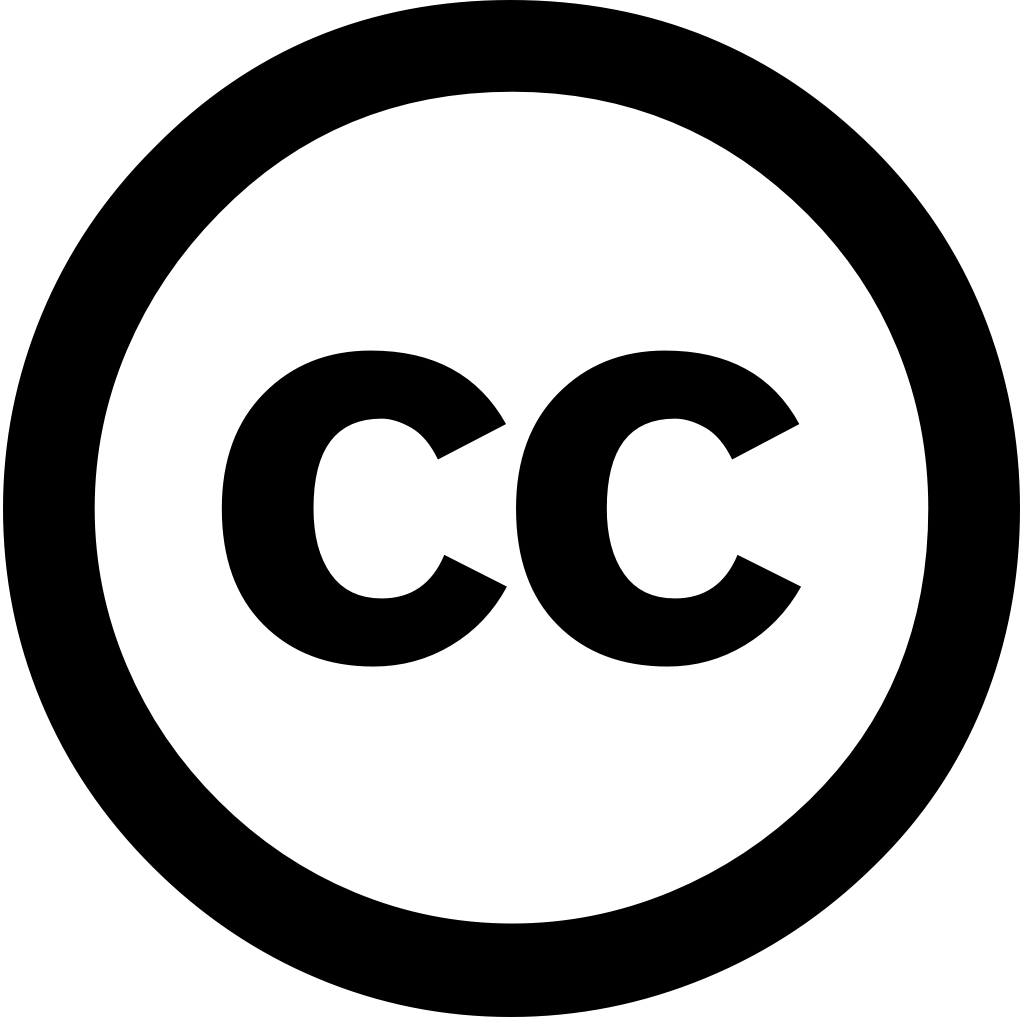
Geoderma, Journal Year: 2024, Volume and Issue: 452, P. 117113 - 117113
Published: Nov. 26, 2024
Language: Английский
Geoderma, Journal Year: 2024, Volume and Issue: 452, P. 117113 - 117113
Published: Nov. 26, 2024
Language: Английский
Published: Jan. 1, 2025
Language: Английский
Citations
0Remote Sensing of Environment, Journal Year: 2025, Volume and Issue: 323, P. 114724 - 114724
Published: March 30, 2025
Language: Английский
Citations
0Remote Sensing of Environment, Journal Year: 2025, Volume and Issue: 323, P. 114736 - 114736
Published: April 5, 2025
Language: Английский
Citations
0Remote Sensing, Journal Year: 2025, Volume and Issue: 17(9), P. 1574 - 1574
Published: April 29, 2025
Accurate and efficient crop maps are essential for decision-makers to improve agricultural monitoring management, thereby ensuring food security. The integration of advanced artificial intelligence (AI) models with hyperspectral remote sensing data, which provide richer spectral information than multispectral imaging, has proven highly effective in the precise discrimination types. This systematic review examines evolution platforms, from Unmanned Aerial Vehicle (UAV)-mounted sensors space-borne satellites (e.g., EnMAP, PRISMA), explores recent scientific advances AI methodologies mapping. A protocol was applied identify 47 studies databases peer-reviewed publications, focusing on sensors, input features, classification architectures. analysis highlights significant contributions Deep Learning (DL) models, particularly Vision Transformers (ViTs) hybrid architectures, improving accuracy. However, also identifies critical gaps, including under-utilization limited multi-sensor need modeling approaches such as Graph Neural Networks (GNNs)-based methods geospatial foundation (GFMs) large-scale type Furthermore, findings highlight importance developing scalable, interpretable, transparent maximize potential imaging (HSI), underrepresented regions Africa, where research remains limited. provides valuable insights guide future researchers adopting HSI reliable mapping, contributing sustainable agriculture global
Language: Английский
Citations
0Remote Sensing, Journal Year: 2025, Volume and Issue: 17(9), P. 1600 - 1600
Published: April 30, 2025
The emergence of new-generation hyperspectral satellites offers more potential for mapping soil properties. This study presents the first assessment EnMAP (Environmental Mapping and Analysis Program) imagery organic matter (SOM) prediction using actual spectral data from 282 samples. Different preprocessing techniques, including Savitzky–Golay (SG) smoothing, second derivative SG, Standard Normal Variate (SNV) transformation, were evaluated in combination with embedded feature selection to identify most relevant wavelengths SOM prediction. Partial Least Squares Regression (PLSR) models developed under different pre-treatment scenarios. best performance was obtained SNV top 30 bands (wavelengths) selected, giving R2 = 0.68, RMSE 0.34%, RPIQ 1.75. successfully identified significant prediction, particularly around 550 nm Vis–NIR region, 1570–1630 nm, 1600 2200 SWIR region. resulting predictions exhibited spatially consistent patterns that corresponded known soil–landscape relationships, highlighting properties despite its limited geographical availability. While these results are promising, this limitations ability PLSR extrapolate beyond sampled areas, suggesting need explore non-linear modeling approaches. Future research should focus on evaluating EnMAP’s advanced machine learning techniques comparing it other available products establish robust protocols satellite-based monitoring.
Language: Английский
Citations
0Geoderma, Journal Year: 2024, Volume and Issue: 452, P. 117113 - 117113
Published: Nov. 26, 2024
Language: Английский
Citations
1