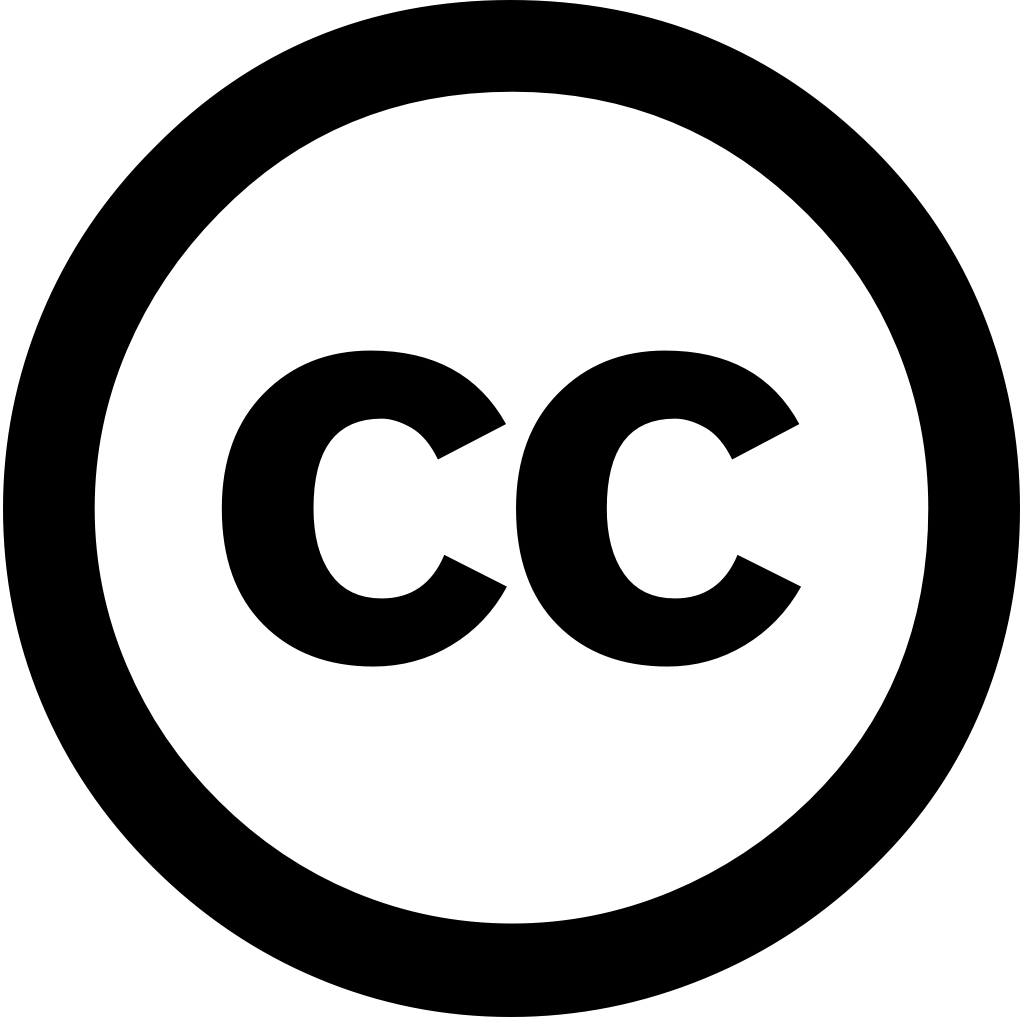
International Journal of Applied Earth Observation and Geoinformation, Journal Year: 2024, Volume and Issue: 134, P. 104145 - 104145
Published: Sept. 12, 2024
Language: Английский
International Journal of Applied Earth Observation and Geoinformation, Journal Year: 2024, Volume and Issue: 134, P. 104145 - 104145
Published: Sept. 12, 2024
Language: Английский
Atmospheric Research, Journal Year: 2024, Volume and Issue: 300, P. 107261 - 107261
Published: Jan. 21, 2024
Language: Английский
Citations
37npj Climate and Atmospheric Science, Journal Year: 2024, Volume and Issue: 7(1)
Published: March 13, 2024
Abstract Surface visibility (SV), a key indicator of atmospheric transparency, is used widely in the fields environmental monitoring, transportation, and aviation. However, sparse distribution limited number SV monitoring sites make it difficult to fulfill urgent need for spatiotemporally seamless fine-scale monitoring. Here, we developed operational real-time retrieval (RT-SVR) framework China that incorporates information from multiple data sources, including Chinese Land Data Assimilation System meteorological data, situ observations, other ancillary data. Seamless hourly with 6.25-km spatial resolution are available real time via RT-SVR model, which was built using two-layer stacked ensemble approach combines machine learning algorithms deep module. Sample-based cross-validation model on approximately 41.3 million pairs revealed strong robustness high accuracy, Pearson correlation coefficient (R) value 0.95 root mean square error (RMSE) 3.17 km. An additional hindcast-validation experiment, performed continuous observations obtained over one year (approximately 20.8 pairs), demonstrated powerful generalization capabilities albeit slight degradation performance ( R = 0.85, RMSE 5.28 km). The update capability enable tracking generation, development, dissipation various low-SV events (e.g., fog, haze, dust storms) China. might also prove useful quantitative aerosol-related parameters PM 2.5 , 10 aerosol optical depth).
