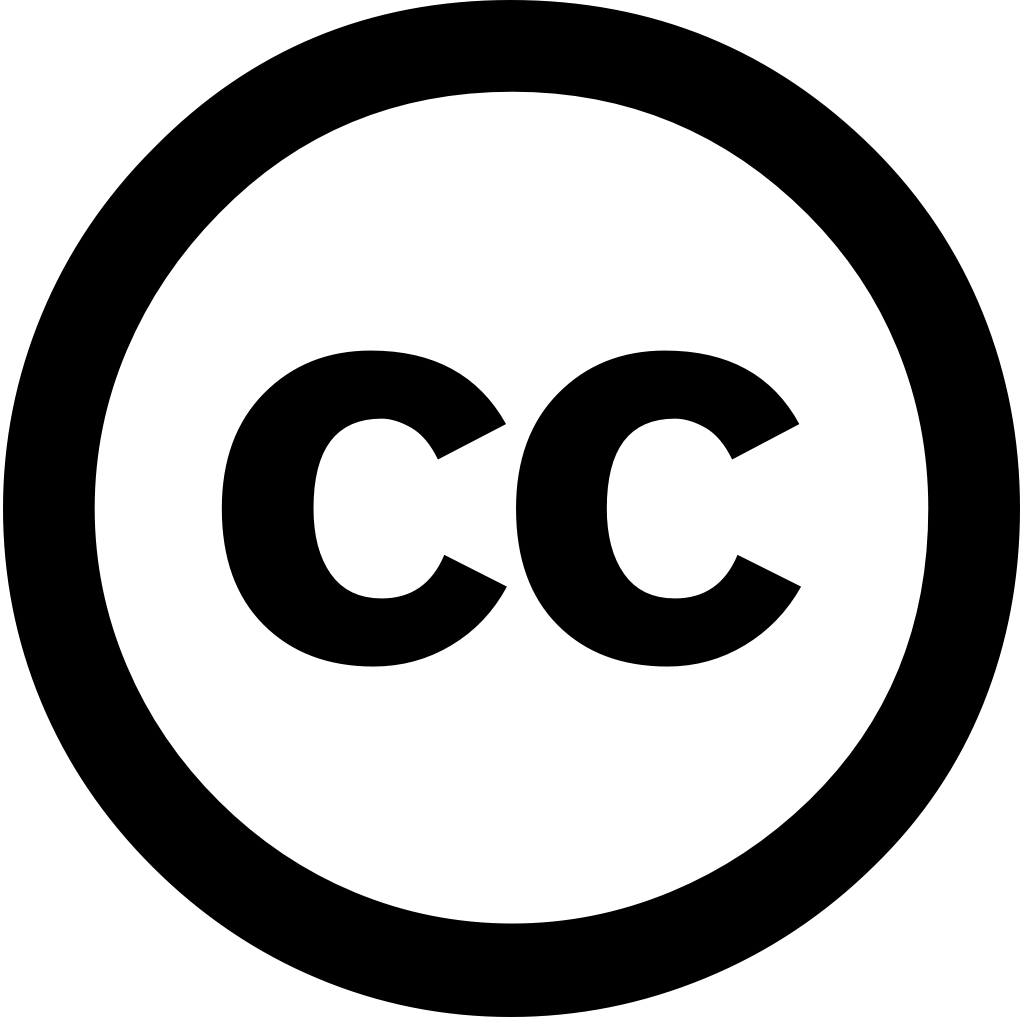
Scientific Reports, Journal Year: 2024, Volume and Issue: 14(1)
Published: Dec. 28, 2024
Language: Английский
Scientific Reports, Journal Year: 2024, Volume and Issue: 14(1)
Published: Dec. 28, 2024
Language: Английский
International Journal of Intelligent Computing and Cybernetics, Journal Year: 2024, Volume and Issue: 18(1), P. 133 - 152
Published: Nov. 13, 2024
Purpose Vision transformers (ViT) detectors excel in processing natural images. However, when remote sensing images (RSIs), ViT methods generally exhibit inferior accuracy compared to approaches based on convolutional neural networks (CNNs). Recently, researchers have proposed various structural optimization strategies enhance the performance of detectors, but progress has been insignificant. We contend that frequent scarcity RSI samples is primary cause this problem, and model modifications alone cannot solve it. Design/methodology/approach To address this, we introduce a faster RCNN-based approach, termed QAGA-Net, which significantly enhances recognition. Initially, propose novel quantitative augmentation learning (QAL) strategy sparse data distribution RSIs. This integrated as QAL module, plug-and-play component active exclusively during model’s training phase. Subsequently, enhanced feature pyramid network (FPN) by introducing two efficient modules: global attention (GA) module long-range dependencies multi-scale information fusion, an pooling (EP) optimize capability understand both high low frequency information. Importantly, QAGA-Net compact size achieves balance between computational efficiency accuracy. Findings verified using different models detector’s backbone. Extensive experiments NWPU-10 DIOR20 datasets demonstrate superior 23 other or CNN literature. Specifically, shows increase mAP 2.1% 2.6% challenging dataset top-ranked respectively. Originality/value paper highlights impact detection performance. fundamentally data-driven approach: module. Additionally, introduced modules FPN. More importantly, our potential collaborate with method does not require any
Language: Английский
Citations
8Forests, Journal Year: 2024, Volume and Issue: 15(7), P. 1244 - 1244
Published: July 17, 2024
A lightweight forest fire detection model based on YOLOv8 is proposed in this paper response to the problems existing traditional sensors for detection. The performance of easily constrained by hardware computing power, and their adaptability different environments needs improvement. To balance accuracy speed detection, GhostNetV2 network adopted replace backbone feature extraction YOLOv8. Ghost module utilized convolution operations, conducting independently dimensional channels, significantly reducing complexity while maintaining excellent performance. Additionally, an improved CPDCA channel priority attention mechanism proposed, which extracts spatial features through dilated convolution, thereby computational overhead enabling focus more targets, achieving accurate In problem small targets Inner IoU loss function introduced. By adjusting size auxiliary bounding boxes, effectively enhances convergence effect target further missed detections, improving overall accuracy. Experimental results indicate that, compared with methods, algorithm improves average precision FPS a smaller size. Through experimental analysis, YOLOv3-tiny, increased 5.9% frame rate reached 285.3 when was only 4.9 M; Shufflenet, 2.9%, inference tripled. addresses false positives, such as cloud reflective light, enhancing detections.
