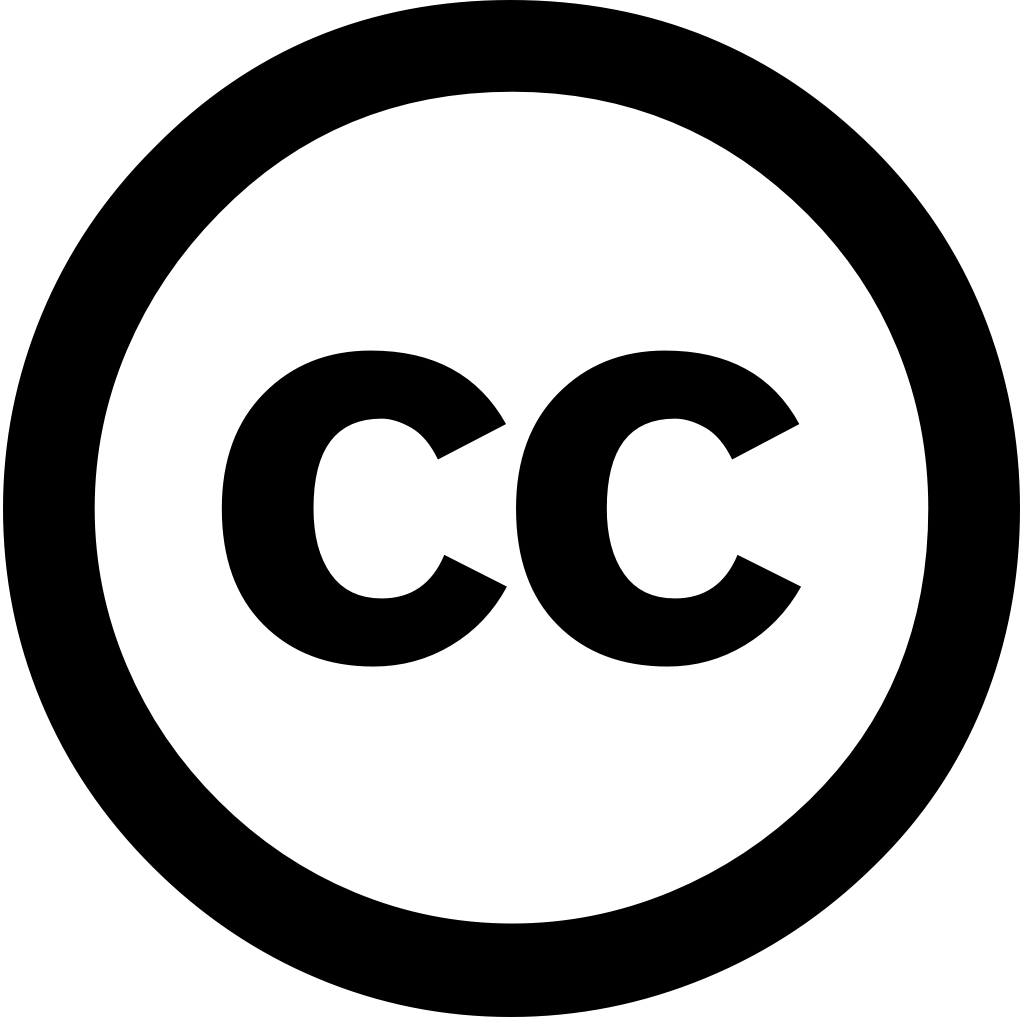
Remote Sensing, Journal Year: 2024, Volume and Issue: 16(24), P. 4730 - 4730
Published: Dec. 18, 2024
Accurate and reliable knowledge about grassland distribution is essential for farmers, stakeholders, government to effectively manage resources from agro-economical ecological perspectives. This study developed a novel pixel-based classification approach using three supervised machine learning (ML) algorithms, which were assessed in the province of Manitoba, Canada. The process involved stages: (1) distinguish between vegetation non-vegetation covers, (2) differentiate non-grassland landscapes, (3) identify specific classes (tame, native, mixed grasses). Initially, this investigated different satellite data, such as Sentinel-1 (S1), Sentinel-2 (S2), Landsat 8 9, individually combined, random forest (RF) method, with best performance at first two steps achieved combination S1 S2. was then utilized conduct support vector (SVM) gradient tree boosting (GTB). In step 3, after filtering out pixels, RF, SVM, GTB classifiers evaluated combined S2 data types. Eighty-nine multitemporal raster-based variables, including spectral bands, SAR backscatters, digital elevation models (DEM), input ML models. RF had highest accuracy 69.96% overall (OA) Kappa value 0.55. After feature selection, variables reduced 61, increasing OA 72.62% 0.58. ranked second, its values improving 67.69% 0.50 72.18% 0.58 selection. impact raster quality on through multisensor image fusion. Grassland Hue, Saturation, Value (HSV) fused images showed higher (59.18%) (0.36) than Brovey Transform (BT) non-fused images. Finally, web map created show results within Soil Landscapes Canada (SLC) polygons, relating soil landscapes providing valuable information decision-makers researchers. Future work may include extending current methodology by considering other influential like meteorological parameters or properties, create comprehensive inventory across whole Prairie ecozone
Language: Английский