Language: Английский
Citations
7Journal of Cleaner Production, Journal Year: 2024, Volume and Issue: 473, P. 143531 - 143531
Published: Aug. 30, 2024
Language: Английский
Citations
4Remote Sensing, Journal Year: 2025, Volume and Issue: 17(9), P. 1632 - 1632
Published: May 4, 2025
Fine particulate matter (PM2.5) has garnered significant scientific and public health concern owing to its capacity for deep penetration into the human respiratory system, presenting risks. Despite implementation of strict environmental policies in China over past decade reduce PM2.5 levels, long-term concerns remain a serious issue. Our study aims provide high-quality, seamless daily dataset covering years 2015 2024. A two-step estimation model is established based on machine learning algorithm spatio-temporal decomposition method. First, we utilize XGBoost (EXtreme Gradient Boosting) address gaps MAIAC (Multi-Angle Implementation Atmospheric Correction) AOD (Aerosol Optical Depth), with R2/RMSE (coefficient determination/Root Mean Square Error) 0.67/0.2678 compared AERONET Robotic Network) AOD. Then, novel approach by integrating EOF (Empirical Orthogonal Function) introduced estimation. The integration allows incorporation entire meteorological field information model, significantly enhancing accuracy: spatial CV (cross-validation)-R2 improved from 0.8340 0.8935, CV-RMSE reduced 13.8177 11.0668. Leveraging newly produced dataset, analyze variations across decomposition, particularly noting that levels eastern anthropogenic intensive regions continuously declined 2020, fluctuated steadily during 2020–2024. This research underscores critical need sustained effective air quality management strategies China.
Language: Английский
Citations
0Remote Sensing of Environment, Journal Year: 2025, Volume and Issue: 326, P. 114795 - 114795
Published: May 7, 2025
Language: Английский
Citations
0The Science of The Total Environment, Journal Year: 2024, Volume and Issue: 926, P. 171910 - 171910
Published: March 24, 2024
Language: Английский
Citations
2IEEE Transactions on Geoscience and Remote Sensing, Journal Year: 2024, Volume and Issue: 62, P. 1 - 15
Published: Jan. 1, 2024
Ambient fine particulate matter (PM 2.5 ) has significant adverse effects on human health, thereby urgent hunger for accurate monitoring of ground-level PM , especially its space distribution. Since satellites can observe the Earth a large spatial scale, remote sensing technology be applied to estimate concentrations at national level. Based it and machine learning (ML) methods, numerous studies mapped high-accuracy, wholesale continuous . However, different models data in these made their results incomparable, more samples were needed provided. Here, large-column long-term sample dataset (ML-CNPM ML-based was constructed with 5,076,608 records 24 features from 2014 2023 China. Multiple approaches used guarantee quantity quality dataset. Due comprehensiveness objectivity, ML-CNPM train validate models, further improving accuracy estimating. Using eight basic also as baseline judging other derivative models. These daily full-coverage most performed well, 10-fold cross-validation RMSE 16.94-11.21μg/m 3 R xmlns:xlink="http://www.w3.org/1999/xlink">2 0.71-0.89, which is consistent previous effectively capture trends period suffered high pollution. Overall, our construct, validate, compare various estimation, helping develop new algorithms higher robustness.