Language: Английский
Citations
5Fire, Journal Year: 2025, Volume and Issue: 8(1), P. 23 - 23
Published: Jan. 10, 2025
Outdoor fire detection faces significant challenges due to complex and variable environmental conditions. Fiber Optic Distributed Temperature Sensing (FO-DTS), recognized for its high sensitivity broad monitoring range, provides advantages in detecting outdoor fires. However, prediction models trained laboratory settings often yield false missed alarms when deployed settings, interferences. To address this issue, study developed a fixed-power source simulation device establish reliable small-scale experimental platform incorporating various influences generating anomalous temperature data. We employed deep learning autoencoders (AEs) integrate spatiotemporal data, aiming minimize the impact of conditions on performance. This research focused analyzing how changes rapid fluctuations affected capabilities, evaluating metrics such as accuracy delay. Results showed that, compared AE VAE handling spatial or temporal CNN-AE demonstrated superior anomaly performance strong robustness applied Furthermore, findings emphasize that factors extreme temperatures can affect outcomes, increasing likelihood alarms. underscores potential utilizing FO-DTS data with scenarios suggestions mitigating interference practical applications.
Language: Английский
Citations
0Applied Sciences, Journal Year: 2025, Volume and Issue: 15(7), P. 3699 - 3699
Published: March 27, 2025
As global climate change escalates, wildfires have emerged as a critical form of natural disaster, presenting substantial risks to ecosystems, public safety, and economic development. While satellite remote sensing has been extensively utilized for wildfire monitoring, current methodologies face limitations in addressing complex backgrounds environmental variations. These techniques usually depend on set thresholds or the extraction local features, which can lead incorrect positives overlooked detections. Consequently, existing methods inadequately capture comprehensive characteristics fire points. To mitigate these challenges, this study proposes deep-learning-based point detection method that integrates Swin Transformer BiLSTM multi-dimensional features associated with This research represents inaugural application context detection, leveraging its self-attention mechanism discern dependencies information within environments. By amalgamating at various levels, proposed significantly improves accuracy robustness detection. Experimental findings demonstrate surpasses traditional models such DenseNet, SimpleCNN, Multi-Layer Perceptron (MLP) across multiple performance metrics, including accuracy, recall, F1 score.
