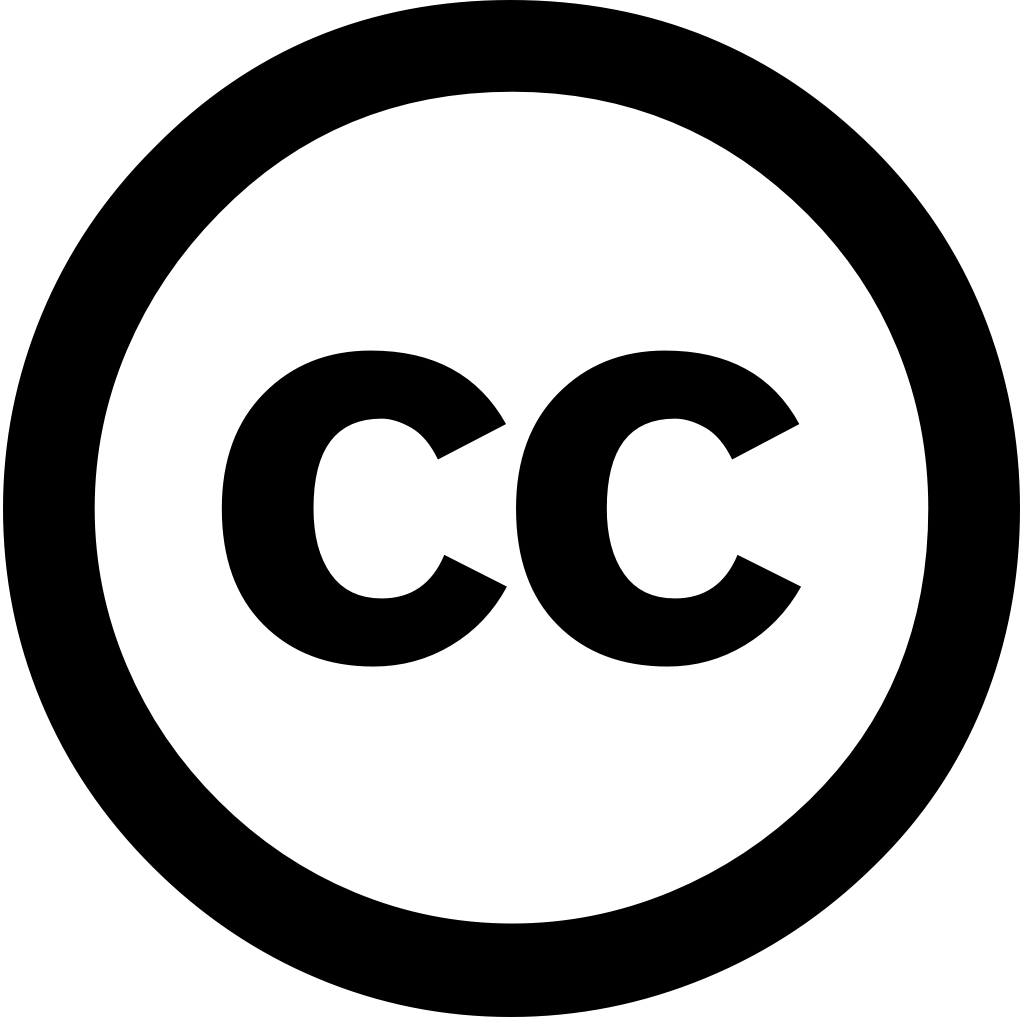
Computers & Chemical Engineering, Journal Year: 2024, Volume and Issue: unknown, P. 108982 - 108982
Published: Dec. 1, 2024
Language: Английский
Computers & Chemical Engineering, Journal Year: 2024, Volume and Issue: unknown, P. 108982 - 108982
Published: Dec. 1, 2024
Language: Английский
Results in Engineering, Journal Year: 2024, Volume and Issue: 21, P. 101837 - 101837
Published: Feb. 6, 2024
Contemporary infrastructure requires structural elements with enhanced mechanical strength and durability. Integrating nanomaterials into concrete is a promising solution to improve However, the intricacies of such nanoscale cementitious composites are highly complex. Traditional regression models encounter limitations in capturing these intricate compositions provide accurate reliable estimations. This study focuses on developing robust prediction for compressive (CS) graphene nanoparticle-reinforced (GrNCC) through machine learning (ML) algorithms. Three ML models, bagging regressor (BR), decision tree (DT), AdaBoost (AR), were employed predict CS based comprehensive dataset 172 experimental values. Seven input parameters, including graphite nanoparticle (GrN) diameter, water-to-cement ratio (wc), GrN content (GC), ultrasonication (US), sand (SC), curing age (CA), thickness (GT), considered. The trained 70 % data, remaining 30 data was used testing models. Statistical metrics as mean absolute error (MAE), root square (RMSE) correlation coefficient (R) assess predictive accuracy DT AR demonstrated exceptional accuracy, yielding high coefficients 0.983 0.979 training, 0.873 0.822 testing, respectively. Shapley Additive exPlanation (SHAP) analysis highlighted influential role positively impacting CS, while an increased (w/c) negatively affected CS. showcases efficacy techniques accurately predicting nanoparticle-modified concrete, offering swift cost-effective approach assessing nanomaterial impact reducing reliance time-consuming expensive experiments.