Language: Английский
Citations
2Earth system science data, Journal Year: 2024, Volume and Issue: 16(8), P. 3781 - 3793
Published: Aug. 27, 2024
Abstract. Ground-level PM2.5 data derived from satellites with machine learning are crucial for health and climate assessments. However, uncertainties persist due to the absence of spatially covered observations. To address this, we propose a novel testbed using nontraditional numerical simulations evaluate estimation across entire spatial domain. The emulates general machine-learning approach by training model grids corresponding ground monitoring sites subsequently testing its predictive accuracy other locations. Our enables comprehensive evaluation various methods' performance in estimating domain first time. Unexpected results shown application China, larger absolute biases found densely populated regions abundant observations all benchmark models higher baseline concentration, though relative error (approximately 20 %) is smaller compared that rural areas (over 50 %). imbalance samples, mostly urban high emissions, main reason, leading significant overestimation lack monitors downwind where transported varying vertical profiles. proposed also provides an efficient strategy optimizing structure or samples enhance satellite-retrieval performance. Integration spatiotemporal features, especially conventional neural network (CNN)-based deep-learning approaches like residual (ResNet) model, has successfully mitigated (by 5–30 µg m−3) exposure 3 million people ⋅ area over 9 years (2013–2021) traditional approach. Furthermore, incorporation 600 strategically positioned identified through essential achieving more balanced distribution thereby ensuring precise facilitating assessment associated impacts China. In addition presenting retrieved surface concentrations China 2013 2021, this study dataset physical modeling which can serve data-driven methodologies, such as learning, community (Li et al., 2024a; https://doi.org/10.5281/zenodo.11122294).
Language: Английский
Citations
2ISPRS International Journal of Geo-Information, Journal Year: 2024, Volume and Issue: 13(6), P. 206 - 206
Published: June 17, 2024
Air pollution has become an increasing concern in the Mekong region due to seasonal vegetative burning triggered by related anthropogenic activities and climate change. While assumption of a correlation between agriculture air is common postulation, little evidence exists on association fire incidents concentrations. The current study explores relationship satellite-derived occurrence, land surface characteristics, particulate matter 2.5 (PM2.5) concentrations for five Lower countries, namely Cambodia, Laos, Myanmar, Thailand, Vietnam, effort gain new insights into distributions quality. Publicly available daily active hotspots from VIIRS satellite instrument, annual cover products MODIS satellite, mean monthly ground-level PM2.5 estimates V5.GL.04 database were analyzed two relational assessments; first, distribution counts radiative power (FRP) temporally spatially secondly, correlations counts, cumulative FRP per country type. results suggest statistically significant positive FRP, each country, which differ based cover. strongest incidences was found case Myanmar. For all countries combined, fires detected forests displayed highest with estimates. This demonstrates use product provides important temporal spatial as baseline information prevention mitigation strategies region.
Language: Английский
Citations
1Remote Sensing, Journal Year: 2024, Volume and Issue: 16(18), P. 3363 - 3363
Published: Sept. 10, 2024
By utilizing top-of-atmosphere radiation (TOAR) data from China’s new generation of geostationary satellites (FY-4A and FY-4B) along with interpretable machine learning models, near-surface particulate matter concentrations in China were estimated, achieving hourly temporal resolution, 4 km spatial 100% coverage. First, the cloudless TOAR matched modeled solar products ERA5 dataset to construct estimate a fully covered under assumed clear-sky conditions, which increased coverage 20–30% 100%. Subsequently, this was applied matter. The analysis demonstrated that (R2 = 0.83) performed better than original 0.76). Additionally, using feature importance scores SHAP values, impact meteorological factors air mass trajectories on increase PM10 PM2.5 during dust events investigated. haze indicated main driving changes included pressure, temperature, boundary layer height. concentration obtained exhibit high spatiotemporal resolution. Combined data-driven learning, they can effectively reveal influencing China.
Language: Английский
Citations
1