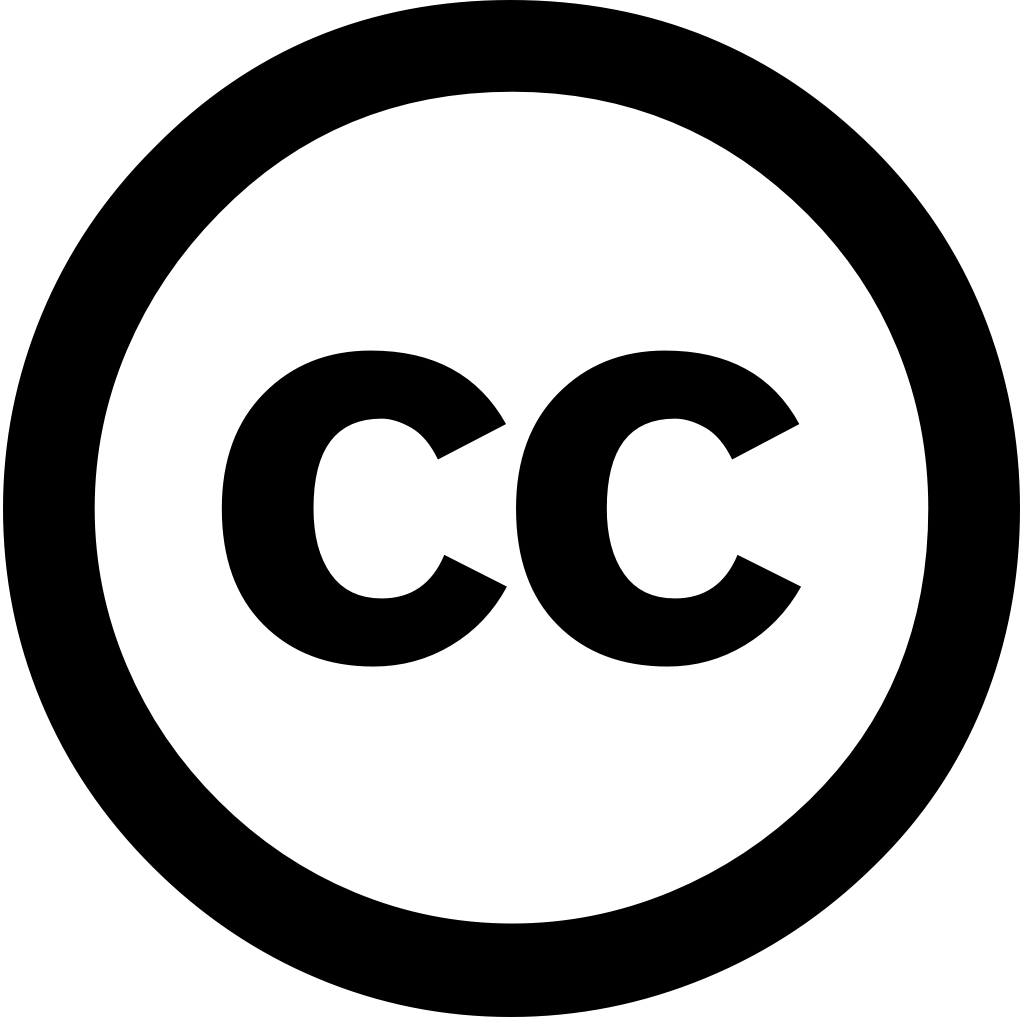
Scientific Reports, Journal Year: 2024, Volume and Issue: 14(1)
Published: Dec. 28, 2024
Language: Английский
Scientific Reports, Journal Year: 2024, Volume and Issue: 14(1)
Published: Dec. 28, 2024
Language: Английский
Engineering Applications of Artificial Intelligence, Journal Year: 2024, Volume and Issue: 142, P. 109902 - 109902
Published: Dec. 22, 2024
Language: Английский
Citations
1Fire, Journal Year: 2024, Volume and Issue: 7(12), P. 451 - 451
Published: Nov. 30, 2024
Fires constitute a significant risk to public safety and property, making early accurate detection essential for an effective response damage mitigation. Traditional fire methods have limitations in terms of accuracy adaptability, particularly complex environments which various stages (such as smoke active flames) need be distinguished. This study addresses the critical comprehensive system capable multistage classification, differentiating between non-fire, smoke, apartment fires, forest fires. We propose deep learning-based model using customized DenseNet201 architecture that integrates preprocessing steps explainable AI techniques, such Grad-CAM++ SmoothGrad, enhance transparency interpretability. Our was trained tested on diverse, multisource dataset, achieving 97%, along with high precision recall. The comparative results demonstrate superiority proposed over other baseline models handling detection. research provides advancement toward more reliable, interpretable, systems adapting different types, opening new possibilities environmentally friendly type detection, ultimately enhancing enabling faster, targeted emergency responses.
Language: Английский
Citations
0Forests, Journal Year: 2024, Volume and Issue: 15(12), P. 2146 - 2146
Published: Dec. 5, 2024
Forest fires represent a paramount natural disaster of global concern. Zhejiang Province has the highest forest coverage rate in China, and are one main disasters impacting management region. In this study, we comprehensively analyzed spatiotemporal distribution based on MODIS data from 2013 to 2023. The results showed that annual incidence shown an overall downward trend 2023, with occurring more frequently winter spring. By utilizing eight contributing factors fire occurrence as variables, three models were constructed: Logistic Regression (LR), Random (RF), eXtreme Gradient Boosting (XGBoost). RF XGBoost demonstrated high predictive ability, achieving accuracy rates 0.85 0.92, f1-score 0.84 AUC values 0.892 0.919, respectively. Further analysis using revealed elevation precipitation had most significant effects fires. Additionally, predictions risk generated by indicated is southern part Province, particularly Wenzhou Lishui areas, well southwest Hangzhou area north Quzhou area. future, can be predicted site models, providing scientific reference for aiding prevention mitigation impacts
Language: Английский
Citations
0Published: Nov. 23, 2024
Language: Английский
Citations
0Scientific Reports, Journal Year: 2024, Volume and Issue: 14(1)
Published: Dec. 28, 2024
Language: Английский
Citations
0