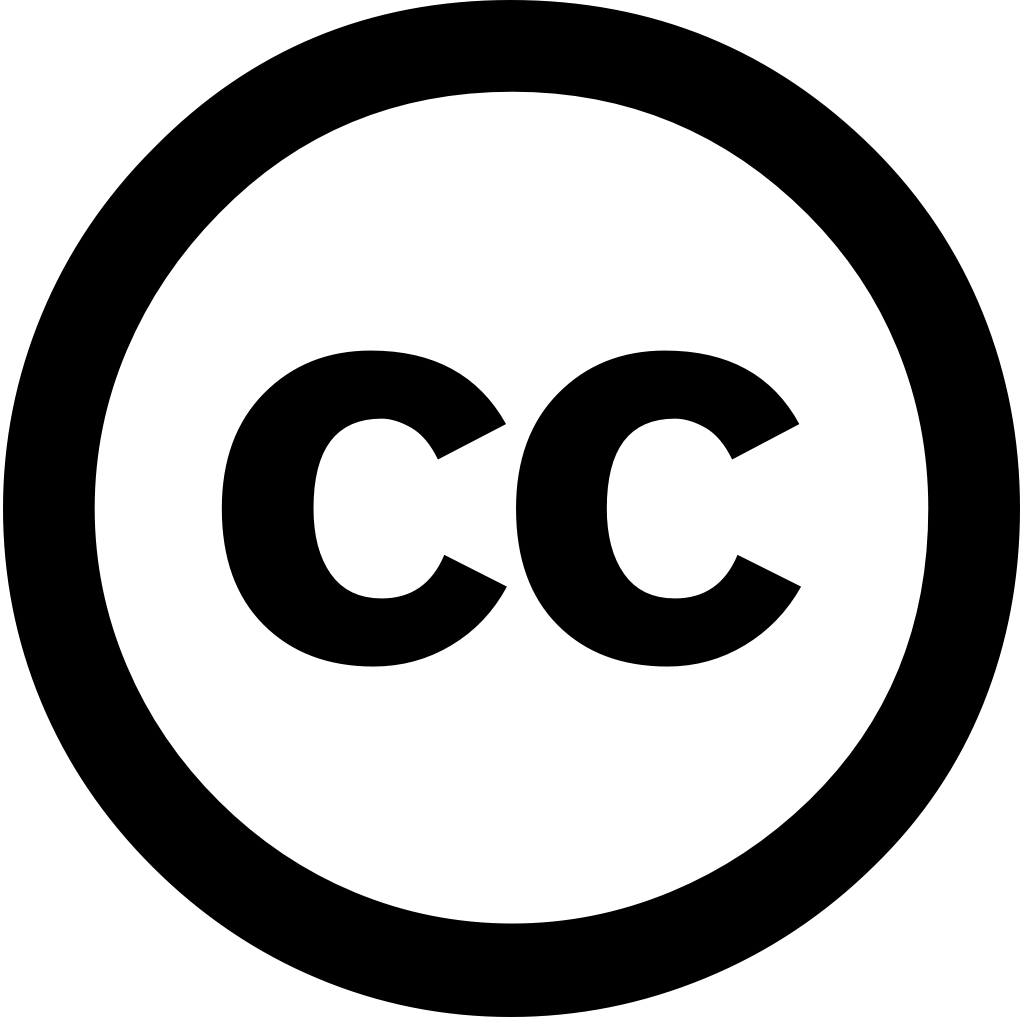
Results in Engineering, Journal Year: 2025, Volume and Issue: unknown, P. 104645 - 104645
Published: March 1, 2025
Language: Английский
Results in Engineering, Journal Year: 2025, Volume and Issue: unknown, P. 104645 - 104645
Published: March 1, 2025
Language: Английский
Turkish Journal of Engineering, Journal Year: 2025, Volume and Issue: 9(3), P. 508 - 518
Published: March 8, 2025
Wind speed prediction plays a crucial role in renewable energy planning and optimization. This study presents comprehensive analysis of wind forecasting using Random Forest (RF) models. The research utilized high-resolution data collected throughout 2023 at the Bowen Abbot facility. Our methodology employed RF with cross-validation techniques to ensure model stability reliability. demonstrated robust performance across multiple evaluation metrics, achieving an average R² score 0.9155 (±0.0035) through 5-fold cross-validation. Error revealed consistent training, testing, validation sets, root mean square errors (RMSE) 0.6624 (±0.0098) m/s. Feature importance that 3-hour rolling was most influential predictor, accounting for 89.84% model's predictive power, followed by 1-hour (2.59%) (2.57%) lagged speeds. hierarchical temporal features suggests recent patterns are accurate predictions. error distribution showed approximately normal distributions slight deviations tails, particularly set (kurtosis: 5.2146). Key findings indicate maintains high accuracy different scales, absolute (MAE) averaging 0.4998 partitions its reliability operational deployment. These results demonstrate potential algorithms applications, providing valuable tool power generation management. study's contribute growing body on machine learning applications energy, offering insights into methodologies systems.
Language: Английский
Citations
0Results in Engineering, Journal Year: 2025, Volume and Issue: unknown, P. 104708 - 104708
Published: March 1, 2025
Language: Английский
Citations
0Processes, Journal Year: 2025, Volume and Issue: 13(4), P. 961 - 961
Published: March 24, 2025
This study develops and evaluates an AI-driven stacked hybrid machine learning model for predicting the total power output of wave energy converters (WECs) across four Australian coastal locations: Adelaide, Perth, Sydney, Tasmania. research enhances prediction accuracy through advanced ensemble techniques while addressing spatial variability in processes. The dataset comprises coordinates readings from 16 fully submerged WECs per location, capturing different regions. Data preprocessing included missing value imputation, duplicate removal, feature transformation via Euclidean distance calculation. Principal component analysis (PCA) was employed to reduce dimensionality preserving critical features influencing generation. To develop accurate model, we a stacking approach using XGBoost, LightGBM, CatBoost as base learners, optimized Optuna hyperparameter tuning with 10-fold cross-validation. A Ridge regression meta-learner combined outputs these models, leveraging their complementary strengths enhance predictive performance. Experimental results demonstrate that consistently outperforms individual enhancing all locations. Sydney exhibited highest (RMSE = 9089.58 W, R2 0.8576), Tasmania posed greatest challenge 45,032.37 0.8378). mitigated overfitting improved generalization by CatBoost. By learning, this provides scalable reliable framework forecasting, facilitating more efficient grid integration resource planning renewable systems.
Language: Английский
Citations
0Results in Engineering, Journal Year: 2025, Volume and Issue: unknown, P. 105151 - 105151
Published: April 1, 2025
Language: Английский
Citations
0Results in Engineering, Journal Year: 2025, Volume and Issue: unknown, P. 104645 - 104645
Published: March 1, 2025
Language: Английский
Citations
0