Language: Английский
Citations
0Fire, Journal Year: 2025, Volume and Issue: 8(4), P. 142 - 142
Published: April 2, 2025
The increasing frequency and severity of agricultural fires pose significant threats to food security, economic stability, environmental sustainability. Traditional fire-detection methods, relying on satellite imagery ground-based sensors, often suffer from delayed response times high false-positive rates, limiting their effectiveness in mitigating fire-related damages. In this study, we propose an advanced deep learning-based framework that integrates the Single-Shot MultiBox Detector (SSD) with computationally efficient MobileNetV2 architecture. This integration enhances real-time fire- smoke-detection capabilities while maintaining a lightweight deployable model suitable for Unmanned Aerial Vehicle (UAV)-based monitoring. proposed was trained evaluated custom dataset comprising diverse fire scenarios, including various conditions intensities. Comprehensive experiments comparative analyses against state-of-the-art object-detection models, such as You Only Look Once (YOLO), Faster Region-based Convolutional Neural Network (Faster R-CNN), SSD-based variants, demonstrated superior performance our model. results indicate approach achieves mean Average Precision (mAP) 97.7%, significantly surpassing conventional models detection speed 45 frames per second (fps) requiring only 5.0 GFLOPs computational power. These characteristics make it particularly deployment edge-computing environments, UAVs remote monitoring systems.
Language: Английский
Citations
0Forests, Journal Year: 2025, Volume and Issue: 16(4), P. 704 - 704
Published: April 19, 2025
In recent years, the increasingly significant impacts of climate change and human activities on environment have led to more frequent occurrences extreme events such as forest fires. The recurrent wildfires pose severe threats ecological environments life safety. Consequently, fire prediction has become a current research hotspot, where accurate forecasting technologies are crucial for reducing economic losses, improving management efficiency, ensuring personnel safety property security. To enhance comprehensive understanding wildfire research, this paper systematically reviews studies since 2015, focusing two key aspects: datasets with related tools algorithms. We categorized literature into three categories: statistical analysis physical models, traditional machine learning methods, deep approaches. Additionally, review summarizes data types open-source used in selected literature. further outlines challenges future directions, including exploring risk multimodal learning, investigating self-supervised model interpretability developing explainable integrating physics-informed models constructing digital twin technology real-time simulation scenario analysis. This study aims provide valuable support natural resource enhanced environmental protection through application remote sensing artificial intelligence
Language: Английский
Citations
0International Journal of Applied Earth Observation and Geoinformation, Journal Year: 2025, Volume and Issue: 137, P. 104416 - 104416
Published: Feb. 15, 2025
Language: Английский
Citations
0Technology Knowledge and Learning, Journal Year: 2025, Volume and Issue: unknown
Published: April 2, 2025
Language: Английский
Citations
0Scientific Reports, Journal Year: 2025, Volume and Issue: 15(1)
Published: May 10, 2025
Language: Английский
Citations
0Forests, Journal Year: 2024, Volume and Issue: 15(9), P. 1672 - 1672
Published: Sept. 23, 2024
Forest fires are a major natural calamity that inflict substantial harm on forest resources and the socio-economic landscape. The eastern region of China is particularly susceptible to frequent fires, characterized by high population density vibrant economic activities. Precise forecasting in this area essential for devising effective prevention strategies. This research utilizes blend kernel analysis, autocorrelation standard deviation ellipse method, augmented geographic information systems (GISs) deep-learning techniques, develop an accurate prediction system forest-fire occurrences. model incorporates data meteorological conditions, topography, vegetation, infrastructure, socio-cultural factors produce monthly forecasts assessments. approach enables identification spatial patterns temporal trends fire occurrences, enhancing both precision breadth predictions. results show global local analyses reveal high-incidence areas mainly concentrated Guangdong, Fujian, Zhejiang provinces, with cities like Jiangmen exhibiting distinct concentration characteristics varied distribution Kernel analysis further pinpoints high-density zones primarily Meizhou, Qingyuan, Guangdong Province, Dongfang City Hainan Province. Standard centroid shift indicate significant northward fire-occurrence over past 20 years, expanding range, decreasing flattening, relatively stable direction. performs effectively validation set, achieving accuracy 80.6%, F1 score 81.6%, AUC 88.2%, demonstrating its practical applicability. Moreover, zoning reveals spring winter Zhejiang, Hainan, while autumn shows widespread medium-incidence areas, summer presents lower occurrences most regions. These findings illustrate influence seasonal climate variations highlight necessity enhanced monitoring measures tailored different seasons.
Language: Английский
Citations
1