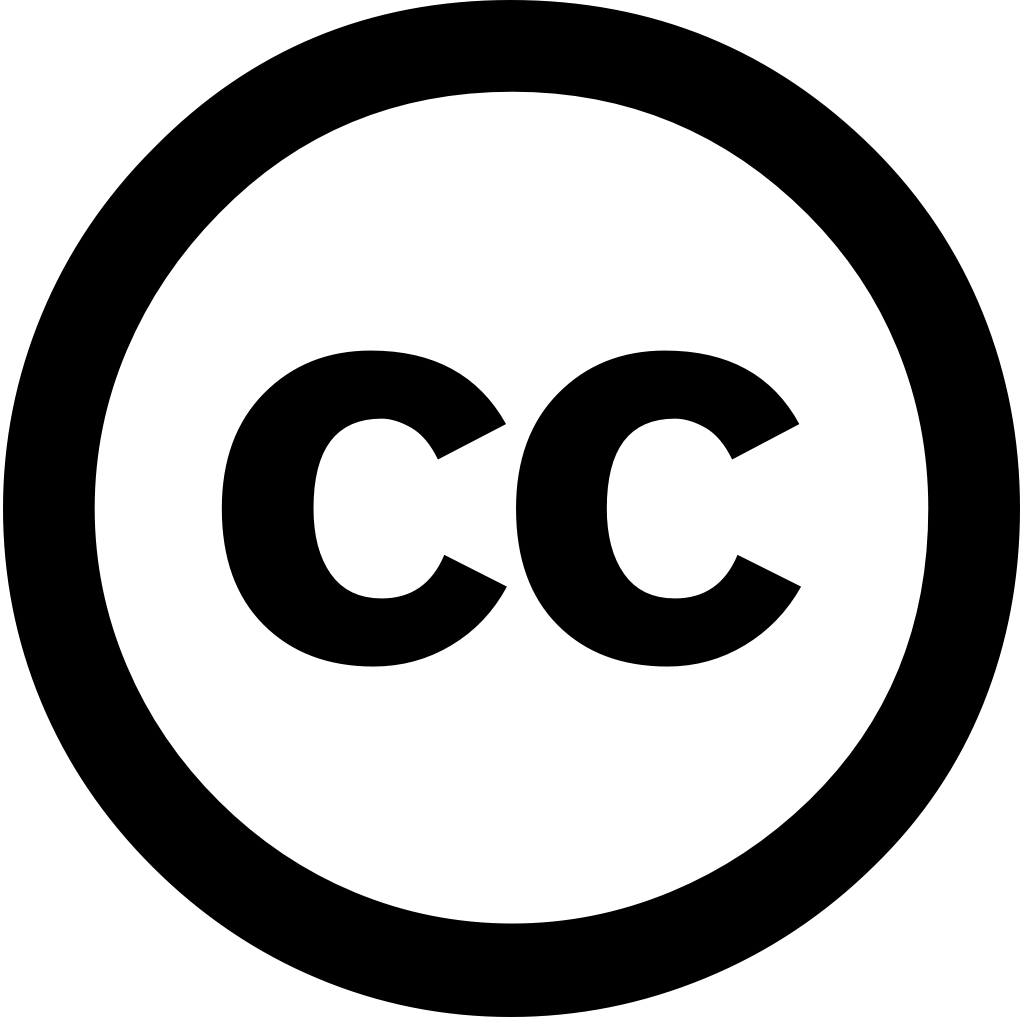
Energy Nexus, Journal Year: 2024, Volume and Issue: 16, P. 100325 - 100325
Published: Sept. 13, 2024
Language: Английский
Energy Nexus, Journal Year: 2024, Volume and Issue: 16, P. 100325 - 100325
Published: Sept. 13, 2024
Language: Английский
Engineering Applications of Computational Fluid Mechanics, Journal Year: 2025, Volume and Issue: 19(1)
Published: Jan. 17, 2025
Language: Английский
Citations
1Geoscience Frontiers, Journal Year: 2024, Volume and Issue: 15(6), P. 101916 - 101916
Published: Aug. 23, 2024
Language: Английский
Citations
8The Science of The Total Environment, Journal Year: 2024, Volume and Issue: 946, P. 174357 - 174357
Published: June 28, 2024
Language: Английский
Citations
6Journal of Hydrology Regional Studies, Journal Year: 2024, Volume and Issue: 54, P. 101858 - 101858
Published: June 13, 2024
Language: Английский
Citations
4Journal of Hydrology Regional Studies, Journal Year: 2024, Volume and Issue: 56, P. 101985 - 101985
Published: Oct. 2, 2024
Language: Английский
Citations
4Journal of Hydrology Regional Studies, Journal Year: 2025, Volume and Issue: 59, P. 102339 - 102339
Published: March 26, 2025
Language: Английский
Citations
0Journal of Hydrology, Journal Year: 2025, Volume and Issue: unknown, P. 133292 - 133292
Published: April 1, 2025
Language: Английский
Citations
0Heliyon, Journal Year: 2024, Volume and Issue: 10(16), P. e35987 - e35987
Published: Aug. 1, 2024
Rivers worldwide are warming due to the impact of climate change and human interventions. This study investigated river heatwaves in Vistula River Basin, one largest systems Europe using long-term observed daily water temperatures from past 30 years (1991-2020). The results showed that increased frequency intensity Basin. total number clear increasing trend with an average rate 1.400 times/decade, duration at 14.506 days/decade, cumulative 53.169 °C/decade. Mann-Kendall (MK) test was also employed, showing statistically significant trends number, duration, for all rivers, including main watercourse its tributaries, few exceptions. Air temperature is major controller each hydrological station, increase air temperatures, will intensity. Another impacting factor flow, tend decrease suggested mitigation measures shall be taken reduce effect on systems.
Language: Английский
Citations
3Frontiers in Environmental Science, Journal Year: 2024, Volume and Issue: 12
Published: July 9, 2024