Language: Английский
Citations
28Results in Engineering, Journal Year: 2024, Volume and Issue: 21, P. 101930 - 101930
Published: March 1, 2024
Accurately predicting key features in WWTPs is essential for optimizing plant performance and minimizing operational costs. This study assesses the potential of various machine learning models inflow to anoxic sludge reactors. Firstly, it conducts a comprehensive evaluation diverse models, including k-Nearest Neighbors (kNN), Random Forest (RF), XGBoost, CatBoost, LightGBM, Decision Tree Regression (DTR), flow into Anoxic section under weather conditions (dry, rainy, stormy). Secondly, introduces parsimonious guided by variable importance from XGBoost algorithm. Furthermore, employs SHAP (SHapley Additive exPlanations) elucidate model predictions, providing insights contribution each feature. Data COST Benchmark Simulation Model (BSM1) used verify investigated models' effectiveness. Each dataset consists 14 days influent data at 15-minute intervals, with 80% training. Results show that ensemble methods, particularly CatBoost demonstrate satisfactory predictive results presence increased variability rainy stormy conditions. Notably, achieve average Mean Absolute Percentage Error values 1.33% 1.59%, outperforming other methods.
Language: Английский
Citations
22Results in Engineering, Journal Year: 2024, Volume and Issue: 23, P. 102342 - 102342
Published: June 7, 2024
Precise traffic flow prediction is a central component of advancing intelligent transportation systems, providing essential insights for optimizing management, reducing travel times, and alleviating congestion. This study introduces an efficient deep learning approach that synergistically integrates the benefits wavelet-based denoising Recurrent Neural Networks (RNNs). integrated methodology introduced to effectively capture inherent nonlinearity temporal dependencies in time series data. Specifically, Long Short-Term Memory (LSTM) Gated Unit (GRU) are address challenges associated with accurately forecasting flow. To enhance quality, data preprocessed using exponential smoothing filtering as filters, eliminating outliers. The effectiveness proposed techniques evaluated measurements collected from diverse highway locations across California, including Old Bayshore highway, situated south Interstate 880 (I880), Ashby Ave positioned west 80 (I80) San Francisco Bay Area. results obtained through integrating both architectures, LSTM GRU, within wavelet transform-based filter demonstrate enhancement performance. Symlet Haar wavelets achieved high performance average R2 0.982 0.9811, respectively.
Language: Английский
Citations
21Results in Engineering, Journal Year: 2023, Volume and Issue: 20, P. 101625 - 101625
Published: Nov. 28, 2023
The use of fly ash in cementitious composites has gained popularity. Assessing this property requires expensive and destructive laboratory tests utilizing specialized equipment like the rotating-cutter method. Therefore, there is a need for alternative methods to predict depth wear (DW) such more efficiently cost-effectively. Accordingly, objective research utilize machine learning (ML) approaches, including one individual algorithm (Decision Tree) two ensemble algorithms (AdaBoost Regressor Bagging Regressor) estimate fly-ash-based concrete. A collection 216 experimental records was obtained from existing literature. efficiency models examined with multiple statistical indexes. bagging regressor (BR) model provided superior estimation performance correlation coefficient (R) 0.999 compared AdaBoost (R = 0.965) decision tree 0.962). models, notably BR, accurate predictions an 87.8 % lower mean absolute error (MAE) 85 root square (RMSE) model. In addition, BR exhibited lowest index (ρ) values 0.016 training 0.012 validation. SHapley Additive exPlanation (SHAP) revealed that time testing age are most dominant controlling features significantly contribute wear. conclusion, ML techniques SHAP interpretation DW concrete reduces reliance on lab tests, making durability assessment practical cost-effective.
