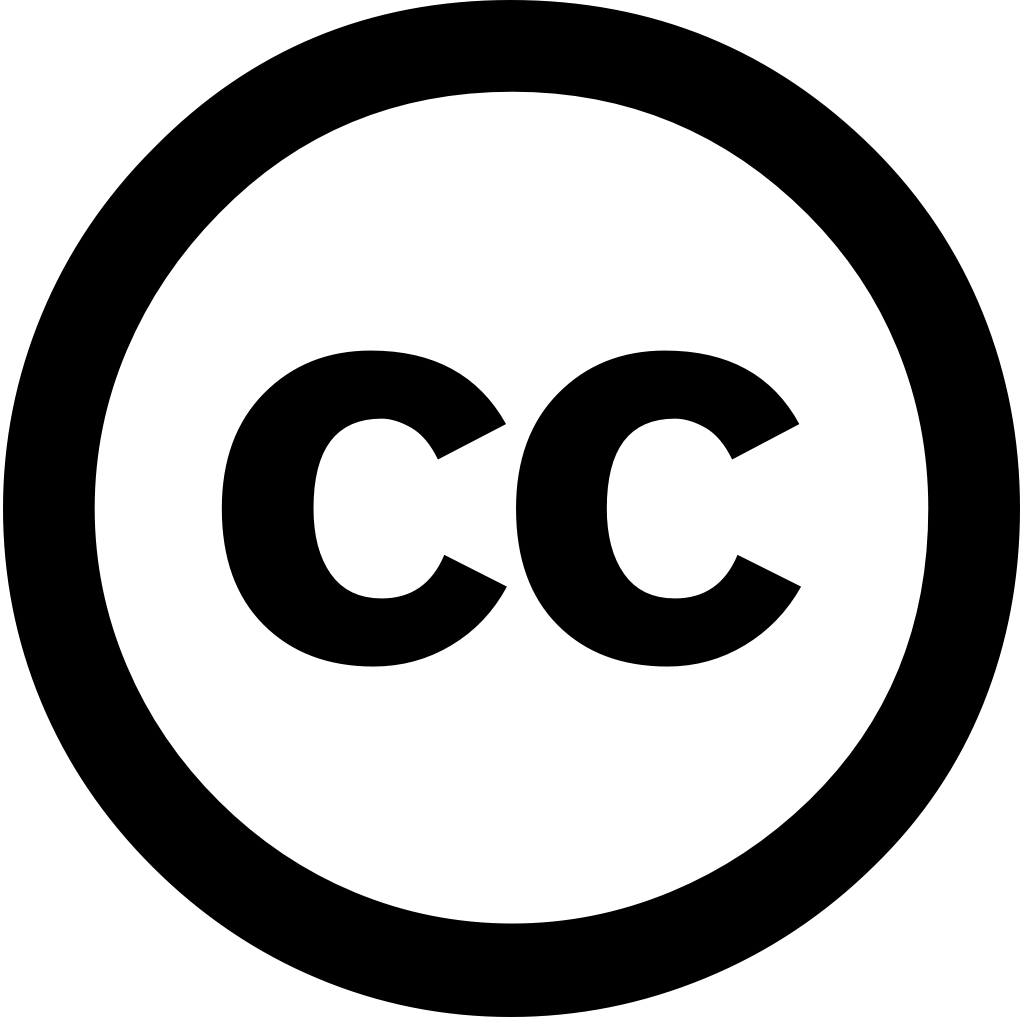
Agronomy, Journal Year: 2024, Volume and Issue: 14(12), P. 2986 - 2986
Published: Dec. 15, 2024
Accurate mapping of tea plantations is crucial for agricultural management and economic planning, yet it poses a significant challenge due to the complex variable nature cultivation landscapes. This study presents high-precision approach in Anji County, Zhejiang Province, China, utilizing multi-source remote sensing data advanced deep learning models. We employed combination Sentinel-2 optical imagery, Sentinel-1 synthetic aperture radar digital elevation models capture rich spatial, spectral, temporal characteristics plantations. Three models, namely U-Net, SE-UNet, Swin-UNet, were constructed trained semantic segmentation Cross-validation point-based accuracy assessment methods used evaluate performance The results demonstrated that Swin-UNet model, transformer-based capturing long-range dependencies global context superior feature extraction, outperformed others, achieving an overall 0.993 F1-score 0.977 when using multi-temporal data. integration with slightly improved classification accuracy, particularly areas affected by cloud cover, highlighting complementary imagery all-weather monitoring. also analyzed influence terrain factors, such as elevation, slope, aspect, on plantation mapping. It was found at higher altitudes or north-facing slopes exhibited improves increasing likely simpler land cover types tea’s preference shade. findings this research not only provide valuable insights into precision but contribute broader application
Language: Английский