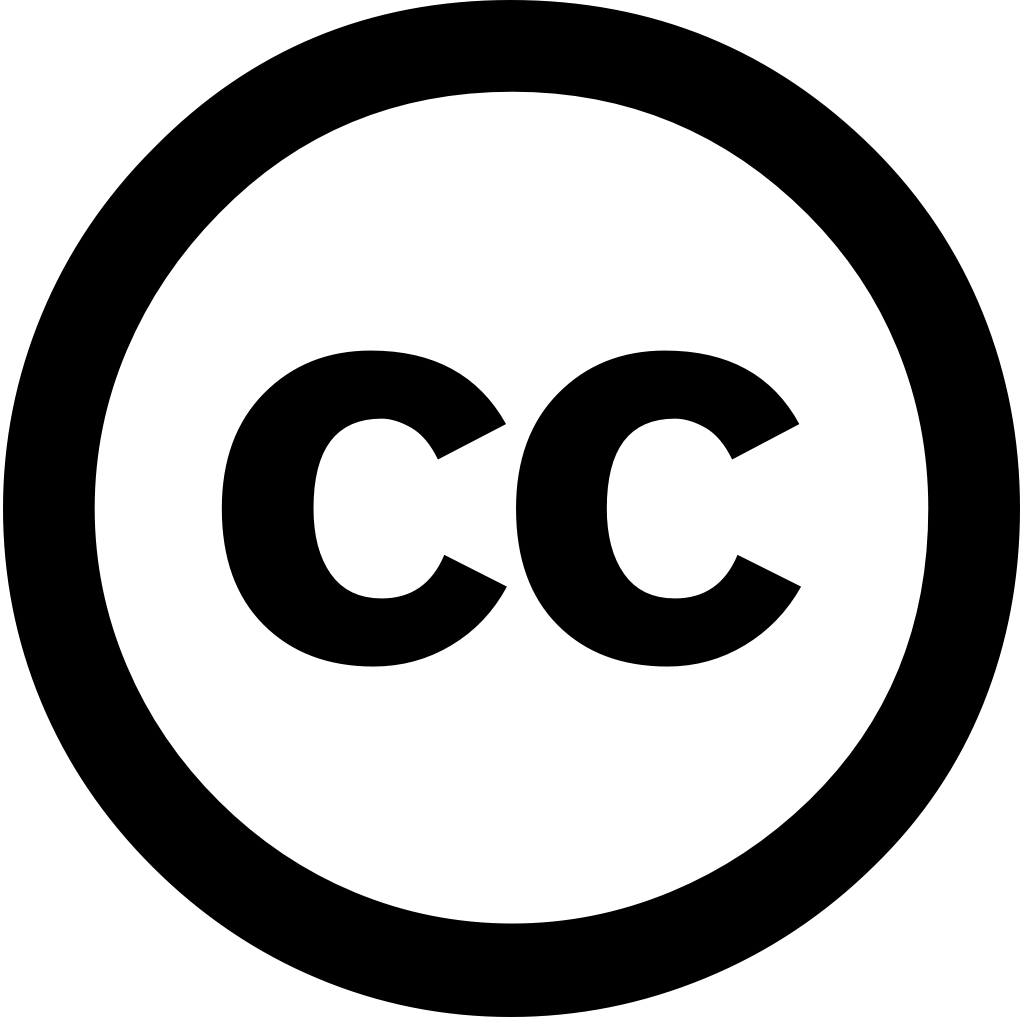
Alexandria Engineering Journal, Journal Year: 2024, Volume and Issue: 111, P. 432 - 445
Published: Oct. 30, 2024
Language: Английский
Alexandria Engineering Journal, Journal Year: 2024, Volume and Issue: 111, P. 432 - 445
Published: Oct. 30, 2024
Language: Английский
Journal of Cleaner Production, Journal Year: 2024, Volume and Issue: 444, P. 141228 - 141228
Published: Feb. 13, 2024
Language: Английский
Citations
13Journal of Hydrologic Engineering, Journal Year: 2024, Volume and Issue: 29(6)
Published: Sept. 14, 2024
Language: Английский
Citations
11Sustainable Energy Technologies and Assessments, Journal Year: 2024, Volume and Issue: 64, P. 103719 - 103719
Published: March 2, 2024
Language: Английский
Citations
6Environmental Research, Journal Year: 2024, Volume and Issue: 246, P. 118533 - 118533
Published: Feb. 26, 2024
Language: Английский
Citations
5Journal of Hydrology, Journal Year: 2024, Volume and Issue: 641, P. 131819 - 131819
Published: Aug. 13, 2024
Language: Английский
Citations
4Journal of Hydrology, Journal Year: 2025, Volume and Issue: unknown, P. 132950 - 132950
Published: Feb. 1, 2025
Language: Английский
Citations
0Hydrology, Journal Year: 2025, Volume and Issue: 12(5), P. 104 - 104
Published: April 26, 2025
Accurate and prompt flood forecasting is essential for effective decision making in control to help minimize or prevent damage. We propose a new custom deep learning model, IF-CNN-GRU, multi-step-ahead that incorporates the index (IF) improve prediction accuracy. The model integrates convolutional neural networks (CNNs) gated recurrent (GRUs) analyze spatiotemporal characteristics of hydrological data, while using recursive network adjusts unit output at each moment based on index. IF-CNN-GRU was applied forecast floods with lead time 1–5 d Baihe station middle reaches Han River, China, accompanied by an in-depth investigation uncertainty. results showed incorporating IF improved precision up 20%. analysis uncertainty revealed contributions modeling factors, such as datasets, structures, their interactions, varied across periods. interaction factors contributed 17–36% uncertainty, contribution datasets increased period (32–53%) structure decreased (32–28%). experiment also demonstrated data samples play critical role improving accuracy, offering actionable insights reduce predictive providing scientific basis early warning systems water resource management.
Language: Английский
Citations
0The Science of The Total Environment, Journal Year: 2023, Volume and Issue: 907, P. 167767 - 167767
Published: Oct. 11, 2023
Language: Английский
Citations
8Expert Systems with Applications, Journal Year: 2024, Volume and Issue: 263, P. 125744 - 125744
Published: Nov. 8, 2024
Language: Английский
Citations
2Electronics, Journal Year: 2024, Volume and Issue: 13(14), P. 2681 - 2681
Published: July 9, 2024
Due to the frequent occurrence of extreme weather in recent years, accurate runoff prediction is crucial for rational planning and management water resources. Addressing high uncertainty multiple influencing factors prediction, this paper proposes a method driven by multi-source data. Based on multivariate observed data runoff, level, temperature, precipitation, Time2Vec-TCN-Transformer model proposed research compared with LSTM, TCN, TCN-Transformer models. The results show that outperforms other models metrics including MAE, RRMSE, MAPE, NSE, demonstrating higher accuracy reliability. By effectively combining Time2Vec, Transformer, improves MAPE forecasting 1–4 days future approximately 7% traditional LSTM 4% standalone TCN model, while maintaining NSE consistently between 0.9 1. This can better capture periodicity, long-term scale information, relationships among variables data, providing reliable predictive support flood resources management.
Language: Английский
Citations
1