Assessing water quality becomes imperative to facilitate informed decision-making concerning the availability and accessibility of resources in Korattur Lake, Chennai, India, which has been adversely affected by human actions. Although numerous state-of-the-art studies have made significant advancements classification, conventional methods for training machine learning model parameters still require substantial material resources. Hence, this study employs stochastic gradient descent (SGD), adaptive boosting (AdaBoosting), Perceptron, artificial neural network algorithms classify categories as these well-established methods, combined with Bayesian optimization hyperparameter tuning, provide a robust framework demonstrate performance enhancements classification. The input features from 2010 2019 comprise such pH, phosphate, total dissolved solids (TDS), turbidity, nitrate, iron, chlorides, sodium, chemical oxygen demand (COD). is employed dynamically tune hyperparameters different select optimal best performance. Comparing algorithms, AdaBoosting exhibits highest level indicated its superior accuracy (100%), precision recall F1 score (100%). top four important factors classification are COD (0.684), phosphate (0.119), iron (0.112), TDS (0.084). Additionally, variations or changes levels likely coincide similar levels.
Language: Английский
Citations
1Journal of Hydraulic Research, Journal Year: 2024, Volume and Issue: 62(4), P. 370 - 382
Published: July 3, 2024
Deep learning (DL) is a powerful tool that has proven highly effective in many applications, but creating new deep models becoming increasingly challenging. However, some fields, such as fluid dynamics, theoretical can help design DL models. Based on the existing air2water (A2W) model, this paper proposes hybrid neural network DL-A2W, which combines long short-term memory (LSTM) with A2W model to predict lake water temperature. The DL-A2W was established using datasets of UK Centre for Ecology & Hydrology, and performance evaluated through three experiments. Compared other models, lowest mean absolute error (MAE), percent (MAPE), root squared (RMSE), highest Nash-Sutcliffe efficiency coefficient (NSC) at any given prediction step. values MAE, MAPE, RMSE NSC test set were 0.223–0.388, 1.946–3.296%, 0.375–0.647 0.985–0.995, respectively. results show good generalization ability portability, accurately perform multi-step ahead
Language: Английский
Citations
1