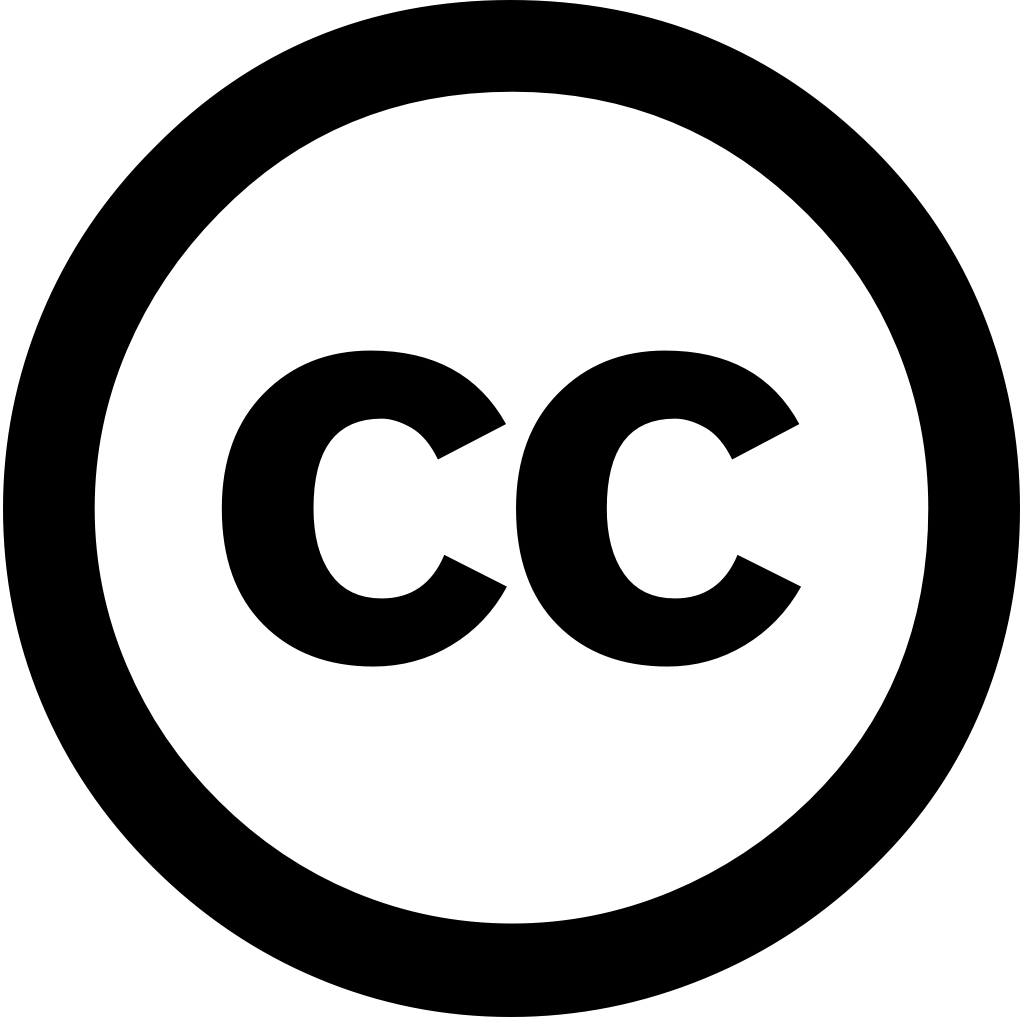
Research Square (Research Square), Journal Year: 2024, Volume and Issue: unknown
Published: Nov. 25, 2024
Language: Английский
Research Square (Research Square), Journal Year: 2024, Volume and Issue: unknown
Published: Nov. 25, 2024
Language: Английский
Plant Phenomics, Journal Year: 2025, Volume and Issue: unknown, P. 100004 - 100004
Published: Feb. 1, 2025
Language: Английский
Citations
1Remote Sensing, Journal Year: 2025, Volume and Issue: 17(8), P. 1409 - 1409
Published: April 16, 2025
Accurate diagnostics of crop yields are essential for climate-resilient agricultural planning; however, conventional datasets often conflate environmental covariates during model training. Here, we present HHHWheatYield1km, a 1 km resolution winter wheat yield dataset China’s Huang-Huai-Hai Plain spanning 2000–2019. By integrating climate-independent multi-source remote sensing metrics with Random Forest model, calibrated against municipal statistical yearbooks, the exhibits strong agreement county-level records (R = 0.90, RMSE 542.47 kg/ha, MRE 9.09%), ensuring independence from climatic influences robust driver analysis. Using Geodetector, reveal pronounced spatial heterogeneity in climate–yield interactions, highlighting distinct regional disparities: precipitation variability exerts strongest constraints on Henan and Anhui, whereas Shandong Jiangsu exhibit weaker dependencies. In Beijing–Tianjin–Hebei, March temperature emerges as critical determinant variability. These findings underscore need tailored adaptation strategies, such enhancing water-use efficiency inland provinces optimizing agronomic practices coastal regions. With its dual ability to resolve pixel-scale dynamics disentangle drivers, HHHWheatYield1km represents resource precision agriculture evidence-based policymaking face changing climate.
Language: Английский
Citations
0ISPRS Journal of Photogrammetry and Remote Sensing, Journal Year: 2025, Volume and Issue: 225, P. 257 - 274
Published: May 5, 2025
Language: Английский
Citations
0Remote Sensing, Journal Year: 2025, Volume and Issue: 17(10), P. 1717 - 1717
Published: May 14, 2025
Crop yield mapping is essential for food security and policy making. Recent machine learning (ML) deep (DL) methods have achieved impressive accuracy in crop estimation. However, these models require numerous training samples that are scarce regions with underdeveloped infrastructure. Furthermore, domain shifts between different spatial prevent DL trained one region from being directly applied to another without adaptation. This effect particularly pronounced significant climate environmental variations such as the U.S. Kenya. To address this issue, we propose using fine-tuning-based transfer learning, which learns general associations predictors response variables data-abundant source then fine-tunes model on data-scarce target domain. We assess model’s performance estimating corn yields Kenya (target domain) (source domain). Feature variables, including time-series vegetation indices (VIs) sequential meteorological both domains, used pre-train fine-tune neural network model. The fine-tuned data 5 years (2019–2023) tested leave-one-year-out cross validation. DNN achieves an overall R2 of 0.632—higher than U.S.-only Kenya-only baselines—but paired significance tests show no aggregate difference, though a statistically gain does occur 2023 under anomalous heat conditions. These results demonstrate fine-tuning can reliably learned representations across continents and, certain climatic scenarios, meaningful improvements.
Language: Английский
Citations
0Research Square (Research Square), Journal Year: 2024, Volume and Issue: unknown
Published: Nov. 25, 2024
Language: Английский
Citations
0