Language: Английский
Citations
34Applied Energy, Journal Year: 2024, Volume and Issue: 362, P. 123008 - 123008
Published: March 16, 2024
Language: Английский
Citations
12Journal of Environmental Management, Journal Year: 2024, Volume and Issue: 364, P. 121430 - 121430
Published: June 13, 2024
Language: Английский
Citations
8Results in Engineering, Journal Year: 2024, Volume and Issue: 23, P. 102504 - 102504
Published: July 14, 2024
Accurate wind power prediction is critical for efficient grid management and the integration of renewable energy sources into grid. This study presents an effective deep-learning approach that improves short-term forecasting accuracy. The method incorporates a Variational Autoencoder (VAE) with self-attention mechanism applied in both encoder decoder. empowers model to leverage VAE's strengths time-series modeling nonlinear approximation while focusing on most relevant features within data. effectiveness this evaluated through comprehensive comparison eight established deep learning methods, including Recurrent Neural Networks (RNNs), Long Short-Term Memory (LSTM) networks, Bidirectional LSTMs (BiLSTMs), Convolutional (ConvLSTMs), Gated Units (GRUs), Stacked Autoencoders (SAEs), Restricted Boltzmann Machines (RBMs), vanilla VAEs. Real-world data from five turbines France Turkey used evaluation. Five statistical metrics are employed quantitatively assess performance each method. results indicate SA-VAE consistently outperformed other models, achieving highest average R2 value 0.992, demonstrating its superior predictive capability compared existing techniques.
Language: Английский
Citations
8Results in Engineering, Journal Year: 2023, Volume and Issue: 20, P. 101585 - 101585
Published: Nov. 14, 2023
Machine learning is one effective way of increasing the accuracy sediment transport models. captures patterns in sequence both structured and unstructured data uses it for forecasting. In this research, different regression models were train to forecast using 8 years measured collected Sg. Linggui suspended station. Data from scenarios used where each scenario indicates number lags. Seven models, namely, Linear Regression, Regression Trees, Support Vector Machines, Gaussian Process Kernel Approximation, Ensemble Neural Network trained compared. The evaluated Root Mean Square Error (RMSE) Coefficient Determination (R2). best-performing two types chosen they tested test find Relative Percentage (RPE) predicted data. Exponential model performs much better than other terms RMSE R2 values. When exponential all 3 are compared, seems have a better-performing but only by very small margin, after testing data, result shows has less RPE compared Hence, can be deduced that gaussian process overall RSME, R2, RPE.
Language: Английский
Citations
19Results in Engineering, Journal Year: 2024, Volume and Issue: 22, P. 102037 - 102037
Published: March 22, 2024
Desertification detection is a crucial step to improve the management of affected areas and aid in mitigating negative impacts desertification. This study proposes semi-supervised approach that uses Landsat imagery radiometric data detect The involves extracting data, which used as an indicator identify thematic type desertification evolution over time. Four anomaly techniques, including One-Class Support Vector Machine (OCSVM), Isolation Forest, Elliptic Envelope, Local Outlier Factor, are trained on from non-desertified regions abnormal events related These techniques use unlabeled during training only require desertification-free making them practical. was conducted arid region around Biskra, Algeria, well-known area strongly by OCSVM method achieved highest accuracy 95.40% comparison other methods studies. Furthermore, enhance result reliability, Bootstrap technique employed generate 95% confidence intervals for five evaluation metrics.
Language: Английский
Citations
7Arabian Journal for Science and Engineering, Journal Year: 2024, Volume and Issue: 49(10), P. 14351 - 14365
Published: May 3, 2024
Abstract Portland cement concrete (PCC) is the construction material most used worldwide. Hence, its proper characterization fundamental for daily-basis engineering practice. Nonetheless, experimental measurements of PCC’s properties (i.e., Poisson’s Ratio - v -, Elastic Modulus E Compressive Strength -ComS-, and Tensile -TenS-) consume considerable amounts time financial resources. Therefore, development high-precision indirect methods fundamental. Accordingly, this research proposes a computational model based on deep neural networks (DNNs) to simultaneously predict , ComS, TenS. For purpose, Long-Term Pavement Performance database was employed as data source. In regard, mix design parameters PCC are adopted input variables. The performance DNN evaluated with 1:1 lines, goodness-of-fit parameters, Shapley additive explanations assessments, running analysis. results demonstrated that proposed exhibited an exactitude higher than 99.8%, forecasting errors close zero (0). Consequently, machine learning-based designed in investigation helpful tool estimating when laboratory tests not attainable. Thus, main novelty study creating robust determine TenS by solely considering parameters. Likewise, central contribution state-of-the-art achieved present effort public launch developed through open-access GitHub repository, which can be utilized engineers, designers, agencies, other stakeholders.
Language: Английский
Citations
5