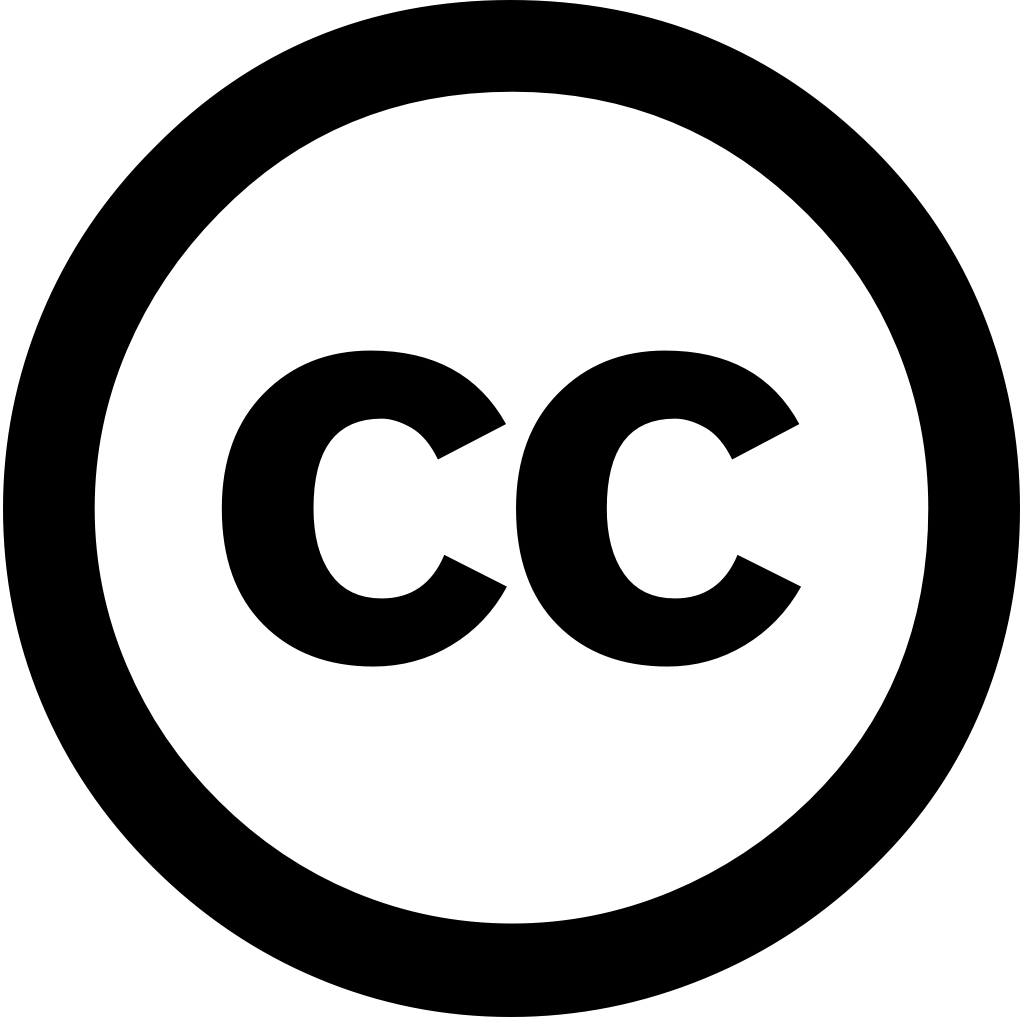
Energy Conversion and Management X, Journal Year: 2024, Volume and Issue: unknown, P. 100843 - 100843
Published: Dec. 1, 2024
Language: Английский
Energy Conversion and Management X, Journal Year: 2024, Volume and Issue: unknown, P. 100843 - 100843
Published: Dec. 1, 2024
Language: Английский
Energy Conversion and Management X, Journal Year: 2025, Volume and Issue: unknown, P. 100907 - 100907
Published: Feb. 1, 2025
Language: Английский
Citations
1Energy, Journal Year: 2024, Volume and Issue: unknown, P. 134285 - 134285
Published: Dec. 1, 2024
Language: Английский
Citations
6International Journal of Thermofluids, Journal Year: 2024, Volume and Issue: unknown, P. 100899 - 100899
Published: Oct. 1, 2024
Language: Английский
Citations
5Fuel, Journal Year: 2024, Volume and Issue: 382, P. 133675 - 133675
Published: Nov. 17, 2024
Language: Английский
Citations
4Desalination, Journal Year: 2025, Volume and Issue: unknown, P. 118595 - 118595
Published: Jan. 1, 2025
Language: Английский
Citations
0Energy Conversion and Management X, Journal Year: 2025, Volume and Issue: unknown, P. 100932 - 100932
Published: Feb. 1, 2025
Language: Английский
Citations
0Thermal Science and Engineering Progress, Journal Year: 2025, Volume and Issue: unknown, P. 103487 - 103487
Published: March 1, 2025
Language: Английский
Citations
0Renewable Energy, Journal Year: 2025, Volume and Issue: unknown, P. 122900 - 122900
Published: March 1, 2025
Language: Английский
Citations
0Atmosphere, Journal Year: 2024, Volume and Issue: 15(11), P. 1344 - 1344
Published: Nov. 9, 2024
In recent years, the application of machine learning methods has become increasingly common in atmospheric science, particularly modeling and predicting processes that impact air quality. This study focuses on hydrogen production from solid oxide electrolytic cells (SOECs), a technology with significant potential for reducing greenhouse gas emissions improving We developed two models using artificial neural networks (ANNs) support vector (SVM) to predict production. The input variables are current, voltage, communication delay time, real-time measured production, while output variable is at next sampling time. Both address critical issue hysteresis. Using 50 h SOEC system data, we evaluated effectiveness ANN SVM methods, incorporating time as an variable. results show model superior terms prediction performance. Specifically, shows strong predictive performance ε = 0.01–0.02 h, RMSE 2.59 × 10−2, MAPE 33.34 10−2%, MAE 1.70 10−2 Nm3/h, R2 99.76 10−2. At 0.03 yields 2.74 34.43 1.73 99.73 model, error values 2.70 44.01 2.24 99.74 they 2.67 43.44 2.11 99.75 With this precision, positive implications pollution control strategies development cleaner energy technologies, contributing overall improvements quality reduction pollutants.
Language: Английский
Citations
2International Journal of Hydrogen Energy, Journal Year: 2024, Volume and Issue: 95, P. 427 - 448
Published: Nov. 20, 2024
Language: Английский
Citations
1